diff --git a/materials/sections/adc-intro-to-policies.Rmd b/materials/sections/adc-intro-to-policies.Rmd
deleted file mode 100644
index c98e84e3..00000000
--- a/materials/sections/adc-intro-to-policies.Rmd
+++ /dev/null
@@ -1,126 +0,0 @@
-## Introduction to the Arctic Data Center and NSF Standards and Policies
-
-### Learning Objectives
-
-In this lesson, we will discuss:
-
-- The mission and structure of the Arctic Data Center
-- How the Arctic Data Center supports the research community
-- About data policies from the NSF Arctic program
-
-### Arctic Data Center - History and Introduction
-
-The Arctic Data Center is the primary data and software repository for the Arctic section of National Science Foundation’s Office of Polar Programs (NSF OPP).
-
-We’re best known in the research community as a data archive – researchers upload their data to preserve it for the future and make it available for re-use. This isn’t the end of that data’s life, though. These data can then be downloaded for different analyses or synthesis projects. In addition to being a data discovery portal, we also offer top-notch tools, support services, and training opportunities. We also provide data rescue services.
-
-
-
-NSF has long had a commitment to data reuse and sharing. Since our start in 2016, we’ve grown substantially – from that original 4 TB of data from ACADIS to now over 76 TB at the start of 2023. In 2021 alone, we saw 16% growth in dataset count, and about 30% growth in data volume. This increase has come from advances in tools – both ours and of the scientific community, plus active community outreach and a strong culture of data preservation from NSF and from researchers. We plan to add more storage capacity in the coming months, as researchers are coming to us with datasets in the terabytes, and we’re excited to preserve these research products in our archive. We’re projecting our growth to be around several hundred TB this year, which has a big impact on processing time. Give us a heads up if you’re planning on having larger submissions so that we can work with you and be prepared for a large influx of data.
-
-
-
-The data that we have in the Arctic Data Center comes from a wide variety of disciplines. These different programs within NSF all have different focuses – the Arctic Observing Network supports scientific and community-based observations of biodiversity, ecosystems, human societies, land, ice, marine and freshwater systems, and the atmosphere as well as their social, natural, and/or physical environments, so that encompasses a lot right there in just that one program. We’re also working on a way right now to classify the datasets by discipline, so keep an eye out for that coming soon.
-
-
-
-Along with that diversity of disciplines comes a diversity of file types. The most common file type we have are image files in four different file types. Probably less than 200-300 of the datasets have the majority of those images – we have some large datasets that have image and/or audio files from drones. Most of those 6600+ datasets are tabular datasets. There’s a large diversity of data files, though, whether you want to look at remote sensing images, listen to passive acoustic audio files, or run applications – or something else entirely. We also cover a long period of time, at least by human standards. The data represented in our repository spans across centuries.
-
-
-
-We also have data that spans the entire Arctic, as well as the sub-Arctic, regions.
-
-
-
-### Data Discovery Portal
-
-To browse the data catalog, navigate to [arcticdata.io](https://arcticdata.io/). Go to the top of the page and under data, go to search. Right now, you’re looking at the whole catalog. You can narrow your search down by the map area, a general search, or searching by an attribute.
-
-
-Clicking on a dataset brings you to this page. You have the option to download all the files by clicking the green “Download All” button, which will zip together all the files in the dataset to your Downloads folder. You can also pick and choose to download just specific files.
-
-
-
-All the raw data is in open formats to make it easily accessible and compliant with [FAIR](https://www.go-fair.org/fair-principles/) principles – for example, tabular documents are in .csv (comma separated values) rather than Excel documents.
-
-The metrics at the top give info about the number of citations with this data, the number of downloads, and the number of views. This is what it looks like when you click on the Downloads tab for more information.
-
-
-
-Scroll down for more info about the dataset – abstract, keywords. Then you’ll see more info about the data itself. This shows the data with a description, as well as info about the attributes (or variables or parameters) that were measured. The green check mark indicates that those attributes have been annotated, which means the measurements have a precise definition to them. Scrolling further, we also see who collected the data, where they collected it, and when they collected it, as well as any funding information like a grant number. For biological data, there is the option to add taxa.
-
-### Tools and Infrastructure
-
-Across all our services and partnership, we are strongly aligned with the community principles of making data FAIR (Findable, Accesible, Interoperable and Reusable).
-
-
-
-We have a number of tools available to submitters and researchers who are there to download data. We also partner with other organizations, like [Make Data Count](https://makedatacount.org/) and [DataONE](https://www.dataone.org/), and leverage those partnerships to create a better data experience.
-
-
-
-One of those tools is provenance tracking. With provenance tracking, users of the Arctic Data Center can see exactly what datasets led to what product, using the particular script that the researcher ran.
-
-
-
-Another tool are our Metadata Quality Checks. We know that data quality is important for researchers to find datasets and to have trust in them to use them for another analysis. For every submitted dataset, the metadata is run through a quality check to increase the completeness of submitted metadata records. These checks are seen by the submitter as well as are available to those that view the data, which helps to increase knowledge of how complete their metadata is before submission. That way, the metadata that is uploaded to the Arctic Data Center is as complete as possible, and close to following the guideline of being understandable to any reasonable scientist.
-
-
-
-### Support Services
-
-Metadata quality checks are the automatic way that we ensure quality of data in the repository, but the real quality and curation support is done by our curation [team](https://arcticdata.io/team/). The process by which data gets into the Arctic Data Center is iterative, meaning that our team works with the submitter to ensure good quality and completeness of data. When a submitter submits data, our team gets a notification and beings to evaluate the data for upload. They then go in and format it for input into the catalog, communicating back and forth with the researcher if anything is incomplete or not communicated well. This process can take anywhere from a few days or a few weeks, depending on the size of the dataset and how quickly the researcher gets back to us. Once that process has been completed, the dataset is published with a DOI (digital object identifier).
-
-
-
-### Training and Outreach
-
-In addition to the tools and support services, we also interact with the community via trainings like this one and outreach events. We run workshops at conferences like the American Geophysical Union, Arctic Science Summit Week and others. We also run an intern and fellows program, and webinars with different organizations. We’re invested in helping the Arctic science community learn reproducible techniques, since it facilitates a more open culture of data sharing and reuse.
-
-
-
-We strive to keep our fingers on the pulse of what researchers like yourselves are looking for in terms of support. We’re active on [Twitter](https://twitter.com/arcticdatactr) to share Arctic updates, data science updates, and specifically Arctic Data Center updates, but we’re also happy to feature new papers or successes that you all have had with working with the data. We can also take data science questions if you’re running into those in the course of your research, or how to make a quality data management plan. Follow us on Twitter and interact with us – we love to be involved in your research as it’s happening as well as after it’s completed.
-
-
-
-
-### Data Rescue
-
-We also run data rescue operations. We digitiazed Autin Post's collection of glacier photos that were taken from 1964 to 1997. There were 100,000+ files and almost 5 TB of data to ingest, and we reconstructed flight paths, digitized the images of his notes, and documented image metadata, including the camera specifications.
-
-
-
-### Who Must Submit
-
-Projects that have to submit their data include all Arctic Research Opportunities through the NSF Office of Polar Programs. That data has to be uploaded within two years of collection. The Arctic Observing Network has a shorter timeline – their data products must be uploaded within 6 months of collection. Additionally, we have social science data, though that data often has special exceptions due to sensitive human subjects data. At the very least, the metadata has to be deposited with us.
-
-**Arctic Research Opportunities (ARC)**
-
-- Complete metadata and all appropriate data and derived products
-- Within 2 years of collection or before the end of the award, whichever comes first
-
-**ARC Arctic Observation Network (AON)**
-
-- Complete metadata and all data
-- Real-time data made public immediately
-- Within 6 months of collection
-
-**Arctic Social Sciences Program (ASSP)**
-
-- NSF policies include special exceptions for ASSP and other awards that contain sensitive data
-- Human subjects, governed by an Institutional Review Board, ethically or legally sensitive, at risk of decontextualization
-- Metadata record that documents non-sensitive aspects of the project and data
- - Title, Contact information, Abstract, Methods
-
-For more complete information see our "Who Must Submit" [webpage](https://arcticdata.io/submit/#who-must-submit)
-
-Recognizing the importance of sensitive data handling and of ethical treatment of all data, the Arctic Data Center submission system provides the opportunity for researchers to document the ethical treatment of data and how collection is aligned with community principles (such as the CARE principles). Submitters may also tag the metadata according to community develop data sensitivity tags. We will go over these features in more detail shortly.
-
-
-
-### Summary
-
-All the above informtion can be found on our website or if you need help, ask our support team at support@arcticdata.io or tweet us \@arcticdatactr!
-
-
-
diff --git a/materials/sections/closing-materials.Rmd b/materials/sections/closing-materials.Rmd
deleted file mode 100644
index 45a92e12..00000000
--- a/materials/sections/closing-materials.Rmd
+++ /dev/null
@@ -1,35 +0,0 @@
-### Material to include:
-
-- Project Messaging
-- Methods / Analytical Process
-- Next Steps
-
-#### Project Messaging
-
-Present your message box. High Level. You can structure it within the envelope style visual format or as a section based document.
-
-Make sure to include:
-
-- Audience
-- Issue
-- Problem
-- So What?
-- Solution
-- Benefits
-
-
-
-#### Methods / Analytical Process
-
-Provide an update on your approaches to solving your 'Problem'. How are you tackling this? If multiple elements, describe each. Present the workflow for your synthesis.
-
-
-
-#### Next Steps
-
-Articulate your plan for the next steps of the project. Some things to consider as you plan:
-
-- The one-day workshop is January 24th
-- January deliverables include analyses and results
- - What needs do you anticipate for data / code support
-- Project funding ends May 2022
diff --git a/materials/sections/collaboration-social-data-policies.Rmd b/materials/sections/collaboration-social-data-policies.Rmd
deleted file mode 100644
index dd00f42a..00000000
--- a/materials/sections/collaboration-social-data-policies.Rmd
+++ /dev/null
@@ -1,172 +0,0 @@
-
-## Developing a Code of Conduct
-
-Whether you are joining a lab group or establishing a new collaboration, articulating a set of shared agreements about how people in the group will treat each other will help create the conditions for successful collaboration. If agreements or a code of conduct do not yet exist, invite a conversation among all members to create them. Co-creation of a code of conduct will foster collaboration and engagement as a process in and of itself, and is important to ensure all voices heard such that your code of conduct represents the perspectives of your community. If a code of conduct already exists, and your community will be a long-acting collaboration, you might consider revising the code of conduct. Having your group 'sign off' on the code of conduct, whether revised or not, supports adoption of the principles.
-
-When creating a code of conduct, consider both the behaviors you want to encourage and those that will not be tolerated. For example, the Openscapes code of conduct includes Be respectful, honest, inclusive, accommodating, appreciative, and open to learning from everyone else. Do not attack, demean, disrupt, harass, or threaten others or encourage such behavior.
-
-Below are other example codes of conduct:
-
-- [NCEAS Code of Conduct](https://www.nceas.ucsb.edu/sites/default/files/2021-11/NCEAS_Code-of-Conduct_Nov2021_0.pdf)
-- [Carpentries Code of Conduct](https://docs.carpentries.org/topic_folders/policies/code-of-conduct.html)
-- [Arctic Data Center Code of Conduct](https://docs.google.com/document/d/1-eVjnwyLBAfg_f4DRIUVWnLeekgrzrz9wgbhnpOmuVE/edit)
-- [Mozilla Community Participation Guidelines](https://www.mozilla.org/en-US/about/governance/policies/participation/)
-- [Ecological Society of America Code of Conduct](https://www.esa.org/esa/code-of-conduct-for-esa-events/)
-
-
-## Authorship and Credit Policies
-
-
-
-
-Navigating issues of intellectual property and credit can be a challenge, particularly for early career researchers. Open communication is critical to avoiding misunderstandings and conflicts. Talk to your coauthors and collaborators about authorship, credit, and data sharing **early and often**. This is particularly important when working with new collaborators and across lab groups or disciplines which may have divergent views on authorship and data sharing. If you feel uncomfortable talking about issues surrounding credit or intellectual property, seek the advice or assistance of a mentor to support you in having these important conversations.
-
-The “Publication” section of the [Ecological Society of America’s Code of Ethics](https://www.esa.org/about/code-of-ethics/) is a useful starting point for discussions about co-authorship, as are the [International Committee of Medical Journal Editors guidelines](http://www.icmje.org/recommendations/browse/roles-and-responsibilities/defining-the-role-of-authors-and-contributors.html) for authorship and contribution. You should also check guidelines published by the journal(s) to which you anticipate submitting your work.
-
-For collaborative research projects, develop an authorship agreement for your group early in the project and refer to it for each product. This example [authorship agreement](http://training.arcticdata.io/2020-10-arctic/files/template-authorship-policy-ADC-training.docx) from the Arctic Data Center provides a useful template. It builds from information contained within [Weltzin et al (2006)](https://core.ac.uk/download/pdf/215745938.pdf) and provides a rubric for inclusion of individuals as authors. Your collaborative team may not choose to adopt the agreement in the current form, however it will prompt thought and discussion in advance of developing a consensus. Some key questions to consider as you are working with your team to develop the agreement:
-
-- What roles do we anticipate contributors will play? e.g., the NISO [Contributor Roles Taxonomy (CRediT)](https://credit.niso.org/) identifies 14 distinct roles:
-
- - Conceptualization
- - Data curation
- - Formal Analysis
- - Funding acquisition
- - Investigation
- - Methodology
- - Project administration
- - Resources
- - Software
- - Supervision
- - Validation
- - Visualization
- - Writing – original draft
- - Writing – review & editing
-
-- What are our criteria for authorship? (See the [ICMJE guidelines](http://www.icmje.org/recommendations/browse/roles-and-responsibilities/defining-the-role-of-authors-and-contributors.html) for potential criteria)
-- Will we extend the opportunity for authorship to all group members on every paper or product?
-- Do we want to have an opt in or opt out policy? (In an opt out policy, all group members are considered authors from the outset and must request removal from the paper if they don’t want think they meet the criteria for authorship)
-- Who has the authority to make decisions about authorship? Lead author? PI? Group?
-- How will we decide authorship order?
-- In what other ways will we acknowledge contributions and extend credit to collaborators?
-- How will we resolve conflicts if they arise?
-
-
-## Data Sharing and Reuse Policies
-
-As with authorship agreements, it is valuable to establish a shared agreement around handling of data when embarking on collaborative projects. Data collected as part of a funded research activity will typically have been managed as part of the Data Management Plan (DMP) associated with that project. However, collaborative research brings together data from across research projects with different data management plans and can include publicly accessible data from repositories where no management plan is available. For these reasons, a discussion and agreement around the handling of data brought into and resulting from the collaboration is warranted and management of this new data may benefit from going through a data management planning process. Below we discuss example data agreements.
-
-The example data policy [template](http://training.arcticdata.io/2020-10-arctic/files/template-data-policy-ADC-training.docx) provided by the Arctic Data Center addresses three categories of data.
-
-- Individual data not in the public domain
-- Individual data with public access
-- Derived data resulting from the project
-
-For the first category, the agreement considers conditions under which those data may be used and permissions associated with use. It also addresses access and sharing. In the case of individual, publicly accessible data, the agreement stipulates that the team will abide by the attribution and usage policies that the data were published under, noting how those requirements we met. In the case of derived data, the agreement reads similar to a DMP with consideration of making the data public; management, documentation and archiving; pre-publication sharing; and public sharing and attribution. As research data objects receive a persistent identifier (PID), often a DOI, there are citable objects and consideration should be given to authorship of data, as with articles.
-
-The following [example lab policy](https://github.com/temporalecologylab/labgit/blob/master/datacodemgmt/tempeco_DMP.pdf) from the [Wolkovich Lab](http://temporalecology.org/) combines data management practices with authorship guidelines and data sharing agreements. It provides a lot of detail about how this lab approaches data use, attribution and authorship. For example:
-
-#### Section 6: Co-authorship & data {- .aside}
-
-If you agree to take on existing data you cannot offer co-authorship for use of the data unless four criteria are met:
-
-- The co-author agrees to (and does) make substantial intellectual contribution to the work, which includes the reading and editing of all manuscripts on which you are a co-author through the submission-for-publication stage. This includes helping with interpretation of the data, system, study questions.
-- Agreement of co-authorship is made at the start of the project.
-- Agreement is approved of by Lizzie.
-- All data-sharers are given an equal opportunity at authorship. It is not allowed to offer or give authorship to one data-sharer unless all other data-sharers are offered an equal opportunity at authorship—this includes data that are publicly-available, meaning if you offer authorship to one data-sharer and were planning to use publicly-available data you must reach out to the owner of the publicly-available data and strongly offer equivalent authorship as offered to the other data-sharer. As an example, if five people share data freely with you for a meta-analysis and and a sixth wants authorship you either must strongly offer equivalent authorship to all five or deny authorship to the sixth person. Note that the above requirements must also be met in this situation. If one or more datasets are more central or critical to a paper to warrant selective authorship this must be discussed and approved by Lizzie (and has not, to date, occurred within the lab).
-
-#### {-}
-
-#### Policy Preview
-
-
-
-
-
-This policy is communicated with all incoming lab members, from undergraduate to postdocs and visiting scholars, and is shared here with permission from Dr Elizabeth Wolkovich.
-
-
-### Community Principles: CARE and FAIR
-
-The CARE and FAIR Principles were introduced previously in the context of introducing the Arctic Data Center and our data submission and documentation process. In this section we will dive a little deeper.
-
-To recap, the Arctic Data Center is an openly-accessible data repository and the data published through the repository is open for anyone to reuse, subject to conditions of the license (at the Arctic Data Center, data is released under one of two licenses: [CC-0 Public Domain](https://creativecommons.org/publicdomain/zero/1.0/) and [CC-By Attribution 4.0](https://creativecommons.org/licenses/by/4.0/)). In facilitating use of data resources, the data stewardship community have converged on principles surrounding best practices for open data management One set of these principles is the [FAIR principles](https://force11.org/info/guiding-principles-for-findable-accessible-interoperable-and-re-usable-data-publishing-version-b1-0/). FAIR stands for Findable, Accessible, Interoperable, and Reproducible.
-
-
-
-
-The “[Fostering FAIR Data Practices in Europe](https://zenodo.org/record/5837500#.Ygb7VFjMJ0t)” project found that it is more monetarily and timely expensive when FAIR principles are not used, and it was estimated that 10.2 billion dollars per years are spent through “storage and license costs to more qualitative costs related to the time spent by researchers on creation, collection and management of data, and the risks of research duplication.” FAIR principles and open science are overlapping concepts, but are distinctive concepts. Open science supports a culture of sharing research outputs and data, and FAIR focuses on how to prepare the data.
-
-
-
-
-Another set of community developed principles surrounding open data are the [CARE Principles](https://static1.squarespace.com/static/5d3799de845604000199cd24/t/5da9f4479ecab221ce848fb2/1571419335217/CARE+Principles_One+Pagers+FINAL_Oct_17_2019.pdf). The CARE principles for Indigenous Data Governance complement the more data-centric approach of the FAIR principles, introducing social responsibility to open data management practices. The CARE Principles stand for:
-
-- Collective Benefit - Data ecosystems shall be designed and function in ways that enable Indigenous Peoples to derive benefit from the data
-- Authority to Control - Indigenous Peoples’ rights and interests in Indigenous data must be recognised and their authority to control such data be empowered. Indigenous data governance enables Indigenous Peoples and governing bodies to determine how Indigenous Peoples, as well as Indigenous lands, territories, resources, knowledges and geographical indicators, are represented and identified within data.
-- Responsibility - Those working with Indigenous data have a responsibility to share how those data are used to support Indigenous Peoples’ self-determination and collective benefit. Accountability requires meaningful and openly available evidence of these efforts and the benefits accruing to Indigenous Peoples.
-- Ethics - Indigenous Peoples’ rights and wellbeing should be the primary concern at all stages of the data life cycle and across the data ecosystem.
-
-The CARE principles align with the FAIR principles by outlining guidelines for publishing data that is findable, accessible, interoperable, and reproducible while at the same time, accounts for Indigenous’ Peoples rights and interests. Initially designed to support Indigenous data sovereignty, CARE principles are now being adopted across domains, and many researchers argue they are relevant for both Indigenous Knowledge and data, as well as data from all disciplines (Carroll et al., 2021). These principles introduce a “game changing perspective” that encourages transparency in data ethics, and encourages data reuse that is purposeful and intentional that aligns with human well-being aligns with human well-being (Carroll et al., 2021).
-
-## Research Data Publishing Ethics
-
-For over 20 years, the [Committee on Publication Ethics (COPE)](https://publicationethics.org/) has provided trusted guidance on ethical practices for scholarly publishing. The COPE guidelines have been broadly adopted by academic publishers across disciplines, and represent a common approach to identify, classify, and adjudicate potential breaches of ethics in publication such as authorship conflicts, peer review manipulation, and falsified findings, among many other areas. Despite these guidelines, there has been a lack of ethics standards, guidelines, or recommendations for data publications, even while some groups have begun to evaluate and act upon reported issues in data publication ethics.
-
-
-
-To address this gap, the [Force 11 Working Group on Research Data Publishing Ethics](https://force11.org/groups/research-data-publishing-ethics/home/) was formed as a collaboration among research data professionals and the Committee on Publication Ethics (COPE) "to develop industry-leading guidance and recommended best practices to support repositories, journal publishers, and institutions in handling the ethical responsibilities associated with publishing research data." The group released the "Joint FORCE11 & COPE Research Data Publishing Ethics Working Group Recommendations" [@puebla_2021], which outlines recommendations for four categories of potential data ethics issues:
-
-
-
-- [Authorship and Contribution Conflicts](https://zenodo.org/record/5391293/files/Authorship%20%26%20Contributions_datapubethics.pdf?download=1)
- - Authorship omissions
- - Authorship ordering changes / conflicts
- - Institutional investigation of author finds misconduct
-
-- [Legal/regulatory restrictions](https://zenodo.org/record/5391293/files/Legal%20%26%20Regulatory%20Restrictions_datapubethics.pdf?download=1)
- - Copyright violation
- - Insufficient rights for deposit
- - Breaches of national privacy laws (GPDR, CCPA)
- - Breaches of biosafety and biosecurity protocols
- - Breaches of contract law governing data redistribution
-
-- [Risks of publication or release](https://zenodo.org/record/5391293/files/Risk_datapubethics.pdf?download=1)
- - Risks to human subjects
- - Lack of consent
- - Breaches of himan rights
- - Release of personally identifiable information (PII)
- - Risks to species, ecosystems, historical sites
- - Locations of endangered species or historical sites
- - Risks to communities or societies
- - Data harvested for profit or surveillance
- - Breaches of data sovereignty
-
-- [Rigor of published data](https://zenodo.org/record/5391293/files/Rigor_datapubethics.pdf?download=1)
- - Unintentional errors in collection, calculation, display
- - Un-interpretable data due to lack of adequate documentation
- - Errors of of study design and inference
- - Data manipulation or fabrication
-
-Guidelines cover what actions need to be taken, depending on whether the data are already published or not, as well as who should be involved in decisions, who should be notified of actions, and when the public should be notified. The group has also published templates for use by publishers and repositories to announce the extent to which they plan to conform to the data ethics guidelines.
-
-### Discussion: Data publishing policies {.unnumbered .exercise}
-
-At the Arctic Data Center, we need to develop policies and procedures governing how we react to potential breaches of data publication ethics. In this exercise, break into groups to provide advice on how the Arctic Data Center should respond to reports of data ethics issues, and whether we should adopt the Joint FORCE11 & COPE Research Data Publishing Ethics Working Group Policy Templates for repositories. In your discussion, consider:
-
-- Should the repository adopt the [repository policy templates](https://zenodo.org/record/6422102/files/Repository%20Policy%20Template%20v1.pdf?download=1) from Force11?
-- Who should be involved in evaluation of the merits of ethical cases reported to ADC?
-- Who should be involved in deciding the actions to take?
-- What are the range of responses that the repository should consider for ethical breaches?
-- Who should be notified when a determination has been made that a breach has occurred?
-
-You might consider a hypothetical scenario such as the following in considering your response.
-
-> The data coordinator at the Arctic Data Center receives an email in 2022 from a prior postdoctoral fellow who was employed as part of an NSF-funded project on microbial diversity in Alaskan tundra ecosystems. The email states that a dataset from 2014 in the Arctic Data Center was published with the project PI as author, but omits two people, the postdoc and an undergraduate student, as co-authors on the dataset. The PI retired in 2019, and the postdoc asks that they be added to the author list of the dataset to correct the historical record and provide credit.
-
-
-### {-}
-
-## Extra Reading
-
-- [Cheruvelil, K. S., Soranno, P. A., Weathers, K. C., Hanson, P. C., Goring, S. J., Filstrup, C. T., & Read, E. K. (2014). Creating and maintaining high-performing collaborative research teams: The importance of diversity and interpersonal skills. Frontiers in Ecology and the Environment, 12(1), 31-38. DOI: 10.1890/130001](https://esajournals.onlinelibrary.wiley.com/doi/epdf/10.1890/130001)
-- [Carroll, S. R., Garba, I., Figueroa-Rodríguez, O. L., Holbrook, J., Lovett, R., Materechera, S., … Hudson, M. (2020). The CARE Principles for Indigenous Data Governance. Data Science Journal, 19(1), 43. DOI: http://doi.org/10.5334/dsj-2020-043](http://doi.org/10.5334/dsj-2020-043)
diff --git a/materials/sections/collaboration-thinking-preferences-in-person.Rmd b/materials/sections/collaboration-thinking-preferences-in-person.Rmd
deleted file mode 100644
index a77f6696..00000000
--- a/materials/sections/collaboration-thinking-preferences-in-person.Rmd
+++ /dev/null
@@ -1,54 +0,0 @@
-
-## Thinking preferences
-
-### Learning Objectives
-
-An activity and discussion that will provide:
-
-- Opportunity to get to know fellow participants and trainers
-- An introduction to variation in thinking preferences
-
-### Thinking Preferences Activity
-
-Step 1:
-
-- Don't read ahead!! We're headed to the patio.
-
-
-### About the Whole Brain Thinking System
-
-Everyone thinks differently. The way individuals think guides the way they work, and the way groups of individuals think guides how teams work. Understanding thinking preferences facilitates effective collaboration and team work.
-
-The Whole Brain Model, developed by Ned Herrmann, builds upon early conceptualizations of brain functioning. For example, the left and right hemispheres were thought to be associated with different types of information processing while our neocortex and limbic system would regulate different functions and behaviours.
-
-
-
-#### {-}
-
-
-The Herrmann Brain Dominance Instrument (HBDI) provides insight into dominant characteristics based on thinking preferences. There are four major thinking styles that reflect the left cerebral, left limbic, right cerebral and right limbic.
-
-- Analytical (Blue)
-- Practical (Green)
-- Relational (Red)
-- Experimental (Yellow)
-
-
-
-These four thinking styles are characterized by different traits. Those in the BLUE quadrant have a strong logical and rational side. They analyze information and may be technical in their approach to problems. They are interested in the 'what' of a situation. Those in the GREEN quadrant have a strong organizational and sequential side. They like to plan details and are methodical in their approach. They are interested in the 'when' of a situation. The RED quadrant includes those that are feelings-based in their apporach. They have strong interpersonal skills and are good communicators. They are interested in the 'who' of a situation. Those in the YELLOW quadrant are ideas people. They are imaginative, conceptual thinkers that explore outside the box. Yellows are interested in the 'why' of a situation.
-
-
-
-Undertsanding how people think and process information helps us understand not only our own approach to problem solving, but also how individuals within a team can contribute. There is great value in diversity of thinking styles within collaborative teams, each type bringing stengths to different aspects of project development.
-
-
-
-
-Of course, most of us identify with thinking styles in more than one quadrant and these different thinking preferences reflect a complex self made up of our rational, theoretical self; our ordered, safekeeping self; our emotional, interpersonal self; and our imaginitive, experimental self.
-
-
-
-#### Bonus Activity: Your Complex Self
-
-Using the statements contrained within this [document](files/ThinkingPreferencesMapping.pdf), plot the quadrilateral representing your complex self.
-
diff --git a/materials/sections/collaboration-thinking-preferences-short.Rmd b/materials/sections/collaboration-thinking-preferences-short.Rmd
deleted file mode 100644
index ceb17e7b..00000000
--- a/materials/sections/collaboration-thinking-preferences-short.Rmd
+++ /dev/null
@@ -1,54 +0,0 @@
-
-## Thinking preferences
-
-### Learning Objectives
-
-An activity and discussion that will provide:
-
-- Opportunity to get to know fellow participants and trainers
-- An introduction to variation in thinking preferences
-
-### Thinking Preferences Activity
-
-Step 1:
-
-- Read through the statements contained within this [document](files/ThinkingPreferencesMapping.pdf) and determine which descriptors are most like you. Make a note of them.
-- Review the descriptors again and determine which are quite like you.
-- You are working towards identifying your top 20. If you have more than 20, discard the descriptors that resonate the least.
-- Using the letter codes in the right hand column, count the number of descriptors that fall into the categories A B C and D.
-
-Step 2: Scroll to the second page and copy the graphic onto a piece of paper, completing the quadrant with your scores for A, B, C and D.
-
-Step 3: Reflect and share out: Do you have a dominant letter? Were some of the statements you included in your top 20 easier to resonate with than others? Were you answering based on how you *are* or how you wish to be?
-
-
-### About the Whole Brain Thinking System
-
-Everyone thinks differently. The way individuals think guides the way they work, and the way groups of individuals think guides how teams work. Understanding thinking preferences facilitates effective collaboration and team work.
-
-The Whole Brain Model, developed by Ned Herrmann, builds upon early conceptualizations of brain functioning. For example, the left and right hemispheres were thought to be associated with different types of information processing while our neocortex and limbic system would regulate different functions and behaviours.
-
-
-
-The Herrmann Brain Dominance Instrument (HBDI) provides insight into dominant characteristics based on thinking preferences. There are four major thinking styles that reflect the left cerebral, left limbic, right cerebral and right limbic.
-
-- Analytical (Blue)
-- Practical (Green)
-- Relational (Red)
-- Experimental (Yellow)
-
-
-
-These four thinking styles are characterized by different traits. Those in the BLUE quadrant have a strong logical and rational side. They analyze information and may be technical in their approach to problems. They are interested in the 'what' of a situation. Those in the GREEN quadrant have a strong organizational and sequential side. They like to plan details and are methodical in their approach. They are interested in the 'when' of a situation. The RED quadrant includes those that are feelings-based in their apporach. They have strong interpersonal skills and are good communicators. They are interested in the 'who' of a situation. Those in the YELLOW quadrant are ideas people. They are imaginative, conceptual thinkers that explore outside the box. Yellows are interested in the 'why' of a situation.
-
-
-
-Most of us identify with thinking styles in more than one quadrant and these different thinking preferences reflect a complex self made up of our rational, theoretical self; our ordered, safekeeping self; our emotional, interpersonal self; and our imaginitive, experimental self.
-
-
-
-Undertsanding the complexity of how people think and process information helps us understand not only our own approach to problem solving, but also how individuals within a team can contribute. There is great value in diversity of thinking styles within collaborative teams, each type bringing stengths to different aspects of project development.
-
-
-
-
diff --git a/materials/sections/collaboration-thinking-preferences.Rmd b/materials/sections/collaboration-thinking-preferences.Rmd
deleted file mode 100644
index 1bcd8e1b..00000000
--- a/materials/sections/collaboration-thinking-preferences.Rmd
+++ /dev/null
@@ -1,57 +0,0 @@
-
-## Thinking preferences
-
-### Learning Objectives
-
-An activity and discussion that will provide:
-
-- Opportunity to get to know fellow participants and trainers
-- An introduction to variation in thinking preferences
-
-### Thinking Preferences Activity
-
-Step 1: Don't jump ahead in this document. (Did I just jinx it?)
-
-Step 2: Review the [list of statements here](files/HBDIStatements.pdf) and reflect on your traits. Do you learn through structured activities? Are you conscious of time and are punctual? Are you imaginative? Do you like to take risks?
-Determine the three statements that resonate most with you and record them. Note the symbol next to each of them.
-
-Step 3: Review the [symbol key here](files/HBDIkey.pdf) and assign a color to each of your three remaining statements. Which is your dominant color or are you a mix of three?
-
-Step 4: Using the zoom breakout room feature, move between the five breakout rooms and talk to other participants about their dominant color statements. Keep moving until you cluster into a group of 'like' dominant colors. If you are a mix of three colors, find other participants that are also a mix.
-
-Step 5: When the breakout rooms have reached stasis, each group should note the name and dominant color of your breakout room in slack.
-
-Step 6: Take a moment to reflect on one of the statements you selected and share with others in your group. Why do you identify strongly with this trait? Can you provide an example that illustrates this in your life?
-
-### About the Whole Brain Thinking System
-
-Everyone thinks differently. The way individuals think guides the way they work, and the way groups of individuals think guides how teams work. Understanding thinking preferences facilitates effective collaboration and team work.
-
-The Whole Brain Model, developed by Ned Herrmann, builds upon our understanding of brain functioning. For example, the left and right hemispheres are associated with different types of information processing and our neocortex and limbic system regulate different functions and behaviours.
-
-
-
-The Herrmann Brain Dominance Instrument (HBDI) provides insight into dominant characteristics based on thinking preferences. There are four major thinking styles that reflect the left cerebral, left limbic, right cerebral and right limbic.
-
-- Analytical (Blue)
-- Practical (Green)
-- Relational (Red)
-- Experimental (Yellow)
-
-
-
-These four thinking styles are characterized by different traits. Those in the BLUE quadrant have a strong logical and rational side. They analyze information and may be technical in their approach to problems. They are interested in the 'what' of a situation. Those in the GREEN quadrant have a strong organizational and sequential side. They like to plan details and are methodical in their approach. They are interested in the 'when' of a situation. The RED quadrant includes those that are feelings-based in their apporach. They have strong interpersonal skills and are good communicators. They are interested in the 'who' of a situation. Those in the YELLOW quadrant are ideas people. They are imaginative, conceptual thinkers that explore outside the box. Yellows are interested in the 'why' of a situation.
-
-
-
-Most of us identify with thinking styles in more than one quadrant and these different thinking preferences reflect a complex self made up of our rational, theoretical self; our ordered, safekeeping self; our emotional, interpersonal self; and our imaginitive, experimental self.
-
-
-
-Undertsanding the complexity of how people think and process information helps us understand not only our own approach to problem solving, but also how individuals within a team can contribute. There is great value in diversity of thinking styles within collaborative teams, each type bringing stengths to different aspects of project development.
-
-
-
-### Bonus Activity: Your Complex Self
-
-Using the statements contrained within this [document](files/ThinkingPreferencesMapping.pdf), plot the quadrilateral representing your complex self.
diff --git a/materials/sections/communication.Rmd b/materials/sections/communication.Rmd
deleted file mode 100644
index f71618d7..00000000
--- a/materials/sections/communication.Rmd
+++ /dev/null
@@ -1,115 +0,0 @@
-## Communication Principles and Practices
-
-*"The ingredients of good science are obvious—novelty of research topic, comprehensive coverage of the relevant literature, good data, good analysis including strong statistical support, and a thought-provoking discussion. The ingredients of good science reporting are obvious—good organization, the appropriate use of tables and figures, the right length, writing to the intended audience— do not ignore the obvious" - Bourne 2005*
-
-### Scholarly publications
-
-Peer-reviewed publication remains a primary mechanism for direct and efficient communication of research findings. Other scholarly communications include abstracts, technical reports, books and book chapters. These communications are largely directed towards students and peers; individuals learning about or engaged in the process of scientific research whether in a university, non-profit, agency, commercial or other setting. In this section we will focus less on peer-reviewed publications and more on messaging in general, whether for publications, reports, articles or social media. That said, the following tabling is a good summary of '10 Simple Rules' for writing research papers (adapted from [Zhang 2014](https://journals.plos.org/ploscompbiol/article?id=10.1371/journal.pcbi.1003453), published in [Budden and Michener, 2017](https://link.springer.com/chapter/10.1007%2F978-3-319-59928-1_14))
-
-1. **Make it a Driving Force** "design a project with an ultimate paper firmly in mind”
-2. **Less Is More** "fewer but more significant papers serve both the research community and one’s career better than more papers of less significance”
-3. **Pick the Right Audience** “This is critical for determining the organization of the paper and the level of detail of the story, so as to write the paper with the audience in mind.”
-4. **Be Logical** “The foundation of ‘‘lively’’ writing for smooth reading is a sound and clear logic underlying the story of the paper.” “An effective tactic to help develop a sound logical flow is to imaginatively create a set of figures and tables, which will ultimately be developed from experimental results, and order them in a logical way based on the information flow through the experiments.”
-5. **Be Thorough and Make It Complete** Present the central underlying hypotheses; interpret the insights gleaned from figures and tables and discuss their implications; provide sufficient context so the paper is self-contained; provide explicit results so readers do not need to perform their own calculations; and include self-contained figures and tables that are described in clear legends
-6. **Be Concise** “the delivery of a message is more rigorous if the writing is precise and concise”
-7. **Be Artistic** “concentrate on spelling, grammar, usage, and a ‘‘lively’’ writing style that avoids successions of simple, boring, declarative sentences”
-8. **Be Your Own Judge** Review, revise and reiterate. “…put yourself completely in the shoes of a referee and scrutinize all the pieces—the significance of the work, the logic of the story, the correctness of the results and conclusions, the organization of the paper, and the presentation of the materials.”
-9. **Test the Water in Your Own Backyard** “…collect feedback and critiques from others, e.g., colleagues and collaborators.”
-10. **Build a Virtual Team of Collaborators** Treat reviewers as collaborators and respond objectively to their criticisms and recommendations. This may entail redoing research and thoroughly re-writing a paper.
-
-### Other communications
-
-Communicating your research outside of peer-reviewed journal articles is increasingly common, and important. These non academic communications can reach a more broad and diverse audience than traditional publications (and should be tailored for specific audience groups accordingly), are not subject to the same pay-walls as journal articles, and can augment and amplify scholarly publications. For example, this twitter announcement from Dr Heather Kropp, providing a synopsis of their synthesis publication in Environmental Research (note the reference to a repository where the data are located, though a DOI would be better).
-
-
-Whether this communication occurs through blogs, social media, or via interviews with others, developing practices to refine your messaging is critical for successful communication. One tool to support your communication practice is 'The Message Box' developed by COMPASS, an organization that helps scientists develop communications skills in order to share their knowledge and research across broad audiences without compromising the accuracy of their research.
-
-### The Message Box
-
-
-
-
-
-The [Message Box](https://www.compassscicomm.org/leadership-development/the-message-box/) is a tool that helps researchers take the information they hold about their research and communicate it in a way that resonates with the chosen audience. It can be used to help prepare for interviews with journalists or employers, plan for a presentation, outline a paper or lecture, prepare a grant proposal, or clearly, and with relevancy, communicate your work to others. While the message box *can* be used in all these ways, you must first identify the audience for your communication.
-
-The Message Box comprises five sections to help you sort and distill your knowledge in a way that will resonate with your (chosen) audience. How we communicate with other scientists (through scholarly publications) is not how the rest of the rest of the world typically communicates. In a scientific paper, we establish credibility in the introduction and methods, provide detailed data and results, and then share the significance of our work in the discussion and conclusions. But the rest of the world leads with the impact, the take home message. A quick glance of newspaper headlines demonstrates this.
-
-
-
-The five sections of the Message Box are provided below. For a detailed explanation of the sections and guidance on how to use the Message Box, work through the [Message Box Workbook](https://www.compassscicomm.org/wp-content/uploads/2020/05/The-Message-Box-Workbook.pdf)
-
-#### Message Box Sections
-
-**The Issue**
-
-The Issue section in the center of the box identifies and describes the overarching issue or topic that you’re addressing in broad terms. It’s the big-picture context of your work. This should be very concise and clear; no more than a short phrase. You might find you revisit the Issue after you’ve filled out your Message Box, to see if your thinking on the overarching topic has changed since you started.
-
-- Describes the overarching issue or topic: Big Picture
-- Broad enough to cover key points
-- Specific enough to set up what's to come
-- Concise and clear
-- 'Frames' the rest of the message box
-
-**The Problem**
-
-The Problem is the chunk of the broader issue that you’re addressing in your area of expertise. It’s your piece of the pie, reflecting your work and expert knowledge. Think about your research questions and what aspect of the specific problem you’re addressing would matter to your audience. The Problem is also where you set up the So What and describe the situation you see and want to address.
-
-- The part of the broader issue that your work is addressing
-- Builds upon your work and expert knowledge
-- Try to focus on one problem per audience
-- Often the ***Problem*** is your research question
-- This section sets you up for ***So What***
-
-**The So What**
-
-The crux of the Message Box, and the critical question the COMPASS team seeks to help scientists answer, is “So what?”
-Why should your audience care? What about your research or work is important for them to know? Why are you talking to them about it? The answer to this question may change from audience to audience, and you’ll want to be able to adjust based on their interests and needs. We like to use the analogy of putting a message through a prism that clarifies the importance to different audiences. Each audience will be interested in different facets of your work, and you want your message to reflect their interests and accommodate their needs. The prism below includes a spectrum of audiences you might want to reach, and some of the questions they might have about your work.
-
-- This is the crux of the message box
-- Why should you audience care?
-- What about your research is important for them to know?
-- Why are you talking to them about it?
-
-
-
-**The Solution**
-
-The Solution section outlines the options for solving the problem you identified. When presenting possible solutions, consider whether they are something your audience can influence or act upon. And remind yourself of your communication goals: Why are you communicating with this audience? What do you want to accomplish?
-
-- Outlines the options for solving the ***Problem ***
-- Can your audience influence or act upon this?
-- There may be multiple solutions
-- Make sure your ***Solution*** relates back to the ***Problem***. Edit one or both as needed
-
-**The Benefit**
-
-In the Benefit section, you list the benefits of addressing the Problem — all the good things that could happen if your Solution section is implemented. This ties into the So What of why your audience cares, but focuses on the positive results of taking action (the So What may be a negative thing — for example, inaction could lead to consequences that your audience cares about). If possible, it can be helpful to be specific here — concrete examples are more compelling than abstract. Who is likely to benefit, and where, and when?
-
-- What are the benefits of addressing the ***Problem***?
-- What good things come from implementing your ***Solution***?
-- Make sure it connects with your ***So What***
-- ***Benefits*** and ***So What*** may be similar
-
-**Finally**, to make you message more memorable you should:
-
-- Support your message with data
-- Limit the use of numbers and statistics
-- Use specific examples
-- Compare numbers to concepts, help people relate
-- Avoid jargon
-- Lead with what you know
-
-
-
-In addition to the [Message Box Workbook](https://www.compassscicomm.org/wp-content/uploads/2020/05/The-Message-Box-Workbook.pdf), COMPASS have resources on how to [increase the impact](https://www.compassscicomm.org/practice/) of your message (include important statistics, draw comparisons, reduce jargon, use examples), exercises for practicing and [refining](https://www.compassscicomm.org/compare/) your message and published [examples](https://www.compassscicomm.org/examples/).
-
-
-### Resources
-
-
-
DataONE Webinar: Communication Strategies to Increase Your Impact from DataONE on Vimeo.
-
-- Budden, AE and Michener, W (2017) [Communicating and Disseminating Research Findings](https://link.springer.com/chapter/10.1007%2F978-3-319-59928-1_14). In: Ecological Informatics, 3rd Edition. Recknagel, F, Michener, W (eds.) Springer-Verlag
-- COMPASS [Core Principles of Science Communication](https://www.compassscicomm.org/core-principles-of-science-communication-2/)
-- [Example Message Boxes](https://www.compassscicomm.org/examples/)
-
diff --git a/materials/sections/data-ethics-eloka-2023.Rmd b/materials/sections/data-ethics-eloka-2023.Rmd
deleted file mode 100644
index d976306c..00000000
--- a/materials/sections/data-ethics-eloka-2023.Rmd
+++ /dev/null
@@ -1,228 +0,0 @@
-## Introduction
-
-This part of the course was developed with input from ELOKA and the NNA-CO, and is a work-in-progress. The training introduces ethics issues in a broad way and includes discussion of social science data and open science, but the majority of the section focuses on issues related to research with, by, and for Indigenous communities. We recognize that there is a need for more in-depth training and focus on open science for social scientists and others who are not engaging with Indigenous Knowledge holders and Indigenous communities, and hope to develop further resources in this area in the future. **Many of the data stewardship practices that have been identified as good practices through Indigenous Data Sovereignty framework development are also relevant for those working with Arctic communities that are not Indigenous, although the rights frameworks and collective ownership is specific to the Indigenous context.**
-
-The examples we include in this training are primarily drawn from the North American research context. In future trainings, we plan to expand and include examples from other Indigenous Arctic contexts. **We welcome suggestions and resources that would strengthen this training for audiences outside of North America.**
-
-We also recognize the importance of trainings on Indigenous data sovereignty and ethics that are being developed and facilitated by Indigenous organizations and facilitators. In this training we offer some introductory material but there is much more depth offered in IDS specific trainings.
-
-## Introduction to ELOKA
-
-The Exchange for Local Observations and Knowledge of the Arctic is an NSF funded project. ELOKA partners with Indigenous communities in the Arctic to create online products that facilitate the collection, preservation, exchange, and use of local observations and Indigenous Knowledge of the Arctic. ELOKA fosters collaboration between resident Arctic experts and visiting researchers, provides data management and user support, and develops digital tools for Indigenous Knowledge in collaboration with our partners. By working together, Arctic residents and researchers can make significant contributions to a deeper understanding of the Arctic and the social and environmental changes ongoing in the region.
-
-Arctic residents and Indigenous peoples have been increasingly involved in, and taking control of, research. Through Local and Indigenous Knowledge and community-based monitoring, Arctic communities have made, and continue to make, significant contributions to understanding recent Arctic change. In ELOKA's work, we subscribe to ideas of information and data sovereignty, in that we want our projects to be community-driven with communities having control over how their data, information, and knowledge are shared in an ethical manner.
-
-A key challenge of Local and Indigenous Knowledge research and community-based monitoring to date is having an effective and appropriate means of recording, storing, representing, and managing data and information in an ethical manner. Another challenge is to find an effective means of making such data and information available to Arctic residents and researchers, as well as other interested groups such as teachers, students, and decision-makers. Without a network and data management system to support Indigenous Knowledge and community-based research, a number of problems have arisen, such as misplacement or loss of extremely precious data, information, and stories from Elders who have passed away, a lack of awareness of previous studies causing repetition of research and wasted resources occurring in the same communities, and a reluctance or inability to initiate or maintain community-based research without an available data management system. Thus, there is an urgent need for effective and appropriate means of recording, preserving, and sharing the information collected in Arctic communities. ELOKA aims to fill this gap by partnering with Indigenous communities to ensure their knowledge and data are stored in an ethical way, thus ensuring sovereignty over these valuable sources of information.
-
-ELOKA's overarching philosophy is that Local and Indigenous Knowledge and scientific expertise are complementary and reinforcing ways of understanding the Arctic system. Collecting, documenting, preserving, and sharing knowledge is a cooperative endeavor, and ELOKA is dedicated to fostering that shared knowledge between Arctic residents, scientists, educators, policy makers, and the general public. ELOKA operates on the principle that all knowledge should be treated ethically, and intellectual property rights should be respected.
-
-ELOKA is a service available for research projects, communities, organizations, schools, and individuals who need help storing, protecting, and sharing Local and Indigenous Knowledge. ELOKA works with many different types of data and information, including:
-
-- Written interview transcripts
-
-- Audio or video tapes and files
-
-- Photographs, artwork, illustrations, and maps
-
-- Digital geographic information such as GPS tracks, and data created using Geographic Information Systems
-
-- Quantitative data such as temperature, snow thickness, wind data, etc.
-
-- Many other types of Indigenous Knowledge and local observations, including place names
-
-ELOKA collaborates with other organizations engaged in addressing data management issues for community-based research. Together, we are working to build a community that facilitates international knowledge exchange, development of resources, and collaboration focused on local communities and stewardship of their data, information, and knowledge.
-
-## Working With Arctic Communities
-
-Arctic communities (defined as a place and the people who live there, based on geographic location in the Arctic/sub-Arctic) are involved in research in diverse ways - as hosts to visiting or non-local researchers, as well as "home" to community researchers who are leading or collaborating on research projects. **Over the past decades, community voices of discontent with standard research practices that are often exclusive and perpetuate inequities have grown stronger**. The Arctic research community (defined more broadly as the range of institutions, organizations, researchers and local communities involved in research) is in the midst of a complex conversation about equity in research aimed at transforming research practice to make it more equitable and inclusive.
-
-**One of the drivers of community concerns is the colonial practice of extracting knowledge from a place or group of people without respect for local norms of relationship with people and place, and without an ethical commitment to sharing and making benefits of knowledge accessible and accountable to that place.** Such approaches to knowledge and data extraction follow hundreds of years of exploration and research that viewed science as a tool of "Enlightenment" yet focused exclusively on benefits to White, European (or "southern" from an Arctic community perspective) researchers and scientists. This prioritization of non-local perspectives and needs (to Arctic communities) continues in Arctic research.
-
-**One result of this approach to research has been a lack of access for Arctic residents to the data and knowledge that have resulted from research conducted in their own communities.** Much of this data was stored in the personal files or hard drives of researchers, or in archives located in urban centers far from the Arctic.
-
-## Indigenous Data Governance and Sovereignty
-
-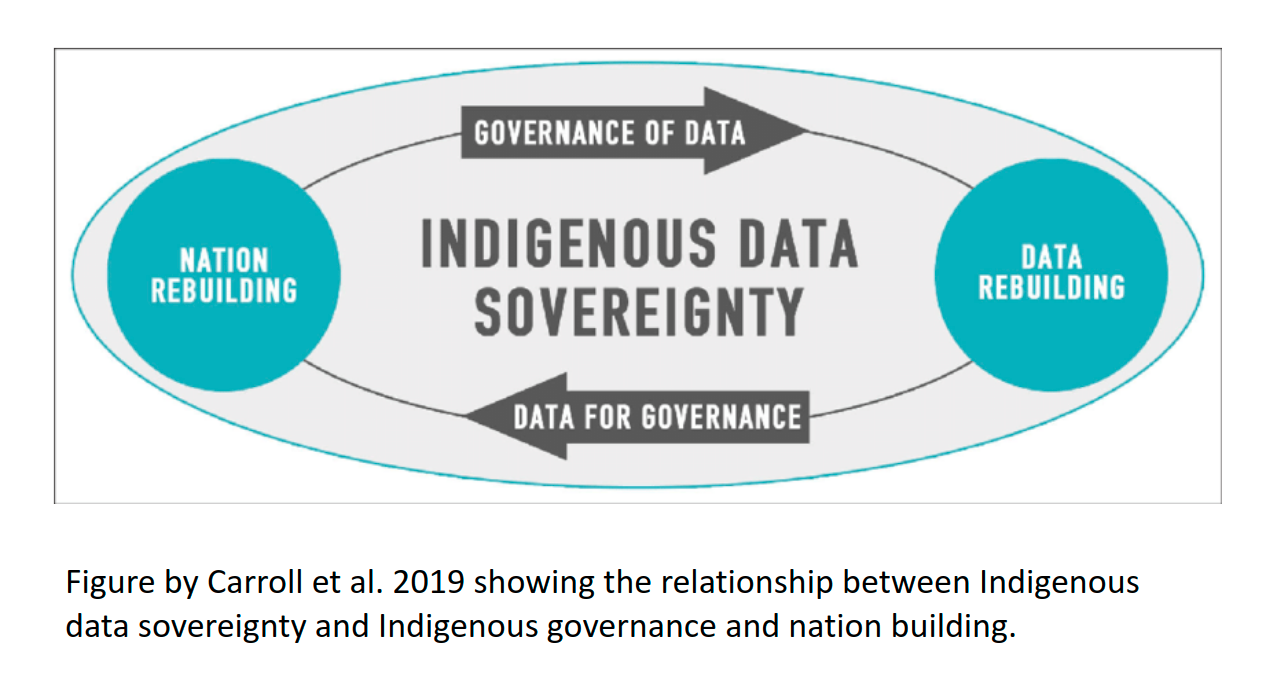
-
-**All governing entities, whether national, state, local, or tribal, need access to good, current, relevant data in order to make policy, planning, and programmatic decisions.** Indigenous nations and organizations have had to push for data about their peoples and communities to be collected and shared in ethical and culturally appropriate ways, and they have also had to fight for resources and capacity to develop and lead their own research programs.
-
-#### **Indigenous Data Definitions**
-
-**Indigenous data sovereignty** "...refers to the right of Indigenous peoples to govern the collection, ownership, and application of data about Indigenous communities, peoples, lands, and resources (Rainie et al. 2019). These governance rights apply "regardless of where/by whom data is held (Rainie et al. 2019).
-
-Some Indigenous individuals and communities have expressed dissatisfaction with the term "data" as being too narrowly focused and abstract to represent the embedded and holistic nature of knowledge in Indigenous communities. **Knowledge sovereignty** is a related term that has a similar meaning but is framed more broadly, and has been defined as:
-
-"Tribal communities having control over the documentation and production of knowledge (such as through research activities) which relate to Alaska Native people and the resources they steward and depend on" (Kawerak 2021).
-
-**Indigenous data** is "data in a wide variety of formats inclusive of digital data and data as knowledge and information. It encompasses data, information, and knowledge about Indigenous individuals, collectives, entities, lifeways, cultures, lands, and resources." (Rainie et al. 2019)
-
-**Indigenous data governance** is "The entitlement to determine how Indigenous data is governed and stewarded" (Rainie et al. 2019)
-
-## CARE Principles
-
-In facilitating use of data resources, the data stewardship community have converged on principles surrounding best practices for open data management One set of these principles is the FAIR principles. FAIR stands for Findable, Accessible, Interoperable, and Reproducible.
-
-The FAIR (Findable, Accessible, Interoperable, Reproducible) principles for data management are widely known and broadly endorsed.
-
-FAIR principles and open science are overlapping concepts, but are distinctive concepts. Open science supports a culture of sharing research outputs and data, and FAIR focuses on how to prepare the data. **The FAIR principles place emphasis on machine readability, "distinct from peer initiatives that focus on the human scholar" (Wilkinson et al 2016) and as such, do not fully engage with sensitive data considerations and with Indigenous rights and interests (Research Data Alliance International Indigenous Data Sovereignty Interest Group, 2019)**. Research has historically perpetuated colonialism and represented extractive practices, meaning that the research results were not mutually beneficial. These issues also related to how data was owned, shared, and used.
-
-**To address issues like these, the Global Indigenous Data Alliance (GIDA) introduced CARE Principles for Indigenous Data Governance to support Indigenous data sovereignty**. To many, the FAIR and CARE principles are viewed by many as complementary: CARE aligns with FAIR by outlining guidelines for publishing data that contributes to open-science and at the same time, accounts for Indigenous' Peoples rights and interests. CARE Principles for Indigenous Data Governance stand for Collective Benefit, Authority to Control, Responsibility, Ethics. The CARE principles for Indigenous Data Governance complement the more data-centric approach of the FAIR principles, introducing social responsibility to open data management practices. These principles ask researchers to put human well-being at the forefront of open-science and data sharing (Carroll et al., 2021; Research Data Alliance International Indigenous Data Sovereignty Interest Group, September 2019).
-
-Indigenous data sovereignty and considerations related to working with Indigenous communities are particularly relevant to the Arctic. The CARE Principles stand for:
-
-- **Collective Benefit** - Data ecosystems shall be designed and function in ways that enable Indigenous Peoples to derive benefit from the data for:
-
- - Inclusive development/innovation
-
- - Improved governance and citizen engagement
-
- - Equitable outcomes
-
-- **Authority to Control** - Indigenous Peoples' rights and interests in Indigenous data must be recognised and their authority to control such data be empowered. Indigenous data governance enables Indigenous Peoples and governing bodies to determine how Indigenous Peoples, as well as Indigenous lands, territories, resources, knowledges and geographical indicators, are represented and identified within data.
-
- - Recognizing Indigenous rights (individual and collective) and interests
-
- - Data for governance
-
- - Governance of data
-
-- **Responsibility** - Those working with Indigenous data have a responsibility to share how those data are used to support Indigenous Peoples' self-determination and collective benefit. Accountability requires meaningful and openly available evidence of these efforts and the benefits accruing to Indigenous Peoples.
-
- - For positive relationships
-
- - For expanding capability and capacity (enhancing digital literacy and digital infrastructure)
-
- - For Indigenous languages and worldviews (sharing data in Indigenous languages)
-
-- **Ethics** - Indigenous Peoples' rights and wellbeing should be the primary concern at all stages of the data life cycle and across the data ecosystem.
-
- - Minimizing harm/maximizing benefit - not using a "deficit lens" that conceives of and portrays Indigenous communities as dysfunctional, lacking solutions, and in need of intervention. For researchers, adopting a deficit lens can lead to collection of only a subset of data while excluding other data and information that might identify solutions, innovations, and sources of resilience from within Indigenous communities. For policy makers, a deficit lens can lead to harmful interventions framed as "helping."
-
- - For justice - addressing power imbalances and equity
-
- - For future use - acknowledging potential future use/future harm. Metadata should acknowledge provenance and purpose and any limitations in secondary use inclusive of issues of consent.
-
-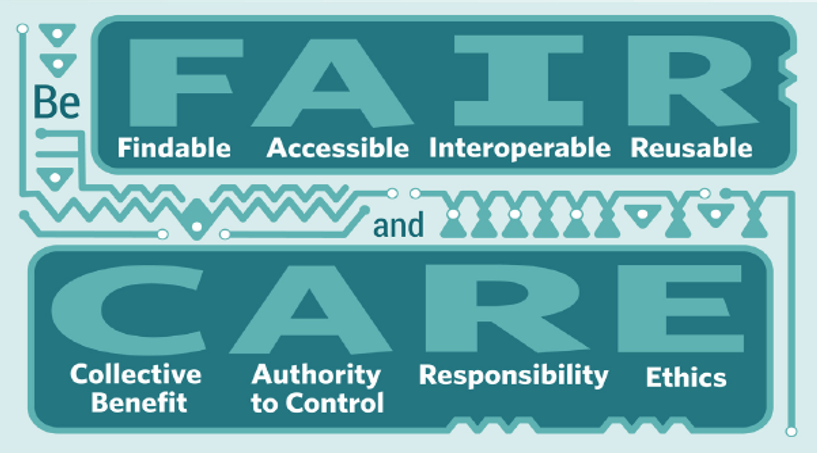
-
-**Sharing sensitive data introduces unique ethical considerations, and FAIR and CARE principles speak to this by recommending sharing anonymized metadata to encourage discover ability and reduce duplicate research efforts, following consent of rights holders (Puebla & Lowenberg, 2021)**. While initially designed to support Indigenous data sovereignty, CARE principles are being adopted more broadly and researchers argue they are relevant across all disciplines (Carroll et al., 2021). As such, these principles introduce a "game changing perspective" for all researchers that encourages transparency in data ethics, and encourages data reuse that is both purposeful and intentional and that aligns with human well-being (Carroll et al., 2021). Hence, to enable the research community to articulate and document the degree of data sensitivity, and ethical research practices, the Arctic Data Center has introduced new submission requirements.
-
-\
-\
-
-### Discussion Questions:
-
-1. Do any of the practices of your data management workflow reflect the CARE Principles, or incorporate aspects of them?
-
-\
-
-### Examples from ELOKA
-
-#### Nunaput Atlas
-
-
-
-\
-
-Nunaput translates to "our land" in Cup'ik, the Indigenous language of Chevak, Alaska. Chevak or Cev'aq means "cut through channel" and refers to the creation of a short cut between two rivers. Chevak is a Cup'ik community, distinct from the Yup'ik communities that surround it, located in the Yukon-Kuskokwim Delta region of Western Alaska.
-
-The Nunaput Atlas is a community driven, interactive, online atlas for the Chevak Traditional Council and Chevak community members to create a record of observations, knowledge, and share stories about their land. The Nunaput Atlas is being developed in collaboration with the Exchange for Local Observations and Knowledge of the Arctic (ELOKA) and the U.S. Geological Survey (USGS). The community of Chevak has been involved in a number of community-based monitoring and research projects with the USGS and the Yukon River Inter-Tribal Watershed Council (YRITWC) over the years. The monitoring data collected by the Chevak Traditional Council's Environmental department as well as results from research projects are also presented in this atlas.
-
-All atlases are created uniquely and data ethics issues and privacy are presented. There is no standard template for user agreements that all atlases adopt: each user agreement is designed by the community, and it isdesigned specific to their needs. Nunaput Atlas has a public view, but is primarily used on the private, password protected side.
-
-#### Yup'ik Atlas
-
-
-
-Yup'ik Atlas is a great example of the data owners and community wanting the data to be available and public. Part of Indigenous governance and data governance is about having good data, to enable decision making. The Yup'ik atlas has many aspects and uses, and one of the primary uses is for it to be integrated into the regional curriculum to engage youth.
-
-#### AAOKH
-
-
-
-The Alaska Arctic Observatory and Knowledge Hub is a great example of the continual correspondence and communication between researchers and community members/knowledge holders once the data is collected. Specifically, AAOKH has dedicated a lot of time and in person meetings to creating a data citation that best reflects what the community members want.
-
-\
-
-### Final Questions
-
-1. Do CARE Principles apply to your research? Why or why not?
-
-2. Are there any limitations or barriers to adopting CARE Principles?
-
-### Data Ethics Resources
-
-**Trainings:**
-
-Fundamentals of OCAP (online training - for working with First Nations in Canada):
-
-Native Nations Institute trainings on Indigenous Data Sovereignty and Indigenous Data Governance:
-
-The [Alaska Indigenous Research Program](https://anthc.org/alaska-indigenous-research-program/), is a collaboration between the Alaska Native Tribal Health Consortium (ANTHC) and Alaska Pacific University (APU) to increase capacity for conducting culturally responsive and respectful health research that addresses the unique settings and health needs of Alaska Native and American Indian People. The 2022 program runs for three weeks (May 2 - May 20), with specific topics covered each week. Week two (Research Ethics) may be of particular interest. Registration is free.
-
-The r-ETHICS training (Ethics Training for Health in Indigenous Communities Study) is starting to become an acceptable, recognizable CITI addition for IRB training by tribal entities.
-
-Kawerak, Inc and First Alaskans Institute have offered trainings in research ethics and Indigenous Data Sovereignty. Keep an eye out for further opportunities from these Alaska-based organizations.
-
-**On open science and ethics:**
-
-
-
-ON-MERRIT recommendations for maximizing equity in open and responsible research
-
-
-
-
-
-**Arctic social science and data management:**
-
-Arctic Horizons report: Anderson, S., Strawhacker, C., Presnall, A., et al. (2018). Arctic Horizons: Final Report. Washington D.C.: Jefferson Institute.
-
-Arctic Data Center workshop report:
-
-**Arctic Indigenous research and knowledge sovereignty frameworks, strategies and reports:**
-
-Kawerak, Inc. (2021) [Knowledge & Research Sovereignty Workshop](https://kawerak.org/download/kawerak-knowledge-and-research-sovereignty-ksi-workshop-report/) May 18-21, 2021 Workshop Report. Prepared by Sandhill.Culture. Craft and Kawerak Inc. Social Science Program. Nome, Alaska.
-
-Inuit Circumpolar Council. 2021. Ethical and Equitable Engagement Synthesis Report: A collection of Inuit rules, guidelines, protocols, and values for the engagement of Inuit Communities and Indigenous Knowledge from Across Inuit Nunaat. Synthesis Report. International.
-
-Inuit Tapiriit Kanatami. 2018. National Inuit Strategy on Research. Accessed at:
-
-**Indigenous Data Governance and Sovereignty:**
-
-McBride, K. [Data Resources and Challenges for First Nations Communities](https://www.afnigc.ca/main/includes/media/pdf/digital%20reports/Data_Resources_Report.pdf). Document Review and Position Paper. Prepared for the Alberta First Nations Information Governance Centre.
-
-Carroll, S.R., Garba, I., Figueroa-Rodríguez, O.L., Holbrook, J., Lovett, R., Materechera, S., Parsons, M., Raseroka, K., Rodriguez-Lonebear, D., Rowe, R., Sara, R., Walker, J.D., Anderson, J. and Hudson, M., 2020. The CARE Principles for Indigenous Data Governance. Data Science Journal, 19(1), p.43. DOI:
-
-Kornei, K. (2021), Academic citations evolve to include Indigenous oral teachings, Eos, 102, . Published on 9 November 2021.
-
-Kukutai, T. & Taylor, J. (Eds.). (2016). Indigenous data sovereignty: Toward an agenda. Canberra: Australian National University Press. See the editors' Introduction and Chapter 7.
-
-Kukutai, T. & Walter, M. (2015). Indigenising statistics: Meeting in the recognition space. Statistical Journal of the IAOS, 31(2), 317--326.
-
-Miaim nayri Wingara Indigenous Data Sovereignty Collective and the Australian Indigenous Governance Institute. (2018). Indigenous data sovereignty communique. Indigenous Data Sovereignty Summit, 20 June 2018, Canberra.
-
-National Congress of American Indians. (2018). Resolution KAN-18-011: Support of US Indigenous data sovereignty and inclusion of tribes in the development of tribal data governance principles.
-
-Rainie, S., Kukutai, T., Walter, M., Figueroa-Rodriguez, O., Walker, J., & Axelsson, P. (2019) Issues in Open Data - Indigenous Data Sovereignty. In T. Davies, S. Walker, M. Rubinstein, & F. Perini (Eds.), The State of Open Data: Histories and Horizons. Cape Town and Ottawa: African Minds and International Development Research Centre.
-
-Schultz, Jennifer Lee, and Stephanie Carroll Rainie. 2014. "The Strategic Power of Data : A Key Aspect of Sovereignty." 5(4).
-
-Trudgett, Skye, Kalinda Griffiths, Sara Farnbach, and Anthony Shakeshaft. 2022. "A Framework for Operationalising Aboriginal and Torres Strait Islander Data Sovereignty in Australia: Results of a Systematic Literature Review of Published Studies." eClinicalMedicine 45: 1--23.
-
-**IRBs/Tribal IRBs:**
-
-Around Him D, Aguilar TA, Frederick A, Larsen H, Seiber M, Angal J. Tribal IRBs: A Framework for Understanding Research Oversight in American Indian and Alaska Native Communities. Am Indian Alsk Native Ment Health Res. 2019;26(2):71-95. doi: 10.5820/aian.2602.2019.71. PMID: 31550379.
-
-Kuhn NS, Parker M, Lefthand-Begay C. Indigenous Research Ethics Requirements: An Examination of Six Tribal Institutional Review Board Applications and Processes in the United States. Journal of Empirical Research on Human Research Ethics. 2020;15(4):279-291.
-
-Marley TL. Indigenous Data Sovereignty: University Institutional Review Board Policies and Guidelines and Research with American Indian and Alaska Native Communities. American Behavioral Scientist. 2019;63(6):722-742.
-
-Marley TL. Indigenous Data Sovereignty: University Institutional Review Board Policies and Guidelines and Research with American Indian and Alaska Native Communities. American Behavioral Scientist. 2019;63(6):722-742.
-
-**Ethical research with Sami communities:**
-
-Eriksen, H., Rautio, A., Johnson, R. et al. Ethical considerations for community-based participatory research with Sami communities in North Finland. Ambio 50, 1222--1236 (2021).
-
-Jonsson, Å.N. Ethical guidelines for the documentation of árbediehtu, Sami traditional knowledge. In Working with Traditional Knowledge: Communities, Institutions, Information Systems, Law and Ethics. Writings from the Árbediehtu Pilot Project on Documentation and Protection of Sami Traditional Knowledge. Dieđut 1/2011. Sámi allaskuvla / Sámi University College 2011: 97--125.
-
-\
diff --git a/materials/sections/data-management-plans-reduced.Rmd b/materials/sections/data-management-plans-reduced.Rmd
deleted file mode 100644
index 5f1ef3d2..00000000
--- a/materials/sections/data-management-plans-reduced.Rmd
+++ /dev/null
@@ -1,135 +0,0 @@
-## Writing Good Data Management Plans
-
-### Learning Objectives
-
-In this lesson, you will learn:
-
-- Why create data management plans
-- The major components of data management plans
-- Tools that can help create a data management plan
-- Features and functionality of the DMPTool
-
-### When to Plan: The Data Life Cycle
-
-Shown below is one version of the [Data Life Cycle](https://www.dataone.org/data-life-cycle) that was developed by DataONE. The data life cycle provides a high level overview of the stages involved in successful management and preservation of data for use and reuse. Multiple versions of the data life cycle exist with differences attributable to variation in practices across domains or communities. It is not necessary for researchers to move through the data life cycle in a cyclical fashion and some research activities might use only part of the life cycle. For instance, a project involving meta-analysis might focus on the Discover, Integrate, and Analyze steps, while a project focused on primary data collection and analysis might bypass the Discover and Integrate steps. However, Plan is at the top of the data life cycle as it is advisable to initiate your data management planning at the beginning of your research process, before any data has been collected.
-
-
-
-### Why Plan?
-
-Planning data management in advance provides a number of benefits to the researcher.
-
-- **Saves time and increases efficiency**: Data management planning requires that a researcher think about data handling in advance of data collection, potentially raising any challenges before they occur.
-- **Engages your team**: Being able to plan effectively will require conversation with multiple parties, engaging project participants from the outset.
-- **Allows you to stay organized**: It will be easier to organize your data for analysis and reuse if you've made a plan about what analysis you want to run, future iterations, and more.
-- **Meet funder requirements**: Most funders require a data management plan (DMP) as part of the proposal process.
-- **Share data**: Information in the DMP is the foundation for archiving and sharing data with community.
-
-### How to Plan
-
-1) Make sure to **plan from the start** to avoid confusion, data loss, and increase efficiency. Given DMPs are a requirement of funding agencies, it is nearly always necessary to plan from the start. However, the same should apply to research that is being undertaken outside of a specific funded proposal.
-2) As indicated above, engaging your team is a benefit of data management planning. Collaborators involved in the data collection and processing of your research data bring diverse expertise. Therefore, **plan in collaboration** with these individuals.
-3) Make sure to **utilize resources** that are available to assist you in helping to write a good DMP. These might include your institutional library or organization data manager, online resources or education materials such as these.
-4) **Use tools** available to you; you don't have to reinvent the wheel.
-5) **Revise your plan** as situations change or as you potentially adapt/alter your project. Like your research projects, DMPs are not static, they require changes and updates throughout the research project process.
-
-### What to include in a DMP
-
-If you are writing a DMP as part of a solicitation proposal, the funding agency will have guidelines for the information they want to be provided in the plan. However, in general, a good plan will provide information on the:
-
-- study design
-
-- data to be collected
-
-- metadata
-
-- policies for access
-
-- sharing & reuse
-
-- long-term storage & data management
-
-- and budget
-
-*A note on Metadata:* Both basic metadata (such as title and researcher contact information) and comprehensive metadata (such as complete methods of data collection) are critical for accurate interpretation and understanding. The full definitions of variables, especially units, inside each dataset are also critical as they relate to the methods used for creation. Knowing certain blocking or grouping methods, for example, would be necessary to understand studies for proper comparisons and synthesis.
-
-### NSF DMP requirements
-
-In the 2014 Proposal Preparation Instructions, Section J ['Special Information and Supplementary Documentation'](https://www.nsf.gov/pubs/policydocs/pappguide/nsf14001/gpg_2.jsp#IIC2j) NSF put forward the baseline requirements for a DMP. In addition, there are specific division and program requirements that provide additional detail. If you are working on a research project with funding that does not require a DMP, or are developing a plan for unfunded research, the NSF generic requirements are a good set of guidelines to follow.
-
-The following questions are the prompting questions in the Arctic Data Center DMP template for NSF projects, excluding the fairly straightforward personnel section.
-
-**Five Sections of the NSF DMP Requirements**
-
-**1. What types of data, samples, collections, software, materials, etc. will be produced during your project?**\
-Types of data, samples, physical collections, software, curriculum materials, other materials produced during project
-
-**2. What format(s) will data and metadata be collected, processed, and stored in?**\
-Standards to be used for data and metadata format and content (for initial data collection, as well as subsequent storage and processing)
-
-**How will data be accessed and shared during the course of the project?**\
-Provisions for appropriate protection of privacy, confidentiality, security, intellectual property, or other rights or requirements
-
-**4. How do you anticipate the data for this project will be used?**\
-Including re-distribution and the production of derivatives
-
-**5. What is the long-term strategy for maintaining, curating, and archiving the data?**\
-Plans for archiving data, samples, research products and for preservation of access
-
-#### Individual Reflection
-
-Now that we've discussed the data life cycle, how to plan, what to generally include in a DMP, and the NSF DMP requirements - take five minutes to go through each required section for a NSF DMP and write down some initial thoughts on how you would approach completing those sections. What information would you include? How would you plan to answer the questions? What do you need to answer the questions in each section?
-
-After we'll get into groups to further discuss.
-
-#### Group Discussion
-
-Let's split up into five groups; one group for each required section of a NSF DMP. As a group, share your initial thoughts about the section you've been assigned to and together as a group discuss how you would complete that section. Select someone in the group to share your approach to the whole class. Take the next 10-15 minutes for group discussion.
-
-Some guiding questions:
-
-- What information do you need to complete the section? Think both broadly and detailed.
-
-- Do you need to reference outside materials to complete the section? Is this information already known / found or is additional research required?
-
-- What is the relevant, key information necessary for the research to be understood for either your future self or for someone new to the data? What information would you want to know if you were given a new project to work on? Being explicit and including details are important to think about for this question.
-
-- What workflows, documentation, standards, maintenance, tools / software, or roles are required?
-
-### Tools in Support of Creating a DMP
-
-
-
-The [DMPTool](https://dmptool.org) and [DMP Online](https://dmponline.dcc.ac.uk) are both easy to use web based tools that support the development of a DMP. The tools are partnered and share a code base; the DMPTool incorporates templates from US funding agencies and the DMP Online is focused on EU requirements.
-
-
-
-#### Quick Tips for DMPTool
-
-- There is no requirement to answer all questions in one sitting. Completing a DMP can require information gathering from multiple sources. Saving the plan at any point does not submit the plan, it simply saves your edits. This means you can move between sections in any order or save as you go.
-
-- You can collaborate in DMPTool which keeps all commentary together, saves time on collaboration, and makes it easy to access the most current version at any time since it is always available in DMPTool.
-
-### Arctic Data Center Support for DMPs
-
-To support researchers in creating DMPs that fulfills NSF template requirements and provides guidance on how to work with the Arctic Data Center for preservation, we have created an Arctic Data Center template within the DMPTool. This template walks researchers through the questions required by NSF and includes recommendations directly from the Arctic Data Center team.
-
-
-
-When creating a new plan, indicate that your funding agency is the National Science Foundation and you will then have the option to select a template. Here you can choose the Arctic Data Center.
-
-
-
-As you answer the questions posed, guidance information from the Arctic Data Center will be visible under the 'NSF' tab on the right hand side. An example answer is also provided at the bottom. It is not intended that you copy and paste this verbatim. Rather, this is example prose that you can refer to for answering the question.
-
-
-
-### Sharing Your DMP
-
-The DMPTool allows you to collaborate with authorized individuals on your plan and also to publish it (make it publicly accessible) via the website. If your research activity is funded, it is also useful to share your DMP with the Arctic Data Center. This is not an NSF requirement, but can greatly help the Arctic Data Center team prepare for your data submission. Especially if you are anticipating a high volume of data.
-
-### Additional Resources
-
-The article [Ten Simple Rules for Creating a Good Data Management Plan](https://journals.plos.org/ploscompbiol/article?id=10.1371/journal.pcbi.1004525) is a great resource for thinking about writing a data management plan and the information you should include within the plan.
-
-
diff --git a/materials/sections/data-modeling-socialsci.Rmd b/materials/sections/data-modeling-socialsci.Rmd
deleted file mode 100644
index c8f4adc7..00000000
--- a/materials/sections/data-modeling-socialsci.Rmd
+++ /dev/null
@@ -1,231 +0,0 @@
----
-author: "Jeanette Clark"
----
-
-
-### Learning Objectives
-
-- Understand basics of relational data models aka tidy data
-- Learn how to design and create effective data tables
-
-### Introduction
-
-In this lesson we are going to learn what relational data models are, and how they can be used to manage and analyze data efficiently. Relational data models are what relational databases use to organize tables. However, you don't have to be using a relational database (like mySQL, MariaDB, Oracle, or Microsoft Access) to enjoy the benefits of using a relational data model. Additionally, your data don't have to be large or complex for you to benefit. Here are a few of the benefits of using a relational data model:
-
-- Powerful search and filtering
-- Handle large, complex data sets
-- Enforce data integrity
-- Decrease errors from redundant updates
-
-#### Simple guidelines for data management {.unnumbered}
-
-A great paper called 'Some Simple Guidelines for Effective Data Management' [@borer_simple_2009] lays out exactly that - guidelines that make your data management, and your reproducible research, more effective.
-
-- **Use a scripted program (like R!)**
-
-A scripted program helps to make sure your work is reproducible. Typically, point-and-click actions, such as clicking on a cell in a spreadsheet program and modifying the value, are not reproducible or easily explained. Programming allows you to both reproduce what you did, and explain it if you use a tool like Rmarkdown.
-
-
-- **Non-proprietary file formats are preferred (eg: csv, txt)**
-
-Using a file that can be opened using free and open software greatly increases the longevity and accessibility of your data, since your data do not rely on having any particular software license to open the data file.
-
-- **Keep a raw version of data**
-
-In conjunction with using a scripted language, keeping a raw version of your data is definitely a requirement to generate a reproducible workflow. When you keep your raw data, your scripts can read from that raw data and create as many derived data products as you need, and you will always be able to re-run your scripts and know that you will get the same output.
-
-- **Use descriptive file and variable names (without spaces!)**
-
-When you use a scripted language, you will be using file and variable names as arguments to various functions. Programming languages are quite sensitive with what they are able to interpret as values, and they are particularly sensitive to spaces. So, if you are building reproducible workflows around scripting, or plan to in the future, saving your files without spaces or special characters will help you read those files and variables more easily. Additionally, making file and variables descriptive will help your future self and others more quickly understand what type of data they contain.
-
-- **Include a header line in your tabular data files**
-
-Using a single header line of column names as the first row of your data table is the most common and easiest way to achieve consistency among files.
-
-- **Use plain ASCII text**
-
-ASCII (sometimes just called plain text) is a very commonly used standard for character encoding, and is far more likely to persist very far into the future than proprietary binary formats such as Excel.
-
-The next three are a little more complex, but all are characteristics of the relational data model:
-
-- Design tables to add rows, not columns
-- Each column should contain only one type of information
-- Record a single piece of data only once; separate information collected at different scales into different tables.
-
-#### File and folder organization {-}
-
-Before moving on to discuss the last 3 rules, here is an example of how you might organize the files themselves following the simple rules above. Note that we have all open formats, plain text formats for data, sortable file names without special characters, scripts, and a special folder for raw files.
-
-
-
-### Recognizing untidy data
-
-Before we learn how to create a relational data model, let's look at how to recognize data that does not conform to the model.
-
-#### Data Organization {-}
-
-This is a screenshot of an actual dataset that came across NCEAS. We have all seen spreadsheets that look like this - and it is fairly obvious that whatever this is, it isn't very tidy. Let's dive deeper in to exactly **why** we wouldn't consider it tidy.
-
-
-
-#### Multiple tables {-}
-
-Your human brain can see from the way this sheet is laid out that it has three tables within it. Although it is easy for us to see and interpret this, it is extremely difficult to get a computer to see it this way, which will create headaches down the road should you try to read in this information to R or another programming language.
-
-
-
-#### Inconsistent observations {-}
-
-Rows correspond to **observations**. If you look across a single row, and you notice that there are clearly multiple observations in one row, the data are likely not tidy.
-
-
-
-#### Inconsistent variables {-}
-
-Columns correspond to **variables**. If you look down a column, and see that multiple variables exist in the table, the data are not tidy. A good test for this can be to see if you think the column consists of only one unit type.
-
-
-
-#### Marginal sums and statistics {-}
-
-Marginal sums and statistics also are not considered tidy, and they are not the same type of observation as the other rows. Instead, they are a combination of observations.
-
-
-### Good enough data modeling
-
-#### Denormalized data {-}
-
-When data are "denormalized" it means that observations about different entities are combined.
-
-
-
-In the above example, each row has measurements about both the community in which observations occurred, as well as observations of two individuals surveyed in that community. This is *not normalized* data.
-
-People often refer to this as *wide* format, because the observations are spread across a wide number of columns. Note that, should one survey another individual in either community, we would have to add new columns to the table. This is difficult to analyze, understand, and maintain.
-
-#### Tabular data {-}
-
-**Observations**. A better way to model data is to organize the observations about each type of entity in its own table. This results in:
-
-- Separate tables for each type of entity measured
-
-- Each row represents a single observation within that entity
-
-- Observations (rows) are all unique
-
-- This is *normalized* data (aka *tidy data*)
-
-**Variables**. In addition, for normalized data, we expect the variables to be organized such that:
-
-- All values in a column are of the same type
-- All columns pertain to the same observed entity (e.g., row)
-- Each column represents either an identifying variable or a measured variable
-
-#### Challenge {- .exercise}
-
-Try to answer the following questions:
-
-What are the observed entities in the example above?
-
-What are the measured variables associated with those observations?
-
-#### {-}
-
-
-Answer:
-
-
-
-
-If we use these questions to tidy our data, we should end up with:
-
-- one table for each entity observed
-- one column for each measured variable
-- additional columns for identifying variables (such as community)
-
-Here is what our tidy data look like:
-
-
-
-Note that this normalized version of the data meets the three guidelines set by [@borer_simple_2009]:
-
-- Design tables to add rows, not columns
-- Each column should contain only one type of information
-- Record a single piece of data only once; separate information collected at different scales into different tables.
-
-### Using normalized data
-
-Normalizing data by separating it into multiple tables often makes researchers really uncomfortable. This is understandable! The person who designed this study collected all of this information for a reason - so that they could analyze it together. Now that our community and survey information are in separate tables, how would we use population as a predictor variable for language spoken, for example? The answer is keys - and they are the cornerstone of relational data models.
-
-When one has normalized data, we often use unique identifiers to reference particular observations, which allows us to link across tables. Two types of identifiers are common within relational data:
-
-- Primary Key: unique identifier for each observed entity, one per row
-- Foreign Key: reference to a primary key in another table (linkage)
-
-
-#### Challenge {- .exercise}
-
-
-
-In our normalized tables above, identify the following:
-
-- the primary key for each table
-- any foreign keys that exist
-
-#### {-}
-
-**Answer**
-
-The primary key of the top table is `community`. The primary key of the bottom table is `id`.
-
-The `community` column is the *primary key* of that table because it uniquely identifies each row of the table as a unique observation of a community. In the second table, however, the `community` column is a *foreign key* that references the primary key from the first table.
-
-
-
-#### Entity-Relationship Model (ER) {-}
-
-An Entity-Relationship model allows us to compactly draw the structure of the tables in a relational database, including the primary and foreign keys in the tables.
-
-
-
-In the above model, one can see that each community in the community observations table must have one or more survey participants in the survey table, whereas each survey response has one and only one community.
-
-Here is a more complicated ER Model showing examples of other types of relationships.
-
-
-
-#### Merging data {-}
-
-Frequently, analysis of data will require merging these separately managed tables back together. There are multiple ways to join the observations in two tables, based on how the rows of one table are merged with the rows of the other.
-
-When conceptualizing merges, one can think of two tables, one on the *left* and one on the *right*. The most common (and often useful) join is when you merge the subset of rows that have matches in both the left table and the right table: this is called an *INNER JOIN*. Other types of join are possible as well. A *LEFT JOIN* takes all of the rows from the left table, and merges on the data from matching rows in the right table. Keys that don't match from the left table are still provided with a missing value (na) from the right table. A *RIGHT JOIN* is the same, except that all of the rows from the right table are included with matching data from the left, or a missing value. Finally, a *FULL OUTER JOIN* includes all data from all rows in both tables, and includes missing values wherever necessary.
-
-
-
-Sometimes people represent these as Venn diagrams showing which parts of the left and right tables are included in the results for each join. These however, miss part of the story related to where the missing value come from in each result.
-
-
-
-In the figure above, the blue regions show the set of rows that are included in the result. For the INNER join, the rows returned are all rows in A that have a matching row in B.
-
-### Data modeling exercise
-
-- Break into groups
-
-Our funding agency requires that we take surveys of individuals who complete our training courses so that we can report on the demographics of our trainees and how effective they find our courses to be. In your small groups, design a set of tables that will capture information collected in a participant survey that would apply to many courses.
-
-Don't focus on designing a comprehensive set of questions for the survey, one or two simple stand ins (eg: "Did the course meet your expectations?", "What could be improved?", "To what degree did your knowledge increase?") would be sufficient.
-
-Include as variables (columns) a basic set of information not only from the surveys, but about the courses, such as the date of the course and name of the course.
-
-
-Draw your entity-relationship model for your tables.
-
-
-### Resources
-
-- [Borer et al. 2009. **Some Simple Guidelines for Effective Data Management.** Bulletin of the Ecological Society of America.](http://matt.magisa.org/pubs/borer-esa-2009.pdf)
-- [White et al. 2013. **Nine simple ways to make it easier to (re)use your data.** Ideas in Ecology and Evolution 6.](https://doi.org/10.4033/iee.2013.6b.6.f)
-- [Software Carpentry SQL tutorial](https://swcarpentry.github.io/sql-novice-survey/)
-- [Tidy Data](http://vita.had.co.nz/papers/tidy-data.pdf)
-
diff --git a/materials/sections/exercise-intro-programming-socialsci.Rmd b/materials/sections/exercise-intro-programming-socialsci.Rmd
deleted file mode 100644
index 75a4058d..00000000
--- a/materials/sections/exercise-intro-programming-socialsci.Rmd
+++ /dev/null
@@ -1,33 +0,0 @@
-## R Practice I
-
-### Learning Objectives
-
-- practice base R skills
-- practice Markdown syntax
-- work in RMarkdown document
-
-### Introduction
-
-In this session of R practice, we will be working with the dataset: [Tobias Schwoerer, Kevin Berry, and Jorene Joe. 2022. A household survey documenting experiences with coastal hazards in a western Alaska community (2021-2022). Arctic Data Center. doi:10.18739/A29Z90D3V.](https://doi.org/10.18739/A29Z90D3V)
-
-This survey dataset has a few files, for this lesson we will be focusing on the initial survey results (Initial_Survey111721_ADC.csv). In the file, individual survey responses are oriented as rows, and the questions are oriented as columns. The column names are Q1, Q2, etc. Information about what the question was that was asked, and what the allowed values mean, are available in the metadata for each file. You can access the metadata for each file by clicking the "more info" link next to the file name at the top of the page.
-
-The goal for this session is to practice downloading data, reading it into R from an RMarkdown document, using base R commands to summarize a variable within the dataset, and practice formatting an RMardown docuement using Markdown syntax.
-
-### High level steps
-
-- navigate to the dataset and download the file `Initial_Survey111721_ADC.csv`
-- move the file to a `data` folder within your training project
-- create a new RMarkdown document, and structure your documents with relevant headers according to the steps below
-- read in the data
-- explore the data
- - try `summary()`, `colnames()`, `str()`, `unique()`, `View()`
-- calculate the mean of the answers to question 3
- - make sure to look at the help page (arguments section if your answer isn't what you expect)
- - interpret this value using the metadata for the table
-- write a conclusion based on your interpretation calling the mean value you calculated in text
-
-
-#### Bonus {.unnumbered}
-
-What other ways might you summarize the answers to question 3? Explore!
diff --git a/materials/sections/exercise-tidyverse-socialsci.Rmd b/materials/sections/exercise-tidyverse-socialsci.Rmd
deleted file mode 100644
index d67aa2e1..00000000
--- a/materials/sections/exercise-tidyverse-socialsci.Rmd
+++ /dev/null
@@ -1,25 +0,0 @@
-## R Practice II
-
-### Learning Objectives
-
-- practice tidyverse R skills
-
-### Introduction
-
-In this session of R practice, we will continue working with the dataset: [Tobias Schwoerer, Kevin Berry, and Jorene Joe. 2022. A household survey documenting experiences with coastal hazards in a western Alaska community (2021-2022). Arctic Data Center. doi:10.18739/A29Z90D3V.](https://doi.org/10.18739/A29Z90D3V)
-
-In this practice session, we will build upon the previous session by using `dplyr`, `tidyr`, and other packages form the tidyverse to create more summarizations of the survey answers.
-
-### High level steps
-
-- work in the same Rmd you did during R practice I
-- add necessary headers and text to describe what you are doing during this practice
-- using `group_by` and `summarize`, calculate how many responses there were to each unique answer for question 3
-- create a `data.frame` containing the definitions to the answer codes in question 3
- - use the metadata to get code-definition pairs
- - create your `data.frame` either by writing a new file and reading it in, or by exploring the function `tribble` (see the examples)
-- use a `left_join` to join your definitions table to the summarized answers
-
-#### Bonus {.unnumbered}
-
-Explore how you might summarize other questions in these survey results.
diff --git a/materials/sections/git-pull-requests-branches.Rmd b/materials/sections/git-pull-requests-branches.Rmd
deleted file mode 100644
index 55672b55..00000000
--- a/materials/sections/git-pull-requests-branches.Rmd
+++ /dev/null
@@ -1,140 +0,0 @@
-## Collaborating using Git
-
-
-### Learning Objectives
-
-In this lesson, you will learn:
-
-- New mechanisms to collaborate using __git__
-- What is a __Pull Request__ in Github?
-- How to contribute code to colleague's repository using Pull Requests
-- What is a __branch__ in git?
-- How to use a branch to organize code
-- What is a __tag__ in git and how is it useful for collaboration?
-
-### Pull requests
-
-We've shown in other chapters how to directly collaborate on a repository with
-colleagues by granting them `write` privileges as a collaborator to your repository.
-This is useful with close collaborators, but also grants them tremendous latitude to
-change files and analyses, to remove files from the working copy, and to modify all
-files in the repository.
-
-Pull requests represent a mechanism to more judiciously collaborate, one in which
-a collaborator can suggest changes to a repository, the owner and collaborator can
-discuss those changes in a structured way, and the owner can then review and accept
-all or only some of those changes to the repository. This is useful with open source
-code where a community is contributing to shared analytical software, to students in
-a lab working on related but not identical projects, and to others who want the
-capability to review changes as they are submitted.
-
-To use pull requests, the general procedure is as follows. The collaborator first
-creates a `fork` of the owner's repository, which is a cloned copy of the original
-that is linked to the original. This cloned copy is in the collaborator's GitHub
-account, which means they have the ability to make changes to it. But they don't have
-the right to change the original owner's copy. So instead, they `clone` their GitHub
-copy onto their local machine, which makes the collaborator's GitHub copy the `origin`
-as far as they are concerned. In this scenario, we generally refer to the Collaborator's
-repository as the remote `origin`, and the Owner's repository as `upstream`.
-
-
-
-Pull requests are a mechanism for someone that has a forked copy of a repository
-to **request** that the original owner review and pull in their changes. This
-allows them to collaborate, but keeps the owner in control of exactly what changed.
-
-### Exercise: Create and merge pull requests
-
-In this exercise, work in pairs. Each pair should create a `fork` of their partner's
-training repository, and then clone that onto their local machine. Then they can make changes
-to that forked repository, and, from the GitHub interface, create a pull request that the
-owner can incorporate. We'll walk through the process from both the owner and the collaborator's
-perspectives. In the following example, `mbjones` will be the repository owner, and `metamattj`
-will be the collaborator.
-
-1. *Change settings (Owner)*: Edit the github settings file for your `training-test` repository, and ensure that the collaborator does not have editing permission. Also, be sure that all changes in your repository are committed and pushed to the `origin` server.
-
-2. *Fork (Collaborator)*: Visit the GitHub page for the owner's GitHub repository on which you'd like
-to make changes, and click the `Fork` button. This will create a clone of that repository in your
-own GitHub account. You will be able to make changes to this forked copy of the repository, but
-you will not be able to make direct changes to the owner's copy. After you have forked the repository,
-visit your GitHub page for your forked repository, copy the url, and create a new RStudio project
-using that repository url.
-
-
-
-3. *Edit README.md (Collaborator)*: The collaborator should make one or more changes to the README.md
-file from their cloned copy of the repository, `commit` the changes, and `push` them to their forked copy. At this point, their local repo and github copy both have the changes that they made, but the owner's repository
-has not yet been changed. When you now visit your forked copy of the repository on Github, you will now see your change has been made, and it will say that `This branch is 1 commit ahead of mbjones:main.`
-
-
-
-4. *Create Pull Request (Collaborator)*: At this point, click the aptly named `Pull Request` button to create a pull request which will be used to ask that the *owner* pull in your changes to their copy.
-
-
-
-When you click `Create pull request`, provide a brief summary of the request, and a more detailed message to start a conversation about what you are requesting. It's helpful to be polite and concise while providing adequate context for your request. This will start a conversation with the owner in which you can discuss your changes, they can easily review the changes, and they can ask for further changes before the accept and pull them in. The owner of the repository is in control and determines if and when the changes are merged.
-
-
-
-5. *Review pull request (Owner)*: The owner will get an email notification that the Pull Request was created, and can see the PR listed in their `Pull requests` tab of their repsoitory.
-
-
-
-The owner can now initiate a conversation about the change, requesting further changes. The interface indicates whether there are any conflicts with the changes, and if not, gives the owner the option to `Merge pull request`.
-
-
-
-6. *Merge pull request (Owner)*: Once the owner thinks the changes look good, they can click the `Merge pull request` button to accept the changes and pull them into their repository copy. Edit the message, and then click `Confirm merge`.
-
-
-
-Congratulations, the PR request has now been merged into the owner's copy, and has been closed with a note indicating that the changes have been made.
-
-
-
-7. *Sync with owner (Collaborator)*: Now that the pull request has been merged, there is a
-new merge commit in the Owner's repository that is not present in either of the
-collaborator's repositories. To fix that,
-one needs to pull changes from the `upstream` repository into the collaborator's local
-repository, and then push those changes from that local repository to the collaborator's
-`origin` repository.
-
-To add a reference to the `upstream` remote (the repository you made your fork from), in the terminal, run:
-
-`git remote add upstream https://github.com/ORIGINAL_OWNER/ORIGINAL_REPOSITORY.git`
-
-Then to pull from the main branch of the `upstream` repository, in the terminal, run:
-
-`git pull upstream main`
-
-At this point, the collaborator is fully up to date.
-
-
-
-### Branches
-
-Branches are a mechanism to isolate a set of changes in their own thread, allowing multiple
-types of work to happen in parallel on a repository at the same time. These are most often
-used for trying out experimental work, or for managing bug fixes for historical releases
-of software. Here's an example graph showing a `branch2.1` that has changes in parallel
-to the main branch of development:
-
-
-
-The default branch in almost all repositories is called `main`, and it is the
-branch that is typically shown in the GitHub interface and elsewhere.
-There are many mechanisms to create branches. The one we will try is
-through RStudio, in which we use the branch dialog to create and switch
-between branches.
-
-#### Exercise:
-
-Create a new branch in your training repository called `exp-1`, and then make
-changes to the RMarkdown files in the directory. Commit and push those changes
-to the branch. Now you can switch between branches using the github interface.
-
-
-
-
-
diff --git a/materials/sections/inro-reproducibility.Rmd b/materials/sections/inro-reproducibility.Rmd
deleted file mode 100644
index 273a9b68..00000000
--- a/materials/sections/inro-reproducibility.Rmd
+++ /dev/null
@@ -1,78 +0,0 @@
-## Introduction to reproducible research
-
-Reproducibility is the hallmark of science, which is based on empirical observations
-coupled with explanatory models. And reproducible research is at the core of what we do at NCEAS, research synthesis.
-
-The National Center for Ecological Analysis and Synthesis was funded over 25 years ago to bring together interdisciplinary researchers in exploration of grand challenge ecological questions through analysis of existing data. Such questions often require integration, analysis and synthesis of diverse data across broad temporal, spatial and geographic scales. Data that is not typically collected by a single individual or collaborative team. Synthesis science, leveraging previously collected data, was a novel concept at that time and the approach and success of NCEAS has been a model for other synthesis centers.
-
-
-During this course you will learn about some of the challenges that can be encountered when working with published data, but more importantly, how to apply best practices to data collection, documentation, analysis and management to mitigate these challenges in support of reproducible research.
-
-#### Why is reproducible research important? {-}
-Working in a reproducible manner builds efficiencies into your own research practices. The ability to automate processes and rerun analyses as you collect more data, or share your full workflow (including data, code and products) with colleagues, will accelerate the pace of your research and collaborations. However, beyond these direct benefits, reproducible research builds trust in science with the public, policy makers and others.
-
-
-
-What data were used in this study? What methods applied? What were the parameter settings? What documentation or code are available to us to evaluate the results? Can we trust these data and methods?
-
-Are the results reproducible?
-
-
-
-Ionnidis (2005) contends that "Most research findings are false for most research designs and for most fields", and a study of replicability in psychology experiments found that "Most replication effects were smaller than the original results" (Open Science Collaboration, 2015).
-
-
-
-In the case of 'climategate', it took three years, and over 300 personnel, to gather the necessary provenance information in order to document how results, figures and other outputs were derived from input sources. Time and effort that could have been significantly reduced with appropriate documentation and reproducible practices. Moving forward, through reproducible research training, practices, and infrastructure, the need to manually chase this information will be reduced enabling replication studies and great trust in science.
-
-
-#### Computational reproducibility {-}
-
-While reproducibility encompasses the full science lifecycle, and includes issues such as methodological consistency and treatment of bias, in this course we will focus on **computational reproducibility**: the ability to document data, analyses, and models sufficiently for other researchers to be able to understand and ideally re-execute the computations that led to scientific results and conclusions.
-
-The first step towards addressing these issues is to be able to evaluate the data,
-analyses, and models on which conclusions are drawn. Under current practice,
-this can be difficult because data are typically unavailable, the method sections
-of papers do not detail the computational approaches used, and analyses and models
-are often conducted in graphical programs, or, when scripted analyses are employed,
-the code is not available.
-
-And yet, this is easily remedied. Researchers can achieve computational
-reproducibility through open science approaches, including straightforward steps
-for archiving data and code openly along with the scientific workflows describing
-the provenance of scientific results (e.g., @hampton_tao_2015, @munafo_manifesto_2017).
-
-
-#### Conceptualizing workflows {-}
-
-Scientific workflows encapsulate all of the steps from data acquisition, cleaning,
-transformation, integration, analysis, and visualization.
-
-
-
-Workflows can range in detail from simple flowcharts
-to fully executable scripts. R scripts and python scripts are a textual form
-of a workflow, and when researchers publish specific versions of the scripts and
-data used in an analysis, it becomes far easier to repeat their computations and
-understand the provenance of their conclusions.
-
-#### Summary
-
-Computational reproducibility provides:
-
-- transparency by capturing and communicating scientific workflows
-- research to stand on the shoulders of giants (build on work that came before)
-- credit for secondary usage and supports easy attribution
-- increased trust in science
-
-Preserving computational workflows enables understanding, evaluation, and reuse for the benefit of *future you* and your collaborators and colleagues **across disciplines**.
-
-Reproducibility means different things to different researchers. For our purposes, practical reproducibility looks like:
-
-- Preserving the data
-- Preserving the software workflow
-- Documenting what you did
-- Describing how to interpret it all
-
-During this course will outline best practices for how to make those four components happen.
-
diff --git a/materials/sections/logic-modeling.Rmd b/materials/sections/logic-modeling.Rmd
deleted file mode 100644
index aa9dc17b..00000000
--- a/materials/sections/logic-modeling.Rmd
+++ /dev/null
@@ -1,103 +0,0 @@
-## Logic Models
-
-In this session, we will:
-
-- Provide an overview of Logic Models
-- Apply the principles of Logic Models to synthesis development
-
-### Logic Models
-
-Logic models are a planning tool that are designed to support program development by depicting the flow of resources and processes leading to a desired result. They are also used for outcomes-based evaluation of a program and are often requested as part of an evaluation planning process by funders or stakeholders.
-
-A simplified logic models comprise three main parts: Inputs, Outputs and Outcomes.
-
-
-Inputs reflect ***what is invested***, outputs are ***what is done*** and outcomes are the ***results of the program***.
-
-In a more detailed logic model, outputs and outcomes are further broken down. Outputs are often represented as 'Activities' and 'Participants'. By including participation (or participants), the logic model is explicitly considering the intended audience, or stakeholders, impacted by the program. Engagement of this audience is an output. In the case of outcomes, these can be split into short, medium and long-term outcomes. Sometimes this last category may be labeled 'Impact'
-
-
-
-Defining the inputs, outputs and outcomes early in a planning process enables teams to visualize the workflow from activity to results and can help mitigate potential challenges. Logic models can be thought of as having an 'if this then that' structure where inputs -> outputs -> outcomes.
-
-
-
-In the example below we have constructed a simple logic model for a hypothetical project where training materials are being developed for a group of educators to implement at their respective institutions.
-
-
-Linkages are not always sequential and can be within categories, bi-directional and/or include feedback loops. Detailing this complexity of relationships, or theory of action, can be time consuming but is a valuable part of the thought process for project planning. In exploring all relationships, logic modeling also allows for assessing program feasibility.
-
-
-
-The above graphics include two sections within Outputs - Activities and Participants - and this is quite common. There is variation in logic model templates, including versions with a third type of output - "Products'. Sometimes description of these products is contained within the Activities section - for example, 'develop curricula', 'produce a report' - however calling these out explicitly is beneficial for teams focused on product development.
-
-Program development (and logic modeling) occurs in response to a given 'Situation' or need, and exploring this is the first step in modeling. The situation defines the objective, or problem, that the program is designed to solve hence some logic models may omit the left-hand situation column but be framed with Problem and Solution statements. Finally, comprehensive logic modeling takes into consideration assumptions that are made with respect to the resources available, the people involved, or the way the program will work and also recognizes that there are external factors that can impact the program's success.
-
-
-
-In summary:
-
-Logic models support program development and evaluation and comprise three primary steps in the workflow:
-
-- **Inputs:** Resources, contributions, and investments required for a program;
-- **Outputs:** Activities conducted, participants reached, and products produced; and
-- **Outcomes:** Results or expected changes arising from the program structured as short-, medium- and long-term.
-
-
-### Logic models for synthesis development
-
-Logic models are one tool for program development and have sufficient flexibility for a variety of situations, including planning a for a research collaboration. While some logic model categories may feel less relevant (can we scale up to a long-term outcome from a published synthesis?), the process of articulating the research objective, proposed outcome, associated resources and activities has value. Below are examples of questions that a typical logic model (LM) will ask, and how these might be reframed for a research collaboration (RC).
-
-**Objective/Problem Statement**
-
-LM: What is the problem? Why is this a problem? Who does this impact?
-
-RC: What is the current state of knowledge? What gaps exists in understanding? Why is more information / synthesis important?
-
-**Inputs**
-
-LM: What resources are needed for the program? Personnel, money, time, equipment, partnerships ..
-
-RC: What is needed to undertake the synthesis research? For personnel, think in terms of the roles that are needed - data manager, statistician, writer, editor etc. Consider the time frame. DATA - what data are needed and what already exists?
-
-**Outputs - Activities**
-
-LM: What will be done? Development, design, workshops, conferences, counseling, outreach..
-
-RC: What activities are needed to conduct the research? This could be high level or it could be broken down into details such as the types of statistical approaches.
-
-**Outputs - Participants**
-
-LM: Who will we reach? Clients, Participants, Customers..
-
-RC: Who is the target audience? Who will be impacted by this work? Who is positioned to leverage this work?
-
-**Outputs - Products**
-
-LM: What will you create? Publications, websites, media communications ...
-
-RC: What research products are planned / expected? Consider this in relation to the intended audience. Is a peer-reviewed publication, report or white paper most appropriate? How will derived data be handled? Will documentation, workflows or code be published?
-
-**Short-term Outcomes**
-
-LM: What short-term outcomes are anticipated among participants. These can include changes in awareness, knowledge, skills, attitudes, opinions and intent.
-
-RC: Will this work represent a significant contribution to current understanding?
-
-**Medium-term Outcomes**
-
-LM: What medium-term outcomes are predicted among participants? These might include changes in behaviors, decision-making and actions.
-
-RC: Will this work promote increased research activity or open new avenues of inquiry?
-
-**Long-term Outcomes**
-
-LM: What long-term benefits, or impacts, are expected? Changes in social, economic, civic, and environmental conditions?
-
-RC: Will this work result in local, regional or national policy change? What will be the long-term impact of increased investment in the ecosystem?
-
-### Resources
-
-- [Logic model template](https://deltacouncil.sharepoint.com/:p:/r/sites/Extranet-Science/_layouts/15/Doc.aspx?sourcedoc=%7BB1F16AA0-0938-4623-AD23-5E50BF376B68%7D&file=logic_model_template.pptx&action=edit&mobileredirect=true) (ppt) on Sharepoint
-
-
diff --git a/materials/sections/metadata-adc-data-documentation-hands-on.Rmd b/materials/sections/metadata-adc-data-documentation-hands-on.Rmd
deleted file mode 100644
index 30d61309..00000000
--- a/materials/sections/metadata-adc-data-documentation-hands-on.Rmd
+++ /dev/null
@@ -1,354 +0,0 @@
-## Data Documentation and Publishing
-
-### Learning Objectives
-
-In this lesson, you will learn:
-
-- About open data archives, especially the Arctic Data Center
-- What science metadata are and how they can be used
-- How data and code can be documented and published in open data archives
- - Web-based submission
-
-### Data sharing and preservation
-
-
-
-
-
-### Data repositories: built for data (and code)
-
-- GitHub is not an archival location
-- Examples of dedicated data repositories: KNB, Arctic Data Center, tDAR, EDI, Zenodo
- + Rich metadata
- + Archival in their mission
- + Certification for repositories: https://www.coretrustseal.org/
-- Data papers, e.g., Scientific Data
-- List of data repositories: http://re3data.org
- + Repository finder tool: https://repositoryfinder.datacite.org/
-
-
-
-### Metadata
-
-Metadata are documentation describing the content, context, and structure of
-data to enable future interpretation and reuse of the data. Generally, metadata
-describe who collected the data, what data were collected, when and where they were
-collected, and why they were collected.
-
-For consistency, metadata are typically structured following metadata content
-standards such as the [Ecological Metadata Language (EML)](https://knb.ecoinformatics.org/software/eml/).
-For example, here's an excerpt of the metadata for a sockeye salmon dataset:
-
-```xml
-
-
-
- Improving Preseason Forecasts of Sockeye Salmon Runs through
- Salmon Smolt Monitoring in Kenai River, Alaska: 2005 - 2007
-
-
- Mark
- Willette
-
- Alaska Department of Fish and Game
- Fishery Biologist
-
- Soldotna
- Alaska
- USA
-
- (907)260-2911
- mark.willette@alaska.gov
-
- ...
-
-
-```
-
-That same metadata document can be converted to HTML format and displayed in a much
-more readable form on the web: https://knb.ecoinformatics.org/#view/doi:10.5063/F1F18WN4
-
-
-And, as you can see, the whole dataset or its components can be downloaded and
-reused.
-
-Also note that the repository tracks how many times each file has been downloaded,
-which gives great feedback to researchers on the activity for their published data.
-
-### Structure of a data package
-
-Note that the dataset above lists a collection of files that are contained within
-the dataset. We define a *data package* as a scientifically useful collection of
-data and metadata that a researcher wants to preserve. Sometimes a data package
-represents all of the data from a particular experiment, while at other times it
-might be all of the data from a grant, or on a topic, or associated with a paper.
-Whatever the extent, we define a data package as having one or more data files,
-software files, and other scientific products such as graphs and images, all tied
-together with a descriptive metadata document.
-
-
-
-These data repositories all assign a unique identifier to every version of every
-data file, similarly to how it works with source code commits in GitHub. Those identifiers
-usually take one of two forms. A *DOI* identifier is often assigned to the metadata
-and becomes the publicly citable identifier for the package. Each of the other files
-gets a global identifier, often a UUID that is globally unique. In the example above,
-the package can be cited with the DOI `doi:10.5063/F1F18WN4`,and each of the individual
-files have their own identifiers as well.
-
-### DataONE Federation
-
-DataONE is a federation of dozens of data repositories that work together to make their
-systems interoperable and to provide a single unified search system that spans
-the repositories. DataONE aims to make it simpler for researchers to publish
-data to one of its member repositories, and then to discover and download that
-data for reuse in synthetic analyses.
-
-DataONE can be searched on the web (https://search.dataone.org/), which effectively
-allows a single search to find data from the dozens of members of DataONE, rather
-than visiting each of the (currently 44!) repositories one at a time.
-
-
-
-
-### Publishing data from the web
-
-Each data repository tends to have its own mechanism for submitting data and
-providing metadata. With repositories like the KNB Data Repository and the
-Arctic Data Center, we provide some easy to use web forms for editing and submitting
-a data package. This section provides a brief overview of some highlights within the data submission process, in advance of a more comprehensive hands-on activity.
-
-**ORCiDs**
-
-We will walk through web submission on https://demo.arcticdata.io, and start by logging in with an ORCID account. ORCID provides a common account for sharing scholarly data, so if you don’t have one, you can create one when you are redirected to ORCID from the Sign In button.
-
-
-
-
-
-ORCID is a non-profit organization made up of research institutions, funders, publishers and other stakeholders in the research space. ORCID stands for Open Researcher and Contributor ID. The purpose of ORCID is to give researchers a unique identifier which then helps highlight and give credit to researchers for their work. If you click on someone’s ORCID, their work and research contributions will show up (as long as the researcher used ORCID to publish or post their work).
-
-After signing in, you can access the data submission form using the Submit button. Once on the form, upload your data files and follow the prompts to provide the required metadata.
-
-
-
-**Sensitive Data Handling**
-
-Underneath the Title field, you will see a section titled “Data Sensitivity”. As the primary repository for the NSF Office of Polar Programs Arctic Section, the Arctic Data Center accepts data from all disciplines. This includes data from social science research that may include sensitive data, meaning data that contains personal or identifiable information. Sharing sensitive data can pose challenges to researchers, however sharing metadata or anonymized data contributes to discovery, supports open science principles, and helps reduce duplicate research efforts.
-
-To help mitigate the challenges of sharing sensitive data, the Arctic Data Center has added new features to the data submission process influenced by the CARE Principles for Indigenous Data Governance (Collective benefit, Authority to control, Responsibility, Ethics). Researchers submitting data now have the option to choose between varying levels of sensitivity that best represent their dataset. Data submitters can select one of three sensitivity level data tags that best fit their data and/or metadata. Based on the level of sensitivity, guidelines for submission are provided. The data tags range from non-confidential information to maximally sensitive information.
-
-The purpose of these tags is to ethically contribute to open science by making the richest set of data available for future research. The first tag, “non-sensitive data”, represents data that does not contain potentially harmful information, and can be submitted without further precaution. Data or metadata that is “sensitive with minimal risk” means that either the sensitive data has been anonymized and shared with consent, or that publishing it will not cause any harm. The third option, “some or all data is sensitive with significant risk” represents data that contains potentially harmful or identifiable information, and the data submitter will be asked to hold off submitting the data until further notice. In the case where sharing anonymized sensitive data is not possible due to ethical considerations, sharing anonymized metadata still aligns with FAIR (Findable, Accessible, Interoperable, Reproducible) principles because it increases the visibility of the research which helps reduce duplicate research efforts. Hence, it is important to share metadata, and to publish or share sensitive data only when consent from participants is given, in alignment with the CARE principles and any IRB requirements.
-
-You will continue to be prompted to enter information about your research, and in doing so, create your metadata record. We recommend taking your time because the richer your metadata is, the more easily reproducible and usable your data and research will be for both your future self and other researchers. Detailed instructions are provided below for the hands-on activity.
-
-**Research Methods**
-
-Methods are critical to accurate interpretation and reuse of your data. The editor allows you to add multiple different methods sections, so that you can include details of sampling methods, experimental design, quality assurance procedures, and/or computational techniques and software.
-
-
-
-As part of a recent update, researchers are now asked to describe the ethical data practices used throughout their research. The information provided will be visible as part of the metadata record. This feature was added to the data submission process to encourage transparency in data ethics. Transparency in data ethics is a vital part of open science and sharing ethical practices encourages deeper discussion about data reuse and ethics.
-
-We encourage you to think about the ethical data and research practices that were utilized during your research, even if they don’t seem obvious at first.
-
-**File and Variable Level Metadata**
-
-In addition to providing information about, (or a description of) your dataset, you can also provide information about each file and the variables within the file. By clicking the "Describe" button you can add comprehensive information about each of your measurements, such as the name, measurement type, standard units etc.
-
-
-
-**Provenance**
-
-The data submission system also provides the opportunity for you to provide provenance information, describe the relationship between package elements. When viewing your dataset followinng submission, After completing your data description and submitting your dataset you will see the option to add source data and code, and derived data and code.
-
-
-
-These are just some of the features and functionality of the Arctic Data Center submission system and we will go through them in more detail below as part of a hands-on activity.
-
-
-#### Download the data to be used for the tutorial
-
-I've already uploaded the test data package, and so you can access the data here:
-
-- https://demo.arcticdata.io/#view/urn:uuid:0702cc63-4483-4af4-a218-531ccc59069f
-
-Grab both CSV files, and the R script, and store them in a convenient folder.
-
-
-
-#### Login via ORCID
-
-We will walk through web submission on https://demo.arcticdata.io, and start
-by logging in with an ORCID account. [ORCID](https://orcid.org/) provides a common account for sharing
-scholarly data, so if you don't have one, you can create one when you are redirected
-to ORCID from the *Sign In* button.
-
-
-When you sign in, you will be redirected to [orcid.org](https://orcid.org), where you can either provide your existing ORCID credentials or create a new account. ORCID provides multiple ways to login, including using your email address, an institutional login from many universities, and/or a login from social media account providers. Choose the one that is best suited to your use as a scholarly record, such as your university or agency login.
-
-
-
-#### Create and submit the dataset
-
-After signing in, you can access the data submission form using the *Submit* button.
-Once on the form, upload your data files and follow the prompts to provide the
-required metadata.
-
-##### Click **Add Files** to choose the data files for your package
-
-You can select multiple files at a time to efficiently upload many files.
-
-
-
-The files will upload showing a progress indicator. You can continue editing
-metadata while they upload.
-
-
-
-##### Enter Overview information
-
-This includes a descriptive title, abstract, and keywords.
-
-
-
-You also must enter a funding award number and choose a license. The funding field will
-search for an NSF award identifier based on words in its title or the number itself. The licensing options are CC-0 and CC-BY, which both allow your data to be downloaded and re-used by other researchers.
-
-- CC-0 Public Domain Dedication: “...can copy, modify, distribute and perform the work, even for commercial purposes, all without asking permission.”
-- CC-BY: Attribution 4.0 International License: “...free to...copy,...redistribute,...remix, transform, and build upon the material for any purpose, even commercially,...[but] must give appropriate credit, provide a link to the license, and indicate if changes were made.”
-
-
-
-
-##### People Information
-
-Information about the people associated with the dataset is essential to provide
-credit through citation and to help people understand who made contributions to
-the product. Enter information for the following people:
-
-- Creators - **all the people who should be in the citation for the dataset**
-- Contacts - one is required, but defaults to the first Creator if omitted
-- Principal Investigators
-- Any others that are relevant
-
-For each, please provide their [ORCID](https://orcid.org) identifier, which
-helps link this dataset to their other scholarly works.
-
-
-
-##### Location Information
-
-The geospatial location that the data were collected is critical for discovery
-and interpretation of the data. Coordinates are entered in decimal degrees, and
-be sure to use negative values for West longitudes. The editor allows you to
-enter multiple locations, which you should do if you had noncontiguous sampling
-locations. This is particularly important if your sites are separated by large
-distances, so that spatial search will be more precise.
-
-
-
-Note that, if you miss fields that are required, they will be highlighted in red
-to draw your attention. In this case, for the description, provide a comma-separated
-place name, ordered from the local to global:
-
-- Mission Canyon, Santa Barbara, California, USA
-
-
-
-##### Temporal Information
-
-Add the temporal coverage of the data, which represents the time period to which
-data apply. Again, use multiple date ranges if your sampling was discontinuous.
-
-
-
-##### Methods
-
-Methods are critical to accurate interpretation and reuse of your data. The editor
-allows you to add multiple different methods sections, so that you can include details of
-sampling methods, experimental design, quality assurance procedures, and/or computational
-techniques and software. Please be complete with your methods sections, as they
-are fundamentally important to reuse of the data.
-
-
-
-##### Save a first version with **Submit**
-
-When finished, click the *Submit Dataset* button at the bottom.
-
-If there are errors or missing fields, they will be highlighted.
-
-Correct those, and then try submitting again. When you are successful, you should
-see a large green banner with a link to the current dataset view. Click the `X`
-to close that banner if you want to continue editing metadata.
-
-
-Success!
-
-#### File and variable level metadata
-
-The final major section of metadata concerns the structure and content
-of your data files. In this case, provide the names and descriptions of
-the data contained in each file, as well as details of their internal structure.
-
-For example, for data tables, you'll need the name, label, and definition of
-each variable in your file. Click the **Describe** button to access a dialog to enter this information.
-
-
-The **Attributes** tab is where you enter variable (aka attribute)
-information, including:
-
-- variable name (for programs)
-- variable label (for display)
-
-
-- variable definition (be specific)
-- type of measurement
-
-- units & code definitions
-
-
-You'll need to add these definitions for every variable (column) in
-the file. When done, click **Done**.
-
-Now, the list of data files will show a green checkbox indicating that you have
-fully described that file's internal structure. Proceed with the other CSV
-files, and then click **Submit Dataset** to save all of these changes.
-
-
-After you get the big green success message, you can visit your
-dataset and review all of the information that you provided. If
-you find any errors, simply click **Edit** again to make changes.
-
-#### Add workflow provenance
-
-Understanding the relationships between files (aka *provenance*) in a package is critically important,
-especially as the number of files grows. Raw data are transformed and integrated
-to produce derived data, which are often then used in analysis and visualization code
-to produce final outputs. In the DataONE network, we support structured descriptions of these
-relationships, so researchers can see the flow of data from raw data to derived to outputs.
-
-You add provenance by navigating to the data table descriptions and selecting the
-`Add` buttons to link the data and scripts that were used in your computational
-workflow. On the left side, select the `Add` circle to add an **input** data source
-to the filteredSpecies.csv file. This starts building the provenance graph to
-explain the origin and history of each data object.
-
-
-The linkage to the source dataset should appear.
-
-
-
-Then you can add the link to the source code that handled the conversion
-between the data files by clicking on `Add` arrow and selecting the R script:
-
-
-
-
-The diagram now shows the relationships among the data files and the R script, so
-click **Submit** to save another version of the package.
-
-
-Et voilà! A beautifully preserved data package!
diff --git a/materials/sections/metadata-adc-data-documentation-socialsci.Rmd b/materials/sections/metadata-adc-data-documentation-socialsci.Rmd
deleted file mode 100644
index ad80d2fc..00000000
--- a/materials/sections/metadata-adc-data-documentation-socialsci.Rmd
+++ /dev/null
@@ -1,351 +0,0 @@
-## Data Documentation and Publishing
-
-### Learning Objectives
-
-In this lesson, you will learn:
-
-- About open data archives, especially the Arctic Data Center
-- What science metadata are and how they can be used
-- How data and code can be documented and published in open data archives
- - Web-based submission
-
-### Data sharing and preservation
-
-
-
-
-
-### Data repositories: built for data (and code)
-
-- GitHub is not an archival location
-- Examples of dedicated data repositories: KNB, Arctic Data Center, tDAR, EDI, Zenodo
- + Rich metadata
- + Archival in their mission
- + Certification for repositories: https://www.coretrustseal.org/
-- Data papers, e.g., Scientific Data
-- List of data repositories: http://re3data.org
- + Repository finder tool: https://repositoryfinder.datacite.org/
-
-
-
-### Metadata
-
-Metadata are documentation describing the content, context, and structure of
-data to enable future interpretation and reuse of the data. Generally, metadata
-describe who collected the data, what data were collected, when and where they were
-collected, and why they were collected.
-
-For consistency, metadata are typically structured following metadata content
-standards such as the [Ecological Metadata Language (EML)](https://knb.ecoinformatics.org/software/eml/).
-For example, here's an excerpt of the metadata for a sockeye salmon dataset:
-
-```xml
-
-
-
- Improving Preseason Forecasts of Sockeye Salmon Runs through
- Salmon Smolt Monitoring in Kenai River, Alaska: 2005 - 2007
-
-
- Mark
- Willette
-
- Alaska Department of Fish and Game
- Fishery Biologist
-
- Soldotna
- Alaska
- USA
-
- (907)260-2911
- mark.willette@alaska.gov
-
- ...
-
-
-```
-
-That same metadata document can be converted to HTML format and displayed in a much
-more readable form on the web: https://knb.ecoinformatics.org/#view/doi:10.5063/F1F18WN4
-
-
-And, as you can see, the whole dataset or its components can be downloaded and
-reused.
-
-Also note that the repository tracks how many times each file has been downloaded,
-which gives great feedback to researchers on the activity for their published data.
-
-### Structure of a data package
-
-Note that the dataset above lists a collection of files that are contained within
-the dataset. We define a *data package* as a scientifically useful collection of
-data and metadata that a researcher wants to preserve. Sometimes a data package
-represents all of the data from a particular experiment, while at other times it
-might be all of the data from a grant, or on a topic, or associated with a paper.
-Whatever the extent, we define a data package as having one or more data files,
-software files, and other scientific products such as graphs and images, all tied
-together with a descriptive metadata document.
-
-
-
-These data repositories all assign a unique identifier to every version of every
-data file, similarly to how it works with source code commits in GitHub. Those identifiers
-usually take one of two forms. A *DOI* identifier is often assigned to the metadata
-and becomes the publicly citable identifier for the package. Each of the other files
-gets a global identifier, often a UUID that is globally unique. In the example above,
-the package can be cited with the DOI `doi:10.5063/F1F18WN4`,and each of the individual
-files have their own identifiers as well.
-
-### DataONE Federation
-
-DataONE is a federation of dozens of data repositories that work together to make their
-systems interoperable and to provide a single unified search system that spans
-the repositories. DataONE aims to make it simpler for researchers to publish
-data to one of its member repositories, and then to discover and download that
-data for reuse in synthetic analyses.
-
-DataONE can be searched on the web (https://search.dataone.org/), which effectively
-allows a single search to find data from the dozens of members of DataONE, rather
-than visiting each of the (currently 44!) repositories one at a time.
-
-
-
-
-### Publishing data from the web
-
-Each data repository tends to have its own mechanism for submitting data and
-providing metadata. With repositories like the KNB Data Repository and the
-Arctic Data Center, we provide some easy to use web forms for editing and submitting
-a data package. This section provides a brief overview of some highlights within the data submission process, in advance of a more comprehensive hands-on activity.
-
-**ORCiDs**
-
-We will walk through web submission on https://demo.arcticdata.io, and start by logging in with an ORCID account. ORCID provides a common account for sharing scholarly data, so if you don’t have one, you can create one when you are redirected to ORCID from the Sign In button.
-
-
-
-
-
-ORCID is a non-profit organization made up of research institutions, funders, publishers and other stakeholders in the research space. ORCID stands for Open Researcher and Contributor ID. The purpose of ORCID is to give researchers a unique identifier which then helps highlight and give credit to researchers for their work. If you click on someone’s ORCID, their work and research contributions will show up (as long as the researcher used ORCID to publish or post their work).
-
-After signing in, you can access the data submission form using the Submit button. Once on the form, upload your data files and follow the prompts to provide the required metadata.
-
-
-
-**Sensitive Data Handling**
-
-Underneath the Title field, you will see a section titled “Data Sensitivity”. As the primary repository for the NSF Office of Polar Programs Arctic Section, the Arctic Data Center accepts data from all disciplines. This includes data from social science research that may include sensitive data, meaning data that contains personal or identifiable information. Sharing sensitive data can pose challenges to researchers, however sharing metadata or anonymized data contributes to discovery, supports open science principles, and helps reduce duplicate research efforts.
-
-To help mitigate the challenges of sharing sensitive data, the Arctic Data Center has added new features to the data submission process influenced by the CARE Principles for Indigenous Data Governance (Collective benefit, Authority to control, Responsibility, Ethics). Researchers submitting data now have the option to choose between varying levels of sensitivity that best represent their dataset. Data submitters can select one of three sensitivity level data tags that best fit their data and/or metadata. Based on the level of sensitivity, guidelines for submission are provided. The data tags range from non-confidential information to maximally sensitive information.
-
-The purpose of these tags is to ethically contribute to open science by making the richest set of data available for future research. The first tag, “non-sensitive data”, represents data that does not contain potentially harmful information, and can be submitted without further precaution. Data or metadata that is “sensitive with minimal risk” means that either the sensitive data has been anonymized and shared with consent, or that publishing it will not cause any harm. The third option, “some or all data is sensitive with significant risk” represents data that contains potentially harmful or identifiable information, and the data submitter will be asked to hold off submitting the data until further notice. In the case where sharing anonymized sensitive data is not possible due to ethical considerations, sharing anonymized metadata still aligns with FAIR (Findable, Accessible, Interoperable, Reproducible) principles because it increases the visibility of the research which helps reduce duplicate research efforts. Hence, it is important to share metadata, and to publish or share sensitive data only when consent from participants is given, in alignment with the CARE principles and any IRB requirements.
-
-You will continue to be prompted to enter information about your research, and in doing so, create your metadata record. We recommend taking your time because the richer your metadata is, the more easily reproducible and usable your data and research will be for both your future self and other researchers. Detailed instructions are provided below for the hands-on activity.
-
-**Research Methods**
-
-Methods are critical to accurate interpretation and reuse of your data. The editor allows you to add multiple different methods sections, so that you can include details of sampling methods, experimental design, quality assurance procedures, and/or computational techniques and software.
-
-
-
-As part of a recent update, researchers are now asked to describe the ethical data practices used throughout their research. The information provided will be visible as part of the metadata record. This feature was added to the data submission process to encourage transparency in data ethics. Transparency in data ethics is a vital part of open science and sharing ethical practices encourages deeper discussion about data reuse and ethics.
-
-We encourage you to think about the ethical data and research practices that were utilized during your research, even if they don’t seem obvious at first.
-
-**File and Variable Level Metadata**
-
-In addition to providing information about, (or a description of) your dataset, you can also provide information about each file and the variables within the file. By clicking the "Describe" button you can add comprehensive information about each of your measurements, such as the name, measurement type, standard units etc.
-
-
-
-**Provenance**
-
-The data submission system also provides the opportunity for you to provide provenance information, describe the relationship between package elements. When viewing your dataset followinng submission, After completing your data description and submitting your dataset you will see the option to add source data and code, and derived data and code.
-
-
-
-These are just some of the features and functionality of the Arctic Data Center submission system and we will go through them in more detail below as part of a hands-on activity.
-
-
-#### Download the data to be used for the tutorial
-
-I've already uploaded the test data package, and so you can access the data here:
-
-- https://demo.arcticdata.io/view/urn%3Auuid%3A98c799ef-d7c9-4658-b432-4d486221fca3
-
-Grab both CSV files, and the R script, and store them in a convenient folder.
-
-
-#### Login via ORCID
-
-We will walk through web submission on https://demo.arcticdata.io, and start
-by logging in with an ORCID account. [ORCID](https://orcid.org/) provides a common account for sharing
-scholarly data, so if you don't have one, you can create one when you are redirected
-to ORCID from the *Sign In* button.
-
-
-When you sign in, you will be redirected to [orcid.org](https://orcid.org), where you can either provide your existing ORCID credentials or create a new account. ORCID provides multiple ways to login, including using your email address, an institutional login from many universities, and/or a login from social media account providers. Choose the one that is best suited to your use as a scholarly record, such as your university or agency login.
-
-
-
-#### Create and submit the dataset
-
-After signing in, you can access the data submission form using the *Submit* button.
-Once on the form, upload your data files and follow the prompts to provide the
-required metadata.
-
-##### Click **Add Files** to choose the data files for your package
-
-You can select multiple files at a time to efficiently upload many files.
-
-
-
-The files will upload showing a progress indicator. You can continue editing
-metadata while they upload.
-
-
-
-##### Enter Overview information
-
-This includes a descriptive title, abstract, and keywords.
-
-
-
-You also must enter a funding award number and choose a license. The funding field will
-search for an NSF award identifier based on words in its title or the number itself. The licensing options are CC-0 and CC-BY, which both allow your data to be downloaded and re-used by other researchers.
-
-- CC-0 Public Domain Dedication: “...can copy, modify, distribute and perform the work, even for commercial purposes, all without asking permission.”
-- CC-BY: Attribution 4.0 International License: “...free to...copy,...redistribute,...remix, transform, and build upon the material for any purpose, even commercially,...[but] must give appropriate credit, provide a link to the license, and indicate if changes were made.”
-
-
-
-##### People Information
-
-Information about the people associated with the dataset is essential to provide
-credit through citation and to help people understand who made contributions to
-the product. Enter information for the following people:
-
-- Creators - **all the people who should be in the citation for the dataset**
-- Contacts - one is required, but defaults to the first Creator if omitted
-- Principal Investigators
-- Any others that are relevant
-
-For each, please provide their [ORCID](https://orcid.org) identifier, which
-helps link this dataset to their other scholarly works.
-
-
-
-##### Location Information
-
-The geospatial location that the data were collected is critical for discovery
-and interpretation of the data. Coordinates are entered in decimal degrees, and
-be sure to use negative values for West longitudes. The editor allows you to
-enter multiple locations, which you should do if you had noncontiguous sampling
-locations. This is particularly important if your sites are separated by large
-distances, so that spatial search will be more precise.
-
-
-
-Note that, if you miss fields that are required, they will be highlighted in red
-to draw your attention. In this case, for the description, provide a comma-separated
-place name, ordered from the local to global:
-
-- Mission Canyon, Santa Barbara, California, USA
-
-
-
-##### Temporal Information
-
-Add the temporal coverage of the data, which represents the time period to which
-data apply. Again, use multiple date ranges if your sampling was discontinuous.
-
-
-
-##### Methods
-
-Methods are critical to accurate interpretation and reuse of your data. The editor
-allows you to add multiple different methods sections, so that you can include details of
-sampling methods, experimental design, quality assurance procedures, and/or computational
-techniques and software. Please be complete with your methods sections, as they
-are fundamentally important to reuse of the data.
-
-
-
-##### Save a first version with **Submit**
-
-When finished, click the *Submit Dataset* button at the bottom.
-
-If there are errors or missing fields, they will be highlighted.
-
-Correct those, and then try submitting again. When you are successful, you should
-see a large green banner with a link to the current dataset view. Click the `X`
-to close that banner if you want to continue editing metadata.
-
-
-Success!
-
-#### File and variable level metadata
-
-The final major section of metadata concerns the structure and content
-of your data files. In this case, provide the names and descriptions of
-the data contained in each file, as well as details of their internal structure.
-
-For example, for data tables, you'll need the name, label, and definition of
-each variable in your file. Click the **Describe** button to access a dialog to enter this information.
-
-
-The **Attributes** tab is where you enter variable (aka attribute)
-information, including:
-
-- variable name (for programs)
-- variable label (for display)
-
-
-- variable definition (be specific)
-- type of measurement
-
-- units & code definitions
-
-
-You'll need to add these definitions for every variable (column) in
-the file. When done, click **Done**.
-Now, the list of data files will show a green checkbox indicating that you have
-fully described that file's internal structure. Proceed with the other CSV
-files, and then click **Submit Dataset** to save all of these changes.
-
-
-After you get the big green success message, you can visit your
-dataset and review all of the information that you provided. If
-you find any errors, simply click **Edit** again to make changes.
-
-#### Add workflow provenance
-
-Understanding the relationships between files (aka *provenance*) in a package is critically important,
-especially as the number of files grows. Raw data are transformed and integrated
-to produce derived data, which are often then used in analysis and visualization code
-to produce final outputs. In the DataONE network, we support structured descriptions of these
-relationships, so researchers can see the flow of data from raw data to derived to outputs.
-
-You add provenance by navigating to the data table descriptions and selecting the
-`Add` buttons to link the data and scripts that were used in your computational
-workflow. On the left side, select the `Add` circle to add an **input** data source
-to the filteredSpecies.csv file. This starts building the provenance graph to
-explain the origin and history of each data object.
-
-
-The linkage to the source dataset should appear.
-
-
-
-Then you can add the link to the source code that handled the conversion
-between the data files by clicking on `Add` arrow and selecting the R script:
-
-
-
-
-The diagram now shows the relationships among the data files and the R script, so
-click **Submit** to save another version of the package.
-
-
-Et voilà! A beautifully preserved data package!
diff --git a/materials/sections/parallel-computing-in-r.Rmd b/materials/sections/parallel-computing-in-r.Rmd
deleted file mode 100644
index 7a6052a6..00000000
--- a/materials/sections/parallel-computing-in-r.Rmd
+++ /dev/null
@@ -1,294 +0,0 @@
----
-title: "Additional Resources: Parallel Computing in R"
-author: "Matt Jones"
-date: "7/25/2017"
-output: html_document
----
-
-## Parallel Computing in R
-
-```{r setup, include=FALSE}
-knitr::opts_chunk$set(echo = TRUE)
-```
-
-### Learning Outcomes
-
-- Understand what parallel computing is and when it may be useful
-- Understand how parallelism can work
-- Review sequential loops and *apply functions
-- Understand and use the `parallel` package multicore functions
-- Understand and use the `foreach` package functions
-
-### Introduction
-
-Processing large amounts of data with complex models can be time consuming. New types of sensing means the scale of data collection today is massive. And modeled outputs can be large as well. For example, here's a 2 TB (that's Terabyte) set of modeled output data from [Ofir Levy et al. 2016](https://doi.org/10.5063/F1Z899CZ) that models 15 environmental variables at hourly time scales for hundreds of years across a regular grid spanning a good chunk of North America:
-
-
-
-There are over 400,000 individual netCDF files in the [Levy et al. microclimate data set](https://doi.org/10.5063/F1Z899CZ). Processing them would benefit massively from parallelization.
-
-Alternatively, think of remote sensing data. Processing airborne hyperspectral data can involve processing each of hundreds of bands of data for each image in a flight path that is repeated many times over months and years.
-
-
-
-
-### Why parallelism?
-
-Much R code runs fast and fine on a single processor. But at times, computations
-can be:
-
-- **cpu-bound**: Take too much cpu time
-- **memory-bound**: Take too much memory
-- **I/O-bound**: Take too much time to read/write from disk
-- **network-bound**: Take too much time to transfer
-
-To help with **cpu-bound** computations, one can take advantage of modern processor architectures that provide multiple cores on a single processor, and thereby enable multiple computations to take place at the same time. In addition, some machines ship with multiple processors, allowing large computations to occur across the entire cluster of those computers. Plus, these machines also have large amounts of memory to avoid **memory-bound** computing jobs.
-
-### Processors (CPUs) and Cores
-
-A modern CPU (Central Processing Unit) is at the heart of every computer. While
-traditional computers had a single CPU, modern computers can ship with mutliple
-processors, which in turn can each contain multiple cores. These processors and
-cores are available to perform computations.
-
-A computer with one processor may still have 4 cores (quad-core), allowing 4 computations
-to be executed at the same time.
-
-
-
-A typical modern computer has multiple cores, ranging from one or two in laptops
-to thousands in high performance compute clusters. Here we show four quad-core
-processors for a total of 16 cores in this machine.
-
-
-
-You can think of this as allowing 16 computations to happen at the same time. Theroetically, your computation would take 1/16 of the time (but only theoretically, more on that later).
-
-Historically, R has only utilized one processor, which makes it single-threaded. Which is a shame, because the 2017 MacBook Pro that I am writing this on is much more powerful than that:
-
-```{bash eval=FALSE}
-jones@powder:~$ sysctl hw.ncpu hw.physicalcpu
-hw.ncpu: 8
-hw.physicalcpu: 4
-```
-
-To interpret that output, this machine `powder` has 4 physical CPUs, each of which has
-two processing cores, for a total of 8 cores for computation. I'd sure like my R computations to use all of that processing power. Because its all on one machine, we can easily use *multicore* processing tools to make use of those cores. Now let's look at
-the computational server `aurora` at NCEAS:
-
-```{bash eval=FALSE}
-jones@aurora:~$ lscpu | egrep 'CPU\(s\)|per core|per socket'
-CPU(s): 88
-On-line CPU(s) list: 0-87
-Thread(s) per core: 2
-Core(s) per socket: 22
-NUMA node0 CPU(s): 0,2,4,6,8,10,12,14,16,18,20,22,24,26,28,30,32,34,36,38,40,42,44,46,48,50,52,54,56,58,60,62,64,66,68,70,72,74,76,78,80,82,84,86
-NUMA node1 CPU(s): 1,3,5,7,9,11,13,15,17,19,21,23,25,27,29,31,33,35,37,39,41,43,45,47,49,51,53,55,57,59,61,63,65,67,69,71,73,75,77,79,81,83,85,87
-```
-
-Now that's some compute power! Aurora has 384 GB of RAM, and ample storage. All still under the control of a single operating system.
-
-However, maybe one of these NSF-sponsored high performance computing clusters (HPC) is looking attractive about now:
-
-- [JetStream](https://jetstream-cloud.org/)
- - 640 nodes, 15,360 cores, 80TB RAM
-- [Stampede2]() at TACC is coming online in 2017
- - 4200 nodes, 285,600 cores
-
-Note that these clusters have multiple nodes (hosts), and each host has multiple cores. So this is really multiple computers clustered together to act in a coordinated fashion, but each node runs its own copy of the operating system, and is in many ways independent of the other nodes in the cluster. One way to use such a cluster would be to use just one of the nodes, and use a multi-core approach to parallelization to use all of the cores on that single machine. But to truly make use of the whole cluster, one must use parallelization tools that let us spread out our computations across multiple host nodes in the cluster.
-
-### When to parallelize
-
-It's not as simple as it may seem. While in theory each added processor would linearly increase the throughput of a computation, there is overhead that reduces that efficiency. For example, the code and, importantly, the data need to be copied to each additional CPU, and this takes time and bandwidth. Plus, new processes and/or threads need to be created by the operating system, which also takes time. This overhead reduces the efficiency enough that realistic performance gains are much less than theoretical, and usually do not scale linearly as a function of processing power. For example, if the time that a computation takes is short, then the overhead of setting up these additional resources may actually overwhelm any advantages of the additional processing power, and the computation could potentially take longer!
-
-In addition, not all of a task can be parallelized. Depending on the proportion, the expected speedup can be significantly reduced. Some propose that this may follow [Amdahl's Law](https://en.wikipedia.org/wiki/Amdahl%27s_law), where the speedup of the computation (y-axis) is a function of both the number of cores (x-axis) and the proportion of the computation that can be parallelized (see colored lines):
-
-```{r label="amdahl", echo=FALSE}
-library(ggplot2)
-library(tidyr)
-amdahl <- function(p, s) {
- return(1 / ( (1-p) + p/s ))
-}
-doubles <- 2^(seq(0,16))
-cpu_perf <- cbind(cpus = doubles, p50 = amdahl(.5, doubles))
-cpu_perf <- cbind(cpu_perf, p75 = amdahl(.75, doubles))
-cpu_perf <- cbind(cpu_perf, p85 = amdahl(.85, doubles))
-cpu_perf <- cbind(cpu_perf, p90 = amdahl(.90, doubles))
-cpu_perf <- cbind(cpu_perf, p95 = amdahl(.95, doubles))
-#cpu_perf <- cbind(cpu_perf, p99 = amdahl(.99, doubles))
-cpu_perf <- as.data.frame(cpu_perf)
-cpu_perf <- cpu_perf %>% gather(prop, speedup, -cpus)
-ggplot(cpu_perf, aes(cpus, speedup, color=prop)) +
- geom_line() +
- scale_x_continuous(trans='log2') +
- theme_bw() +
- labs(title = "Amdahl's Law")
-```
-
-
-So, its important to evaluate the computational efficiency of requests, and work to ensure that additional compute resources brought to bear will pay off in terms of increased work being done. With that, let's do some parallel computing...
-
-### Loops and repetitive tasks using lapply
-
-When you have a list of repetitive tasks, you may be able to speed it up by adding more computing power. If each task is completely independent of the others, then it is a prime candidate for executing those tasks in parallel, each on its own core. For example, let's build a simple loop that uses sample with replacement to do a bootstrap analysis. In this case, we select `Sepal.Length` and `Species` from the `iris` dataset, subset it to 100 observations, and then iterate across 10,000 trials, each time resampling the observations with replacement. We then run a logistic regression fitting species as a function of length, and record the coefficients for each trial to be returned.
-
-```{r label="bootstrap-loop"}
-x <- iris[which(iris[,5] != "setosa"), c(1,5)]
-trials <- 10000
-res <- data.frame()
-system.time({
- trial <- 1
- while(trial <= trials) {
- ind <- sample(100, 100, replace=TRUE)
- result1 <- glm(x[ind,2]~x[ind,1], family=binomial(logit))
- r <- coefficients(result1)
- res <- rbind(res, r)
- trial <- trial + 1
- }
-})
-```
-
-The issue with this loop is that we execute each trial sequentially, which means that only one of our 8 processors on this machine are in use. In order to exploit parallelism, we need to be able to dispatch our tasks as functions, with one task
-going to each processor. To do that, we need to convert our task to a function, and then use the `*apply()` family of R functions to apply that function to all of the members of a set. In R, using `apply` used to be faster than the equivalent code in a loop, but now they are similar due to optimizations in R loop handling. However, using the function allows us to later take advantage of other approaches to parallelization. Here's the same code rewritten to use `lapply()`, which applies a function to each of the members of a list (in this case the trials we want to run):
-
-```{r label="bootstrap-lapply"}
-x <- iris[which(iris[,5] != "setosa"), c(1,5)]
-trials <- seq(1, 10000)
-boot_fx <- function(trial) {
- ind <- sample(100, 100, replace=TRUE)
- result1 <- glm(x[ind,2]~x[ind,1], family=binomial(logit))
- r <- coefficients(result1)
- res <- rbind(data.frame(), r)
-}
-system.time({
- results <- lapply(trials, boot_fx)
-})
-```
-
-### Approaches to parallelization
-When parallelizing jobs, one can:
-
-- Use the multiple cores on a local computer through `mclapply`
-- Use multiple processors on local (and remote) machines using `makeCluster` and `clusterApply`
-
- - In this approach, one has to manually copy data and code to each cluster member using `clusterExport`
- - This is extra work, but sometimes gaining access to a large cluster is worth it
-
-### Parallelize using: mclapply
-
-The `parallel` library can be used to send tasks (encoded as function calls) to each of the processing cores on your machine in parallel. This is done by using the `parallel::mclapply` function, which is analogous to `lapply`, but distributes the tasks to multiple processors. `mclapply` gathers up the responses from each of these function calls, and returns a list of responses that is the same length as the list or vector of input data (one return per input item).
-
-```{r label="kmeans-comparison"}
-library(parallel)
-library(MASS)
-
-starts <- rep(100, 40)
-fx <- function(nstart) kmeans(Boston, 4, nstart=nstart)
-numCores <- detectCores()
-numCores
-
-system.time(
- results <- lapply(starts, fx)
-)
-
-system.time(
- results <- mclapply(starts, fx, mc.cores = numCores)
-)
-```
-
-Now let's demonstrate with our bootstrap example:
-```{r label="bootstrap-mclapply"}
-x <- iris[which(iris[,5] != "setosa"), c(1,5)]
-trials <- seq(1, 10000)
-boot_fx <- function(trial) {
- ind <- sample(100, 100, replace=TRUE)
- result1 <- glm(x[ind,2]~x[ind,1], family=binomial(logit))
- r <- coefficients(result1)
- res <- rbind(data.frame(), r)
-}
-system.time({
- results <- mclapply(trials, boot_fx, mc.cores = numCores)
-})
-```
-
-### Parallelize using: foreach and doParallel
-
-The normal `for` loop in R looks like:
-
-```{r label="for-loop"}
-for (i in 1:3) {
- print(sqrt(i))
-}
-```
-
-The `foreach` method is similar, but uses the sequential `%do%` operator to indicate an expression to run. Note the difference in the returned data structure.
-```{r label="foreach-loop"}
-library(foreach)
-foreach (i=1:3) %do% {
- sqrt(i)
-}
-```
-
-In addition, `foreach` supports a parallelizable operator `%dopar%` from the `doParallel` package. This allows each iteration through the loop to use different cores or different machines in a cluster. Here, we demonstrate with using all the cores on the current machine:
-```{r label="foreach-doParallel"}
-library(foreach)
-library(doParallel)
-registerDoParallel(numCores) # use multicore, set to the number of our cores
-foreach (i=1:3) %dopar% {
- sqrt(i)
-}
-
-# To simplify output, foreach has the .combine parameter that can simplify return values
-
-# Return a vector
-foreach (i=1:3, .combine=c) %dopar% {
- sqrt(i)
-}
-
-# Return a data frame
-foreach (i=1:3, .combine=rbind) %dopar% {
- sqrt(i)
-}
-```
-
-The [doParallel vignette](https://cran.r-project.org/web/packages/doParallel/vignettes/gettingstartedParallel.pdf) on CRAN shows a much more realistic example, where one can use `%dopar% to parallelize a bootstrap analysis where a data set is resampled 10,000 times and the analysis is rerun on each sample, and then the results combined:
-
-```{r label="foreach-bootstrap"}
-# Let's use the iris data set to do a parallel bootstrap
-# From the doParallel vignette, but slightly modified
-x <- iris[which(iris[,5] != "setosa"), c(1,5)]
-trials <- 10000
-system.time({
- r <- foreach(icount(trials), .combine=rbind) %dopar% {
- ind <- sample(100, 100, replace=TRUE)
- result1 <- glm(x[ind,2]~x[ind,1], family=binomial(logit))
- coefficients(result1)
- }
-})
-
-# And compare that to what it takes to do the same analysis in serial
-system.time({
- r <- foreach(icount(trials), .combine=rbind) %do% {
- ind <- sample(100, 100, replace=TRUE)
- result1 <- glm(x[ind,2]~x[ind,1], family=binomial(logit))
- coefficients(result1)
- }
-})
-
-# When you're done, clean up the cluster
-stopImplicitCluster()
-```
-
-
-### Summary
-
-In this lesson, we showed examples of computing tasks that are likely limited by the number of CPU cores that can be applied, and we reviewed the architecture of computers to understand the relationship between CPU processors and cores. Next, we reviewed the way in which traditional `for` loops in R can be rewritten as functions that are applied to a list serially using `lapply`, and then how the `parallel` package `mclapply` function can be substituted in order to utilize multiple cores on the local computer to speed up computations. Finally, we installed and reviewed the use of the `foreach` package with the `%dopar` operator to accomplish a similar parallelization using multiple cores.
-
-### Readings and tutorials
-
-- [Multicore Data Science with R and Python](https://blog.dominodatalab.com/multicore-data-science-r-python/)
-- [Beyond Single-Core R](https://ljdursi.github.io/beyond-single-core-R/#/) by Jonoathan Dursi (also see [GitHub repo for slide source](https://github.com/ljdursi/beyond-single-core-R))
-- The venerable [Parallel R](http://shop.oreilly.com/product/0636920021421.do) by McCallum and Weston (a bit dated on the tooling, but conceptually solid)
-- The [doParallel Vignette](https://cran.r-project.org/web/packages/doParallel/vignettes/gettingstartedParallel.pdf)
-
-
diff --git a/materials/sections/r-beginning-programming.Rmd b/materials/sections/r-beginning-programming.Rmd
deleted file mode 100644
index 3b44302a..00000000
--- a/materials/sections/r-beginning-programming.Rmd
+++ /dev/null
@@ -1,523 +0,0 @@
-## Learning Objectives
-
-In this lesson we will:
-
-- get oriented to the RStudio interface
-- run code and basic arithmetic in the console
-- be introduced to built-in R functions
-- be introduced to an R script
-- learn to use the help pages
-
-## Introduction and Motivation
-
-
-
-There is a vibrant community out there that is collectively developing increasingly easy to use and powerful open source programming tools. The changing landscape of programming is making learning how to code easier than it ever has been. Incorporating programming into analysis workflows not only makes science more efficient, but also more computationally reproducible. In this course, we will use the programming language R, and the accompanying integrated development environment (IDE) RStudio. R is a great language to learn for data-oriented programming because it is widely adopted, user-friendly, and (most importantly) open source!
-
-So what is the difference between R and RStudio? Here is an analogy to start us off. **If you were a chef, R is a knife.** You have food to prepare, and the knife is one of the tools that you'll use to accomplish your task.
-
-And **if R were a knife, RStudio is the kitchen**. RStudio provides a place to do your work! Other tools, communication, community, it makes your life as a chef easier. RStudio makes your life as a researcher easier by bringing together other tools you need to do your work efficiently - like a file browser, data viewer, help pages, terminal, community, support, the list goes on. So it's not just the infrastructure (the user interface or IDE), although it is a great way to learn and interact with your variables, files, and interact directly with git. It's also data science philosophy, R packages, community, and more. Although you can prepare food without a kitchen and we could learn R without RStudio, that's not what we're going to do. We are going to take advantage of the great RStudio support, and learn R and RStudio together.
-
-Something else to start us off is to mention that you are learning a new language here. It's an ongoing process, it takes time, you'll make mistakes, it can be frustrating, but it will be overwhelmingly awesome in the long run. We all speak at least one language; it's a similar process, really. And no matter how fluent you are, you'll always be learning, you'll be trying things in new contexts, learning words that mean the same as others, etc, just like everybody else. And just like any form of communication, there will be miscommunication that can be frustrating, but hands down we are all better off because of it.
-
-While language is a familiar concept, programming languages are in a different context from spoken languages and you will understand this context with time. For example: you have a concept that there is a first meal of the day, and there is a name for that: in English it's "breakfast". So if you're learning Spanish, you could expect there is a word for this concept of a first meal. (And you'd be right: "desayuno"). **We will get you to expect that programming languages also have words (called functions in R) for concepts as well**. You'll soon expect that there is a way to order values numerically. Or alphabetically. Or search for patterns in text. Or calculate the median. Or reorganize columns to rows. Or subset exactly what you want. We will get you to increase your expectations and learn to ask and find what you're looking for.
-
-### R Resources
-
-This lesson is a combination of excellent lessons by others. Huge thanks to [Julie Lowndes](https://jules32.github.io/) for writing most of this content and letting us build on her material, which in turn was built on [Jenny Bryan's](https://jennybryan.org/about/) materials. We highly recommend reading through the original lessons and using them as reference.
-
-+----------------------+---------------------------------------------------------------------------------------------------------------------------------------------------------------------------------------------------+
-| Learning R Resources | - [Introduction to R](http://www.datacarpentry.org/R-ecology-lesson/01-intro-to-r.html) lesson in [Data Carpentry's R for data analysis](http://www.datacarpentry.org/R-ecology-lesson/) course |
-| | - Jenny Bryan's Stat 545 [course materials](https://stat545.com/r-basics.html) |
-| | - [Julie Lowndes' Data Science Training for the Ocean Health Index](http://ohi-science.org/data-science-training/) |
-| | - Learn R in the console with [swirl](https://swirlstats.com/) |
-| | - [Programming in R](http://ohi-science.org/data-science-training/programming.html) |
-| | - [R, RStudio, RMarkdown](http://ohi-science.org/data-science-training/rstudio.html) |
-+----------------------+---------------------------------------------------------------------------------------------------------------------------------------------------------------------------------------------------+
-| Community Resources | - [NCEAS' EcoDataScience](https://eco-data-science.github.io/) |
-| | |
-| | - [R-Ladies](https://rladies.org/) |
-| | |
-| | - [rOpenSci](https://ropensci.org/community/) |
-| | |
-| | - [Minorities in R (MiR)](https://mircommunity.com/) |
-| | |
-| | - Twitter - there is *a lot* here but some hashtags to start with are: |
-| | |
-| | - #rstats |
-| | |
-| | - #TidyTuesday |
-| | |
-| | - #dataviz |
-+----------------------+---------------------------------------------------------------------------------------------------------------------------------------------------------------------------------------------------+
-| Cheatsheets | - [Base R Cheatsheet](https://iqss.github.io/dss-workshops/R/Rintro/base-r-cheat-sheet.pdf) |
-| | - [LaTeX Equation Formatting](https://www.caam.rice.edu/~heinken/latex/symbols.pdf) |
-| | - [MATLAB/R Translation Cheatsheet](http://mathesaurus.sourceforge.net/octave-r.html) |
-+----------------------+---------------------------------------------------------------------------------------------------------------------------------------------------------------------------------------------------+
-
-## RStudio Interface
-
-Ok let's get started with a tour of RStudio.
-
-
-
-Notice the default panes:
-
-- Console (entire left)
-- Environment/History (tabbed in upper right)
-- Files/Plots/Packages/Help (tabbed in lower right)
-
-> **Quick Tip:** you can change the default location of the panes, among many other things, see [Customizing RStudio](https://support.rstudio.com/hc/en-us/articles/200549016-Customizing-RStudio).
-
-An important first question: **where are we?**
-
-If you've just opened RStudio for the first time, you'll be in your Home directory. This is noted by the `~/` at the top of the console. You can see too that the Files pane in the lower right shows what is in the Home directory where you are. You can navigate around within that Files pane and explore, but note that you won't change where you are: even as you click through you'll still be Home: `~/`.
-
-
-
-## R Basics: Running code in the Console
-
-We can run code in a couple of places in RStudio, including the Console, let's start there.
-
-At it's most basic, we can use R as a calculator, let's try a couple of examples in the console.
-
-While there are many cases where it makes sense to type code directly in to the the console, it is not a great place to write most of your code since you can't save what you ran. A better way is to create an R script, and write your code there. Then when you run your code from the script, you can save it when you are done. We're going to continue doing writing code in the console for now, but we'll code in an R script later in this chapter.
-
-```{r, eval=FALSE}
-# run in the console
-# really basic examples
-3*4
-3+4
-3-4
-3/4
-```
-
-> **Quick Tip:** When you're in the console you'll see a greater than sign (\>) at the start of a line. This is called the "prompt" and when we see it, it means R is ready to accept commands. If you see a plus sign (+) in the Console, it means R is waiting on additional information before running. You can always press escape (esc) to return to the prompt. Try practicing this by running `3*` (or any incomplete expression) in the console.
-
-### Objects in R
-
-Let's say the value of 12 that we got from running `3*4` is a really important value we need to keep. To keep information in R, we will need to create an **object**. The way information is stored in R is through objects.
-
-We can assign a value of a mathematical operation (and more!) to an object in R using the assignment operator, `<-` (greater than sign and minus sign). All objects in R are created using the assignment operator, following this form: `object_name <- value`.
-
-**Exercise:** Assign `3*4` to an object called `important_value` and then inspect the object you just created.
-
-```{r, eval=FALSE}
-# in my head I hear, e.g., "important_value gets 12".
-important_value <- 3*4
-```
-
-Notice how after creating the object, R doesn't print anything. However, we know our code worked because we see the object, and the value we wanted to store is now visible in our **Global Environment**. We can force R to print the value of the object by calling the object name (aka typing it out) or by using parentheses.
-
-> **Quick Tip:** When you begin typing an object name RStudio will automatically show suggested completions for you that you can select by hitting `tab`, then press `return.`
-
-```{r, eval=FALSE}
-# printing the object by calling the object name
-important_value
-# printing the object by wrapping the assignment syntax in parentheses
-(important_value <- 3*4)
-```
-
-> **Quick Tip:** Use the up and down arrow keys to call your command history, with the most recent commands being called first.
-
-### Naming Conventions
-
-Before we run more calculations, let's talk about naming objects. For the object, `important_value` I used an underscore to separate the object name. This naming convention is called **snake case**. There are other naming conventions including, but not limited to:
-
-- i_use_snake_case
-
-- someUseCamelCase
-
-- SomeUseUpperCamelCaseAlsoCalledPascalCase
-
-Choosing a [naming convention](https://en.wikipedia.org/wiki/Naming_convention_(programming)#:~:text=In%20computer%20programming%2C%20a%20naming,in%20source%20code%20and%20documentation) is a personal preference, but once you choose one - be consistent! A consistent naming convention will increase the readability of your code for others and your future self.
-
-> **Quick Tip:** Object names cannot start with a digit and cannot contain certain characters such as a comma or a space.
-
-### R calculations with objects
-
-Now that we know what an object is in R and how to create one let's learn how to use an object in calculations. Let's say we have the weight of a dog in kilograms. Create the object `weight_kg` and assign it a value of 55.
-
-```{r, purl=FALSE}
-# weight of a dog in kilograms
-weight_kg <- 55
-```
-
-Now that R has `weight_kg` saved in the Global Environment, we can run calculations with it. For instance, we may want to convert weight into pounds (weight in pounds is 2.2 times the weight in kg):
-
-```{r, results='hide'}
-# converting weight from kilograms to pounds
-2.2 * weight_kg
-```
-
-You can also store more than one value in a single object. Storing a series of weights in a single object is a convenient way to perform the same operation on multiple values at the same time. One way to create such an object is with the function `c()`, which stands for combine or concatenate.
-
-First let's create a *vector* of weights in kilograms using `c()` (we'll talk more about vectors in the next section, [Data structures in R](#data_structures).
-
-```{r, results='hide'}
-# create a vector of weights in kilograms
-weight_kg <- c(55, 25, 12)
-# call the object to inspect
-weight_kg
-```
-
-Now convert the object `weight_kg` to pounds.
-
-```{r, results='hide'}
-# covert `weight_kg` to pounds
-weight_kg * 2.2
-```
-
-Wouldn't it be helpful if we could save these new weight values we just converted? This might be important information we may need for a future calculation. How would you save these new weights in pounds?
-
-```{r, results='hide'}
-# create a new object
-weight_lb <- weight_kg * 2.2
-# call `weight_lb` to check if the information you expect is there
-weight_lb
-```
-
-> **Quick Tip:** You will make many objects and the assignment operator `<-` can be tedious to type over and over. Instead, use **RStudio's keyboard shortcut: `option` + `-` (the minus sign)**. Notice that RStudio automatically surrounds `<-` with spaces, which demonstrates a useful code formatting practice. Code is miserable to read on a good day. Give your eyes a break and use spaces. RStudio offers many handy [keyboard shortcuts](https://support.rstudio.com/hc/en-us/articles/200711853-Keyboard-Shortcuts). Also, `option`+`Shift`+`K` brings up a keyboard shortcut reference card. For more RStudio tips, check out our Master of Environmental Data Science (MEDS) workshop: [IDE Tips & Tricks](https://ucsb-meds.github.io/IDE-tips-and-tricks/#/title-slide).
-
-### Logical operators and expressions
-
-A moment about **logical operators and expressions**. We can ask questions about the object, `weight_lb` we made.
-
-- `==` means 'is equal to'
-- `!=` means 'is not equal to'
-- `<` means 'is less than'
-- `>` means 'is greater than'
-- `<=` means 'is less than or equal to'
-- `>=` means 'is greater than or equal to'
-
-```{r, results='hide'}
-# examples using logical operators and expressions
-weight_lb == 2
-weight_lb >= 30
-weight_lb != 5
-```
-
-### Data structures in R {#data_structures}
-
-**A vector is the most common and most basic data structure in R**. A common vector you will interact with often are **atomic vectors**. To put it simply, atomic vectors *only* contain elements of the *same* data type.
-
-Vectors are foundational for other data structures in R, including data frames, and while we won't go into detail about other data structures there are great resources online that do. We recommend the chapter [Vectors](https://adv-r.hadley.nz/vectors-chap.html) from the online book [Advanced R](https://adv-r.hadley.nz/index.html) by Hadley Wickham.
-
-[](https://adv-r.hadley.nz/vectors-chap.html)
-
-```{r}
-# atomic vector examples #
-# character vector
-chr_atomic_vector <- c("hello", "good bye", "see you later")
-# numeric vector
-numeric_atomic_vector <- c(5, 1.3, 10)
-# logical vector
-boolean_atomic_vector <- c(TRUE, FALSE, TRUE)
-```
-
-### Data types in R
-
-The most common data types in R are:
-
-- `boolean` (also called `logical`): data take on the value of either `TRUE`, `FALSE`, or `NA`. `NA` is used to represent missing values.
-
-- `character`: data are used to represent string values. You can think of character strings as something like a word (or multiple words). A special type of character string is a `factor`, which is a string but with additional attributes (like levels or an order).
-
-- `integer`: data are whole numbers (those numbers without a decimal point). To explicitly create an integer data type, use the suffix `L` (e.g. `2L`).
-
-- `numeric` (also called `double`): data are numbers that contain a decimal.
-
-Some less common data types are:
-
-- `complex`: data are complex numbers with real and imaginary parts.
-
-- `raw`: data are raw bytes.
-
-Let's create an object that has been assigned a string.
-
-```{r}
-science_rocks <- "yes it does!"
-```
-
-In R, this is called a "string", and R knows it's a word and not a number because it has quotes `" "`. You can work with strings in your data in R easily thanks to the [`stringr`](http://stringr.tidyverse.org/) and [`tidytext`](https://github.com/juliasilge/tidytext) packages.
-
-Strings and numbers lead us to an important concept in programming: that there are different "classes" or types of objects. Everything in R is an object - an object is a variable, function, data structure, or method that you have written to your environment. The operations you can do with an object will depend on what type of object it is because each object has their own specialized format, designed for a specific purpose. This makes sense! Just like you wouldn't do certain things with your car (like use it to eat soup), you won't do certain operations with character objects (strings).
-
-Try running the following line in your console:
-
-```{r, eval=FALSE}
-"Hello world!" * 3
-```
-
-What happened? Why?
-
-> **Quick Tip:** You can see what data type an object is using the `class()` function, or you can use a logical test such as: `is.numeric()`, `is.character()`, `is.logical()`, and so on.
-
-```{r, eval=FALSE}
-class(science_rocks) # returns character
-is.numeric(science_rocks) # returns FALSE
-is.character(science_rocks) # returns TRUE
-```
-
-### Clearing the environment
-
-Now look at the objects in your environment (workspace) -- in the upper right pane. The workspace is where user-defined objects accumulate.
-
-
-
-You can also get a listing of these objects with a few different R commands:
-
-```{r}
-objects()
-ls()
-```
-
-If you want to remove the object named `weight_kg`, you can do this:
-
-```{r}
-rm(weight_kg)
-```
-
-To remove everything:
-
-```{r}
-rm(list = ls())
-```
-
-Or click the broom in RStudio's Environment pane.
-
-> **Quick Tip:** it's good practice to clear your environment. Over time your Global Environmental will fill up with many objects, and this can result in unexpected errors or objects being overridden with unexpected values. Also it's difficult to read / reference your environment when it's cluttered!
-
-## Running code in an R script
-
-So far we've been running code in the console, let's try running code in an R script. An R script is a simple text file. And RStudio copies R commands from this text file and inserts them into the R console as if you were manually entering commands yourself directly into R.
-
-#### Creating an R script {.unnumbered .setup}
-
-In your RStudio server session, follow these steps to set up your R script:
-
-- In the "File" menu, select "New File"
-- Click "R Script" from the list of options
-
-RStudio should open your R script automatically after creating it. Notice a new pane appears above the console. This is called the **source pane** and is where we write and edit R code and documents. This pane is only present if there are files open in the editor.
-
-### R Functions
-
-So far we've learned some of the basic syntax and concepts of R programming, and how to navigate RStudio, but we haven't done any complicated or interesting programming processes yet. This is where functions come in!
-
-A function is a way to group a set of commands together to undertake a task in a reusable way. When a function is executed, it produces a return value. We often say that we are "calling" a function when it is executed. Functions can be user defined and saved to an object using the assignment operator, so you can write whatever functions you need, but R also has a mind-blowing collection of built-in functions ready to use. To start, we will be using some built in R functions.
-
-All functions are called using the same syntax: function name with parentheses around what the function needs in order to do what it was built to do. The pieces of information that the function needs to do its job are called arguments. So the syntax will look something like: `result_value <- function_name(argument1 = value1, argument2 = value2, ...)`.
-
-### Running code in an R script
-
-Notice that after you finish typing your code, pressing enter doesn't run your code. Running code in an R script is different than running code in the Console. To interpret and run the code you've written, R needs you to send the code from the script or editor to the Console. Some common ways to run code in an R script include:
-
-- Place your cursor on the line of code you want to run and use the shortcut `command` and `return` or use the `Run` button in the top right of the Source pane.
-
-- Highlight the code you want to run, then use the shortcut above or `Run` button.
-
-### Use the `mean()` function to run a more complex calculation
-
-Since we just cleared our environment, let's recreate our weight object again. This time let's say we have three dog weights in pounds:
-
-```{r}
-weight_lb <- c(55, 25, 12)
-```
-
-and use the `mean()` function to calculate the mean weight. As you might expect, this is a function that will take the mean of a set of numbers. Very convenient!
-
-```{r, results='hide'}
-mean(weight_lb)
-```
-
-Save the mean to an object called `mean_weight_lb`.
-
-```{r}
-mean_weight_lb <- mean(weight_lb)
-```
-
-Let's say each of the dogs gained 5 pounds and we need to update our vector, so let's change our object's value by assigning it new and update values.
-
-```{r}
-weight_lb <- c(60, 30, 17)
-```
-
-Call `mean_weight_lb` in the console or take a look at your Global Environment. Is that the value you expected? Why or why not?
-
-Notice that `mean_weight_lb` did not change. This demonstrates an important programming concept: assigning a value to one object does not change the values of other objects.
-
-Now, that we understand why the object's value hasn't changed - how do we update the value of `mean_weight_lb`? How is an R script useful for this?
-
-It's important to understand how an R script runs - which is top to bottom. This order of operations is important because if you are running code line by line, the values in object may be unexpected. When you are done writing your code in an R script, it's good practice to clear your Global Environment and use the `Run` button and select "Run all" to test that your script successfully runs top to bottom.
-
-### Use the `read.csv()` function to read a file into R
-
-So far we have learned how to assign values to objects in R, and what a function is, but we haven't quite put it all together yet with real data yet. To do this, we will introduce the function `read.csv()`, which will be in the first lines of many of your future scripts. It does exactly what it says, it reads in a csv file to R.
-
-Since this is our first time using this function, first access the help page for `read.csv()`. This has a lot of information in it, as this function has a lot of arguments, and the first one is especially important - we have to tell it what file to look for. Let's get a file!
-
-#### Download a file from the Arctic Data Center {.unnumbered .setup}
-
-Follow these steps to get set up for the next exercise:
-
-1. Navigate to this dataset by Craig Tweedie that is published on the Arctic Data Center. [Craig Tweedie. 2009. North Pole Environmental Observatory Bottle Chemistry. Arctic Data Center. doi:10.18739/A25T3FZ8X.](http://doi.org/10.18739/A25T3FZ8X)
-2. Download the first csv file called `BGchem2008data.csv` by clicking the "download" button next to the file.
-3. Move this file from your `Downloads` folder into a place you can more easily find it. E.g.: a folder called `data` in your previously-created directory `training_yourname`.
-
-### Use `read.csv()` to read in Arctic Data Center data
-
-Now we have to tell `read.csv()` how to find the file. We do this using the `file` argument which you can see in the usage section in the help page. In R, you can either use absolute paths (which will start with your home directory `~/`) or paths **relative to your current working directory.** RStudio has some great auto-complete capabilities when using relative paths, so we will go that route. Assuming you have moved your file to a folder within `training_yourname` called `data`, and your working directory is your project directory (`training_yourname`) your `read.csv()` call will look like this:
-
-```{r, eval = F}
-# reading in data using relative paths
-bg_chem_dat <- read.csv("data/BGchem2008data.csv")
-```
-
-You should now have an object of the class `data.frame` in your environment called `bg_chem_dat`. Check your environment pane to ensure this is true. Or you can check the class using the function `class()` in the console.
-
-Note that in the help page there are a whole bunch of arguments that we didn't use in the call above. Some of the arguments in function calls are optional, and some are required. Optional arguments will be shown in the usage section with a `name = value` pair, with the default value shown. If you do not specify a `name = value` pair for that argument in your function call, the function will assume the default value (example: `header = TRUE` for `read.csv`). Required arguments will only show the name of the argument, without a value. Note that the only required argument for `read.csv()` is `file`.
-
-You can always specify arguments in `name = value` form. But if you do not, R attempts to resolve by position. So above, it is assumed that we want `file = "data/BGchem2008data.csv"`, since file is the first argument. If we wanted to add another argument, say `stringsAsFactors`, we need to specify it explicitly using the `name = value` pair, since the second argument is `header`. For functions I call often, I use this resolve by position for the first argument or maybe the first two. After that, I always use `name = value`.
-
-Many R users (including myself) will override the default `stringsAsFactors` argument using the following call:
-
-```{r, eval = F}
-# absolute file path
-bg_chem <- read.csv("Documents/arctic_training_files/data/BGchem2008data.csv",
- stringsAsFactors = FALSE)
-```
-
-```{r, eval = F}
-# relative file path
-bg_chem <- read.csv("data/BGchem2008data.csv",
- stringsAsFactors = FALSE)
-```
-
-### Using `data.frames`
-
-A `data.frame` is a two dimensional data structure in R that mimics spreadsheet behavior. It is a collection of rows and columns of data, where each column has a name and represents a variable, and each row represents an observation containing a measurement of that variable. When we ran `read.csv()`, the object `bg_chem_dat` that we created is a `data.frame`. There are a bunch of ways R and RStudio help you explore data frames. Here are a few, give them each a try:
-
-- click on the word `bg_chem_dat` in the environment pane
-- click on the arrow next to `bg_chem_dat` in the environment pane
-- execute `head(bg_chem_dat)` in the console
-- execute `View(bg_chem_dat)` in the console
-
-Usually we will want to run functions on individual columns in a `data.frame`. To call a specific column, we use the list subset operator `$`. Say you want to look at the first few rows of the `Date` column only. This would do the trick:
-
-```{r, eval=FALSE}
-head(bg_chem_dat$Date)
-```
-
-How about calculating the mean temperature of all the CTD samples?
-
-```{r, eval=FALSE}
-mean(bg_chem_dat$CTD_Temperature)
-```
-
-Or, if we want to save this to a variable to use later:
-
-```{r, eval=FALSE}
-mean_temp <- mean(bg_chem_dat$CTD_Temperature)
-```
-
-You can also create basic plots using the list subset operator.
-
-```{r, eval=FALSE}
-plot(x = bg_chem_dat$CTD_Depth,
- y = bg_chem_dat$CTD_Temperature)
-```
-
-There are many more advanced tools and functions in R that will enable you to make better plots using cleaner syntax, we will cover some of these later in the course.
-
-> Exercise: Spend a few minutes exploring this dataset. Try out different functions on columns using the list subset operator and experiment with different plots.
-
-## Getting help using help pages
-
-What if you know the name of the function that you want to use, but don't know exactly how to use it? Thankfully RStudio provides an easy way to access the help documentation for functions.
-
-To access the help page for `read.csv`()`, enter the following into your console:
-
-```{r, eval = F}
-?read.csv
-```
-
-The help pane will show up in the lower right hand corner of your RStudio.
-
-The help page is broken down into sections:
-
-- Description: An extended description of what the function does.
-- Usage: The arguments of the function(s) and their default values.
-- Arguments: An explanation of the data each argument is expecting.
-- Details: Any important details to be aware of.
-- Value: The data the function returns.
-- See Also: Any related functions you might find useful.
-- Examples: Some examples for how to use the function.
-
-> Exercise: Talk to your neighbor(s) and look up the help file for a function that you know or expect to exist. Here are some ideas: `?getwd()`, `?plot()`, `?min()`, `?max()`, `?log()`).
-
-And there's also help for when you only sort of remember the function name: double-question mark:
-
-```{r, eval=F}
-??install
-```
-
-Not all functions have (or require) arguments:
-
-```{r, eval=FALSE}
-?date()
-```
-
-## Error messages are your friends
-
-There is an implicit contract with the computer/scripting language: Computer will do tedious computation for you. In return, you will be completely precise in your instructions. Typos matter. Case matters. Pay attention to how you type.
-
-Remember that this is a language, not dissimilar to English! There are times you aren't understood -- it's going to happen. There are different ways this can happen. Sometimes you'll get an error. This is like someone saying 'What?' or 'Pardon'? Error messages can also be more useful, like when they say 'I didn't understand this specific part of what you said, I was expecting something else'. That is a great type of error message. Error messages are your friend. Google them (copy-and-paste!) to figure out what they mean. Note that knowing how to Google is a skill and takes practice - use our [Masters of Environmental Data Science](https://bren.ucsb.edu/masters-programs/master-environmental-data-science) (MEDS) program workshop [Teach Me How to Google](https://ucsb-meds.github.io/teach-me-how-to-google/#1) as a guide.
-
-::: {style="width:400px"}
-
-:::
-
-And also know that there are errors that can creep in more subtly, without an error message right away, when you are giving information that is understood, but not in the way you meant. Like if I'm telling a story about tables and you're picturing where you eat breakfast and I'm talking about data. This can leave me thinking I've gotten something across that the listener (or R) interpreted very differently. And as I continue telling my story you get more and more confused... So write clean code and check your work as you go to minimize these circumstances!
-
-#### R says my object is not found
-
-New users will frequently see errors that look like this: `Error in mean(myobject) : object 'myobject' not found`
-
-This means that you do not have an object called `myobject` saved in your environment. The common reasons for this are:
-
-- **typo**: make sure your object name is spelled exactly like what shows up in the console. Remember R is case sensitive.
-- **not writing to a variable**: note that the object is only saved in the environment if you use the assignment operator, eg: `myobject <- read.csv(...)`
-- **not executing the line in your script**: remember that writing a line of code in a script or RMarkdown document is not the same as writing in the console, you have to execute the line of code using command + enter or using one of the several ways in the RStudio graphical user interface.
-
-## R Packages
-
-R packages are the building blocks of computational reproducibility in R. Each package contains a set of related functions that enable you to more easily do a task or set of tasks in R. There are thousands of community-maintained packages out there for just about every imaginable use of R - including many that you have probably never thought of!
-
-To install a package, we use the syntax `install.packages("packge_name")`. A package only needs to be installed once, so this code can be run directly in the console if needed. Generally, you don't want to save your install package calls in a script, because when you run the script it will re-install the package, which you only need to do once, or if you need to update the package.
-
-Use the chunk below to check that you have all the necessary packages installed for the course:
-
-```{r, eval = FALSE}
-packages <- c("readr",
- "dplyr",
- "tidyr",
- "googlesheets4",
- "tidytext",
- "wordcloud",
- "reshape2",
- "ggplot2",
- "viridis",
- "scales",
- "leaflet",
- "sf",
- "ggmap",
- "DT",
- "rmarkdown",
- "knitr")
-
-for (package in packages) {
- if (!(package %in% installed.packages())) { install.packages(package) }
- }
-rm(packages) # remove variable from workspace
-```
diff --git a/materials/sections/r-creating-functions.Rmd b/materials/sections/r-creating-functions.Rmd
deleted file mode 100644
index 714f46e2..00000000
--- a/materials/sections/r-creating-functions.Rmd
+++ /dev/null
@@ -1,221 +0,0 @@
-```{r message=FALSE, warning=FALSE, echo=FALSE}
-library(DT)
-```
-
-## Creating R Functions
-
-Many people write R code as a single, continuous stream of commands, often drawn
-from the R Console itself and simply pasted into a script. While any script
-brings benefits over non-scripted solutions, there are advantages to breaking
-code into small, reusable modules. This is the role of a `function` in R. In
-this lesson, we will review the advantages of coding with functions, practice
-by creating some functions and show how to call them, and then do some exercises
-to build other simple functions.
-
-#### Learning outcomes
-
-- Learn why we should write code in small functions
-- Write code for one or more functions
-- Document functions to improve understanding and code communication
-
-### Why functions?
-
-In a word:
-
-- DRY: Don't Repeat Yourself
-
-By creating small functions that only one logical task and do it well, we quickly
-gain:
-
-- Improved understanding
-- Reuse via decomposing tasks into bite-sized chunks
-- Improved error testing
-
-
-#### Temperature conversion {-}
-
-Imagine you have a bunch of data measured in Fahrenheit and you want to convert
-that for analytical purposes to Celsius. You might have an R script
-that does this for you.
-
-```{r}
-airtemps <- c(212, 30.3, 78, 32)
-celsius1 <- (airtemps[1]-32)*5/9
-celsius2 <- (airtemps[2]-32)*5/9
-celsius3 <- (airtemps[3]-32)*5/9
-```
-
-Note the duplicated code, where the same formula is repeated three times. This
-code would be both more compact and more reliable if we didn't repeat ourselves.
-
-#### Creating a function {-}
-
-Functions in R are a mechanism to process some input and return a value. Similarly
-to other variables, functions can be assigned to a variable so that they can be used
-throughout code by reference. To create a function in R, you use the `function` function (so meta!) and assign its result to a variable. Let's create a function that calculates
-celsius temperature outputs from fahrenheit temperature inputs.
-
-```{r}
-fahr_to_celsius <- function(fahr) {
- celsius <- (fahr-32)*5/9
- return(celsius)
-}
-```
-
-By running this code, we have created a function and stored it in R's global environment. The `fahr` argument to the `function` function indicates that the function we are creating takes a single parameter (the temperature in fahrenheit), and the `return` statement indicates that the function should return the value in the `celsius` variable that was calculated inside the function. Let's use it, and check if we got the same value as before:
-
-```{r}
-celsius4 <- fahr_to_celsius(airtemps[1])
-celsius4
-celsius1 == celsius4
-```
-
-Excellent. So now we have a conversion function we can use. Note that, because
-most operations in R can take multiple types as inputs, we can also pass the original vector of `airtemps`, and calculate all of the results at once:
-
-```{r}
-celsius <- fahr_to_celsius(airtemps)
-celsius
-```
-
-This takes a vector of temperatures in fahrenheit, and returns a vector of temperatures in celsius.
-
-#### Challenge {- .exercise}
-
-Now, create a function named `celsius_to_fahr` that does the reverse, it takes temperature data in celsius as input, and returns the data converted to fahrenheit. Then use that formula to convert the `celsius` vector back into a vector of fahrenheit values, and compare it to the original `airtemps` vector to ensure that your answers are correct. Hint: the formula for C to F conversions is `celsius*9/5 + 32`.
-
-```{r}
-# Your code goes here
-```
-
-Did you encounter any issues with rounding or precision?
-
-### Documenting R functions
-
-Functions need documentation so that we can communicate what they do, and why. The `roxygen2` package provides a simple means to document your functions so that you can explain what the function does, the assumptions about the input values, a description of the value that is returned, and the rationale for decisions made about implementation.
-
-Documentation in ROxygen is placed immediately before the function definition, and is indicated by a special comment line that always starts with the characters `#'`. Here's a documented version of a function:
-
-```{r}
-#' Convert temperature data from Fahrenheit to Celsius
-#'
-#' @param fahr Temperature data in degrees Fahrenheit to be converted
-#' @return temperature value in degrees Celsius
-#' @keywords conversion
-#' @export
-#' @examples
-#' fahr_to_celsius(32)
-#' fahr_to_celsius(c(32, 212, 72))
-fahr_to_celsius <- function(fahr) {
- celsius <- (fahr-32)*5/9
- return(celsius)
-}
-```
-
-Note the use of the `@param` keyword to define the expectations of input data, and the `@return` keyword for defining the value that is returned from the function. The `@examples` function is useful as a reminder as to how to use the function. Finally, the `@export` keyword indicates that, if this function were added to a package, then the function should be available to other code and packages to utilize.
-
-### Summary
-
-- Functions are useful to reduce redundancy, reuse code, and reduce errors
-- Build functions with the `function` function
-- Document functions with `roxygen2` comments
-
-
-#### Spoiler -- the exercise answered {-}
-
-Don't peek until you write your own...
-
-```{r}
-# Your code goes here
-celsius_to_fahr <- function(celsius) {
- fahr <- celsius*9/5 + 32
- return(fahr)
-}
-
-result <- celsius_to_fahr(celsius)
-airtemps == result
-```
-
-### Examples: Minimizing work with functions
-
-Functions can of course be as simple or complex as needed. They can be be very effective
-in repeatedly performing calculations, or for bundling a group of commands that are used
-on many different input data sources. For example, we might create a simple function that
-takes fahrenheit temperatures as input, and calculates both celsius and Kelvin temperatures.
-All three values are then returned in a list, making it very easy to create a comparison
-table among the three scales.
-
-```{r}
-convert_temps <- function(fahr) {
- celsius <- (fahr-32)*5/9
- kelvin <- celsius + 273.15
- return(list(fahr=fahr, celsius=celsius, kelvin=kelvin))
-}
-
-temps_df <- data.frame(convert_temps(seq(-100,100,10)))
-```
-
-```{r, echo = FALSE}
-datatable(temps_df)
-```
-
-
-Once we have a dataset like that, we might want to plot it. One thing that we do
-repeatedly is set a consistent set of display elements for creating graphs and plots.
-By using a function to create a custom `ggplot` theme, we can enable to keep key
-parts of the formatting flexible. FOr example, in the `custom_theme` function,
-we provide a `base_size` argument that defaults to using a font size of 9 points.
-Because it has a default set, it can safely be omitted. But if it is provided,
-then that value is used to set the base font size for the plot.
-
-```{r}
-custom_theme <- function(base_size = 9) {
- ggplot2::theme(
- axis.ticks = ggplot2::element_blank(),
- text = ggplot2::element_text(family = 'Helvetica', color = 'gray30', size = base_size),
- plot.title = ggplot2::element_text(size = ggplot2::rel(1.25), hjust = 0.5, face = 'bold'),
- panel.background = ggplot2::element_blank(),
- legend.position = 'right',
- panel.border = ggplot2::element_blank(),
- panel.grid.minor = ggplot2::element_blank(),
- panel.grid.major = ggplot2::element_line(colour = 'grey90', size = .25),
- legend.key = ggplot2::element_rect(colour = NA, fill = NA),
- axis.line = ggplot2::element_blank()
- )
-}
-
-library(ggplot2)
-
-ggplot(temps_df, mapping=aes(x=fahr, y=celsius, color=kelvin)) +
- geom_point() +
- custom_theme(10)
-
-```
-
-In this case, we set the font size to 10, and plotted the air temperatures. The `custom_theme`
-function can be used anywhere that one needs to consistently format a plot.
-
-But we can go further. One can wrap the entire call to ggplot in a function,
-enabling one to create many plots of the same type with a consistent structure. For
-example, we can create a `scatterplot` function that takes a data frame as input,
-along with a point_size for the points on the plot, and a font_size for the text.
-
-```{r}
-scatterplot <- function(df, point_size = 2, font_size=9) {
- ggplot(df, mapping=aes(x=fahr, y=celsius, color=kelvin)) +
- geom_point(size=point_size) +
- custom_theme(font_size)
-}
-```
-
-Calling that let's us, in a single line of code, create a highly customized plot
-but maintain flexibiity via the arguments passed in to the function. Let's set
-the point size to 3 and font to 16 to make the plot more legible.
-
-```{r}
-scatterplot(temps_df, point_size=3, font_size = 16)
-```
-
-Once these functions are set up, all of the plots built with them can be reformatted
-by changing the settings in just the functions, whether they were used to
-create 1, 10, or 100 plots.
diff --git a/materials/sections/r-creating-packages.Rmd b/materials/sections/r-creating-packages.Rmd
deleted file mode 100644
index a761855e..00000000
--- a/materials/sections/r-creating-packages.Rmd
+++ /dev/null
@@ -1,291 +0,0 @@
-## Creating R Packages
-
-### Learning Objectives
-
-In this lesson, you will learn:
-
-- The advantages of using R packages for organizing code
-- Simple techniques for creating R packages
-- Approaches to documenting code in packages
-
-### Why packages?
-
-Most R users are familiar with loading and utilizing packages in their work. And they know how rich CRAN is in providing for many conceivable needs. Most people have never created a package for their own work, and most think the process is too complicated. Really it's pretty straighforward and super useful in your personal work. Creating packages serves two main use cases:
-
-- Mechanism to redistribute reusable code (even if just for yourself)
-- Mechanism to reproducibly document analysis and models and their results
-
-Even if you don't plan on writing a package with such broad appeal such as, say, `ggplot2` or `dplyr`, you still might consider creating a package to contain:
-
-- Useful utility functions you write i.e. a [Personal Package](https://hilaryparker.com/2013/04/03/personal-r-packages/). Having a place to put these functions makes it much easier to find and use them later.
-- A set of shared routines for your lab or research group, making it easier to remain consistent within your team and also to save time.
-- The analysis accompanying a thesis or manuscript, making it all that much easier for others to reproduce your results.
-
-The `usethis`, `devtools` and `roxygen2` packages make creating and maintining a package to be a straightforward experience.
-
-### Install and load packages
-
-```{r, eval=FALSE}
-library(devtools)
-library(usethis)
-library(roxygen2)
-```
-
-### Create a basic package
-
-Thanks to the great [usethis](https://github.com/r-lib/usethis) package, it only takes one function call to create the skeleton of an R package using `create_package()`. Which eliminates pretty much all reasons for procrastination. To create a package called
-`mytools`, all you do is:
-
-```{r, eval=FALSE}
-setwd('..')
-create_package("mytools")
-```
-
- ✔ Setting active project to '/Users/jones/development/mytools'
- ✔ Creating 'R/'
- ✔ Creating 'man/'
- ✔ Writing 'DESCRIPTION'
- ✔ Writing 'NAMESPACE'
- ✔ Writing 'mytools.Rproj'
- ✔ Adding '.Rproj.user' to '.gitignore'
- ✔ Adding '^mytools\\.Rproj$', '^\\.Rproj\\.user$' to '.Rbuildignore'
- ✔ Opening new project 'mytools' in RStudio
-
-Note that this will open a new project (`mytools`) and a new session in RStudio server.
-
-The `create_package` function created a top-level directory structure, including a number of critical files under the [standard R package structure](http://cran.r-project.org/doc/manuals/r-release/R-exts.html#Package-structure). The most important of which is the `DESCRIPTION` file, which provides metadata about your package. Edit the `DESCRIPTION` file to provide reasonable values for each of the fields,
-including your own contact information.
-
-Information about choosing a LICENSE is provided in the [Extending R](http://cran.r-project.org/doc/manuals/r-release/R-exts.html#Licensing) documentation.
-The DESCRIPTION file expects the license to be chose from a predefined list, but
-you can use it's various utility methods for setting a specific license file, such
-as the `Apacxhe 2` license:
-
-```{r, eval=FALSE}
-usethis::use_apache_license()
-```
-
- ✔ Setting License field in DESCRIPTION to 'Apache License (>= 2.0)'
- ✔ Writing 'LICENSE.md'
- ✔ Adding '^LICENSE\\.md$' to '.Rbuildignore'
-
-Once your license has been chosen, and you've edited your DESCRIPTION file with your contact information, a title, and a description, it will look like this:
-
-```{r, eval=FALSE}
-Package: mytools
-Title: Utility Functions Created by Matt Jones
-Version: 0.1
-Authors@R: "Matthew Jones [aut, cre]"
-Description: Package mytools contains a suite of utility functions useful whenever I need stuff to get done.
-Depends: R (>= 3.5.0)
-License: Apache License (>= 2.0)
-LazyData: true
-```
-
-
-### Add your code
-
-The skeleton package created contains a directory `R` which should contain your source files. Add your functions and classes in files to this directory, attempting to choose names that don't conflict with existing packages. For example, you might add a file `cutsom_theme` that contains a function `custom_theme()` that you might want to reuse. The `usethis::use_r()` function will help set up you files in the right places. For example, running:
-
-```{r eval=FALSE}
-usethis::use_r("custom_theme")
-```
-
- ● Modify 'R/custom_theme'
-
-creates the file `R/custom_theme`, which you can then modify to add the implementation fo the following function from the functions lesson:
-
-```{r eval=FALSE}
-custom_theme <- function(base_size = 9) {
- ggplot2::theme(
- axis.ticks = ggplot2::element_blank(),
- text = ggplot2::element_text(family = 'Helvetica', color = 'gray30', size = base_size),
- plot.title = ggplot2::element_text(size = ggplot2::rel(1.25), hjust = 0.5, face = 'bold'),
- panel.background = ggplot2::element_blank(),
- legend.position = 'right',
- panel.border = ggplot2::element_blank(),
- panel.grid.minor = ggplot2::element_blank(),
- panel.grid.major = ggplot2::element_line(colour = 'grey90', size = .25),
- legend.key = ggplot2::element_rect(colour = NA, fill = NA),
- axis.line = ggplot2::element_blank()
- )
-}
-
-```
-
-If your R code depends on functions from another package, then you must declare so
-in the `Imports` list in the `DESCRIPTION` file for your package. In our example
-above, we depend on the `ggplot2` package, and so we need to list it as a dependency.
-Once again, `usethis` provides a handy helper method:
-
-```{r eval=FALSE}
-usethis::use_package("ggplot2")
-```
-
- ✔ Adding 'ggplot2' to Imports field in DESCRIPTION
- ● Refer to functions with `devtools::fun()`
-
-### Add documentation
-
-You should provide documentation for each of your functions and classes. This is done in the `roxygen2` approach of providing embedded comments in the source code files, which are in turn converted into manual pages and other R documentation artifacts. Be sure to define the overall purpose of the function, and each of its parameters.
-
-```{r}
-#' A function set a custom ggplot theme.
-#'
-#' This function sets ggplot theme elements that I like, with the ability to change
-#' the base size of the text.
-#'
-#' @param base_size Base size of plot text
-#'
-#' @keywords plotting
-#'
-#' @export
-#'
-#' @examples
-#' library(ggplot2)
-#'
-#' ggplot(iris, aes(Sepal.Length, Sepal.Width)) +
-#' geom_point() +
-#' custom_theme(base_size = 10)
-#'
-custom_theme <- function(base_size = 9) {
- ggplot2::theme(
- axis.ticks = ggplot2::element_blank(),
- text = ggplot2::element_text(family = 'Helvetica', color = 'gray30', size = base_size),
- plot.title = ggplot2::element_text(size = ggplot2::rel(1.25), hjust = 0.5, face = 'bold'),
- panel.background = ggplot2::element_blank(),
- legend.position = 'right',
- panel.border = ggplot2::element_blank(),
- panel.grid.minor = ggplot2::element_blank(),
- panel.grid.major = ggplot2::element_line(colour = 'grey90', size = .25),
- legend.key = ggplot2::element_rect(colour = NA, fill = NA),
- axis.line = ggplot2::element_blank()
- )
-}
-
-```
-
-Once your files are documented, you can then process the documentation using the `document()` function to generate the appropriate .Rd files that your package needs.
-
-```{r, eval = F}
-devtools::document()
-```
-
- Updating mytools documentation
- Updating roxygen version in /Users/jones/development/mytools/DESCRIPTION
- Writing NAMESPACE
- Loading mytools
- Writing NAMESPACE
- Writing custom_theme.Rd
-
-That's really it. You now have a package that you can `check()` and `install()` and `release()`. See below for these helper utilities.
-
-### Test your package
-
-You can test your code using the `tetsthat` testing framework. The `ussethis::use_testthat()`
-function will set up your package for testing, and then you can use the `use_test()` function
-to setup individual test files. For example, in the functions lesson we created some tests for our `fahr_to_celsius` functions but ran them line by line in the console.
-
-First, lets add that function to our package. Run the `use_r` function in the console:
-
-```{r, eval = FALSE}
-usethis::use_r("fahr_to_celsius")
-```
-
-Then copy the function and documentation into the R script that opens and save the file.
-
-```{r}
-#' Convert temperature data from Fahrenheit to Celsius
-#'
-#' @param fahr Temperature data in degrees Fahrenheit to be converted
-#' @return temperature value in degrees Celsius
-#' @keywords conversion
-#' @export
-#' @examples
-#' fahr_to_celsius(32)
-#' fahr_to_celsius(c(32, 212, 72))
-fahr_to_celsius <- function(fahr) {
- celsius <- (fahr-32)*5/9
- return(celsius)
-}
-```
-
-Now, set up your package for testing:
-
-```{r eval = FALSE}
-usethis::use_testthat()
-```
- ✔ Adding 'testthat' to Suggests field in DESCRIPTION
- ✔ Creating 'tests/testthat/'
- ✔ Writing 'tests/testthat.R'
-
-
-Then write a test for `fahr_to_celsius`:
-
-```{r eval = FALSE}
-usethis::use_test("fahr_to_celsius")
-```
- ✔ Writing 'tests/testthat/test-fahr_to_celsius.R'
- ● Modify 'tests/testthat/test-fahr_to_celsius.R'
-
-You can now add tests to the `test-fahr_to_celsius.R`, and you can run all of the
-tests using `devtools::test()`. For example, if you add a test to the `test-fahr_to_celsius.R` file:
-
-```{r eval=FALSE}
-test_that("fahr_to_celsius works", {
- expect_equal(fahr_to_celsius(32), 0)
- expect_equal(fahr_to_celsius(212), 100)
-})
-
-```
-
-Then you can run the tests to be sure all of your functions are working using `devtools::test()`:
-
-```{r eval=FALSE}
-devtools::test()
-```
-
- Loading mytools
- Testing mytools
- ✔ | OK F W S | Context
- ✔ | 2 | test-fahr_to_celsius [0.1 s]
-
- ══ Results ════════════════════════════════════════════════════════════════════════════════════════════════════════════════════════════════════
- Duration: 0.1 s
-
- OK: 2
- Failed: 0
- Warnings: 0
- Skipped: 0
-
-Yay, all tests passed!
-
-### Checking and installing your package
-
-Now that your package is built, you can check it for consistency and completeness using `check()`, and then you can install it locally using `install()`, which needs to be run from the parent directory of your module.
-
-```{r, eval = FALSE}
-devtools::check()
-devtools::install()
-```
-
-Your package is now available for use in your local environment.
-
-### Sharing and releasing your package
-
-The simplest way to share your package with others is to upload it to a [GitHub repository](https://github.com), which allows others to install your package using the `install_github('mytools','github_username')` function from `devtools`.
-
-If your package might be broadly useful, also consider releasing it to CRAN, using the `release()` method from `devtools(). Releasing a package to CRAN requires a significant amoutn of work to ensure it follows the standards set by the R community, but it is entirely tractable and a valuable contribution to the science community. If you are considering releasing a package more broadly, you may find that the supportive community at [ROpenSci](https://ropensci.org) provides incredible help and valuable feeback through their onboarding process.
-
-#### Challenge {- .exercise}
-
-Add the other temperature conversion functions with full documentation to your package, write tests to ensure the functions work properly, and then
-`document()`, `check()`, and `install()` the new version of the package. Don't forget to update the version number before you install!
-
-### More reading
-
-- Hadley Wickham's awesome book: [R Packages](http://r-pkgs.had.co.nz/)
-- Thomas Westlake's blog [Writing an R package from scratch](https://r-mageddon.netlify.com/post/writing-an-r-package-from-scratch/)
-
-
-
diff --git a/materials/sections/r-intro-rstudio-git-setup-motivation.Rmd b/materials/sections/r-intro-rstudio-git-setup-motivation.Rmd
deleted file mode 100644
index 2f455580..00000000
--- a/materials/sections/r-intro-rstudio-git-setup-motivation.Rmd
+++ /dev/null
@@ -1,302 +0,0 @@
-## RStudio Setup
-
-### Learning Objectives
-
-In this lesson, you will learn:
-
-- Creating an R project and how to organize your work in a project
-- How to make sure your local RStudio environment is set up for analysis*
-- How to set up Git and GitHub
-
-### Logging into the RStudio server
-
-To prevent us from spending most of this lesson troubleshooting the myriad of issues that can arise when setting up the R, RStudio, and git environments, we have chosen to have everyone work on a remote server with all of the software you need installed. We will be using a special kind of RStudio just for servers called RStudio Server. If you have never worked on a remote server before, you can think of it like working on a different computer via the internet. Note that the server has no knowledge of the files on your local filesystem, but it is easy to transfer files from the server to your local computer, and vice-versa, using the RStudio server interface.
-
-Here are the instructions for logging in and getting set up:
-
-#### Server Setup {.unnumbered .setup}
-
-You should have received an email prompting you to change your password for your server account. If you did not, please let us know and someone will help you.
-
-If you were able to successfully change your password, you can log in at:
-
-### Why use an R project?
-
-In this workshop, we are going to be using R project to organize our work. An R project is tied to a directory on your local computer, and makes organizing your work and collaborating with others easier.
-
-**The Big Idea:** using an R project is a reproducible research best practice because it bundles all your work within a *working directory*. Consider your current data analysis workflow. Where do you import you data? Where do you clean and wrangle it? Where do you create graphs, and ultimately, a final report? Are you going back and forth between multiple software tools like Microsoft Excel, JMP, and Google Docs? An R project and the tools in R that we will talk about today will consolidate this process because it can all be done (and updated) in using one software tool, RStudio, and within one R project.
-
-We are going to be doing nearly all of the work in this course in one R project.
-
-Our version of RStudio Server allows you to share projects with others. Sharing your project with the instructors of the course will allow for them to jump into your session and type along with you, should you encounter an error you cannot fix.
-
-#### Creating your project {.unnumbered .setup}
-
-In your RStudio server session, follow these steps to set up your R project:
-
-- In the "File" menu, select "New Project"
-- Click "New Directory"
-- Click "New Project"
-- Under "Directory name" type: `training_{USERNAME}`, eg: `training_vargas`
-- Leave "Create Project as subdirectory of:" set to `~`
-- Click "Create Project"
-
-Your RStudio should open your project automatically after creating it. One way to check this is by looking at the top right corner and checking for the project name.
-
-#### Sharing your project {.unnumbered .setup}
-
-To share your project with the instructor team, locate the "project switcher" dropdown menu in the upper right of your RStudio window. This dropdown has the name of your project (eg: `training_vargas`), and a dropdown arrow. Click the dropdown menu, then "Share Project." When the dialog box pops up, add the following usernames to your project:
-
-- dolinh
-- jclark
-- virlar-knight
-- vargas-pouslen
-
-Once those names show up in the list, click "OK".
-
-
-#### Preparing to work in RStudio
-
-
-
-The default RStudio setup has a few panes that you will use. Here they are with their default locations:
-
-- Console (entire left)
-- Environment/History (tabbed in upper right)
-- Files/Plots/Packages/Help (tabbed in lower right)
-
-You can change the default location of the panes, among many other things: [Customizing RStudio](https://support.rstudio.com/hc/en-us/articles/200549016-Customizing-RStudio).
-
-One key question to ask whenever we open up RStudio is "where am I?" Because we like to work in RStudio projects, often this question is synonymous with "what project am I in?"
-
-There are two places that can indicate what project we are in. The first is the project switcher menu in the upper right hand corner of your RStudio window. The second is the working directory path, in the top bar of your console. Note that by default, your working directory is set to the top level of your R project directory unless you change it using the `setwd()` function.
-
-
-
-
-
-### Understand how to use paths and working directories
-
-
-
-Now that we have your project created (and notice we know it's an R Project because we see a `.Rproj` file in our Files pane), let's learn how to move in a project. We do this using paths.
-
-There are two types of paths in computing: **absolute paths** and **relative paths**.
-
-An absolute path always starts with the root of your file system and locates files from there. The absolute path to my project directory is: `/home/vargas-poulsen/training_vargas`
-
-Relative paths start from some location in your file system that is below the root. Relative paths are combined with the path of that location to locate files on your system. R (and some other languages like MATLAB) refer to the location where the relative path starts as our *working directory*.
-
-RStudio projects automatically set the working directory to the directory of the project. This means that you can reference files from within the project without worrying about where the project directory itself is. If I want to read in a file from the `data` directory within my project, I can simply type `read.csv("data/samples.csv")` as opposed to `read.csv("/home/vargas-poulsen/training_vargas/data/samples.csv")`
-
-This is not only convenient for you, but also when working collaboratively. We will talk more about this later, but if Jeanette makes a copy of my R project that I have published on GitHub, and I am using relative paths, he can run my code exactly as I have written it, without going back and changing `"/home/vargas-poulsen/training_vargas/data/samples.csv"` to `"/home/jclark/training_clark/data/samples.csv"`
-
-Note that once you start working in projects you should basically never need to run the `setwd()` command. If you are in the habit of doing this, stop and take a look at where and why you do it. Could leveraging the working directory concept of R projects eliminate this need? Almost definitely!
-
-Similarly, think about how you work with absolute paths. Could you leverage the working directory of your R project to replace these with relative paths and make your code more portable? Probably!
-
-### Organizing your project
-
-When starting a new research project, one of the first things I do is create an R project for it (just like we have here!). The next step is to then populate that project with relevant directories. There are many tools out there that can do this automatically. Some examples are [`rrtools`](https://github.com/benmarwick/rrtools) or `usethis::create_package()`. The goal is to organize your project so that it is a compendium of your research. This means that the project has all of the digital parts needed to replicate your analysis, like code, figures, the manuscript, and data access.
-
-There are lots of good examples out there of research compendium. Here is one from a friend of NCEAS, Carl Boettiger, which he put together for a paper he wrote.
-
-
-
-The complexity of this project reflects years of work. Perhaps more representative of the situation we are in at the start of our course is a project that looks like this one, which we have just started at NCEAS.
-
-
-
-Currently, the only file in your project is your `.Rproj` file. Let's add some directories and start a file folder structure. Some common directories are:
-
-- `data`: where we store our data (often contains subdirectories for raw, processed, and metadata data)
-
-- `R`: contains scripts for cleaning or wrangling, etc. (some find this name misleading if their work has other scripts beyond the R programming language, in which case they call this directory `scripts`)
-
-- `plots` or `figs`: generated plots, graphs, and figures
-
-- `doc`: summaries or reports of analysis or other relevant project information
-
-Directory organization will vary from project to project, but the ultimate goal is to create a well organized project for both reproducibility and collaboration.
-
-
-### Summary
-
-- organize your research into projects using R projects
-- use R project working directories instead of `setwd()`
-- use relative paths from those working directories, not absolute paths
-- structure your R project as a compendium
-
-
-### Setting up git
-
-Before using git, you need to tell it who you are, also known as setting the global options. The only way to do this is through the command line. Newer versions of RStudio have a nice feature where you can open a terminal window in your RStudio session. Do this by selecting Tools -> Terminal -> New Terminal.
-
-A terminal tab should now be open where your console usually is.
-
-To set the global options, type the following into the command prompt, with your actual name, and press enter:
-
-```{sh git-name, eval=FALSE}
-git config --global user.name "Matt Jones"
-```
-
-Note that if it ran successfully, it will look like nothing happened. We will check at the end to makre sure it worked.
-
-Next, enter the following line, with the email address you used when you created your account on github.com:
-
-```{sh git-email, eval=FALSE}
-git config --global user.email "gitcode@magisa.org"
-```
-
-Note that these lines need to be run one at a time.
-
-Next, we will set our credentials to not time out for a very long time. This is related to the way that our server operating system handles credentials - not doing this will make your PAT (which we will set up soon) expire immediately on the system, even though it is actually valid for a month.
-
-```{sh git-cred, eval=FALSE}
-git config --global credential.helper 'cache --timeout=10000000'
-```
-
-Lastly, we will set up two more configurations to make sure we have everything in place for out `git` lesson tomorrow. We will dive deeper into these concepts tomorrow. So for now, all you need to know us that we are letting git know how we want git to weave in the different versions of our work.
-
-```{r pull conf, eval=FALSE}
-git config pull.rebase false
-```
-
-And then, we will set that the default branch of our work is a branch called `main`. And again, we will go over these in more details concepts tomorrow.
-```{r def branch main, eval=FALSE}
-git config --global init.defaultBranch main
-```
-
-Finally, check to make sure everything looks correct by entering this command, which will return the options that you have set.
-
-```{sh git-list, eval=FALSE}
-git config --global --list
-```
-
-### GitHub Authentication
-
-GitHub recently deprecated password authentication for accessing repositories, so we need to set up a secure way to authenticate. The book [Happy git with R](https://happygitwithr.com/credential-caching.html) has a wealth of information related to working with git in R, and these instructions are based off of section 10.1.
-
-We will be using a PAT (Personal Access Token) in this course, because it is easy to set up. For better security and long term use, we recommend taking the extra steps to set up SSH keys.
-
-Steps:
-
-1. Run `usethis::create_github_token()` in the console
-2. In the browser window that pops up, scroll to the bottom and click "generate token." You may need to log into GitHub first.
-3. Copy the token from the green box on the next page
-4. Back in RStudio, run `credentials::set_github_pat()`
-5. Paste your token into the dialog box that pops up.
-
-
-
-### Setting up the R environment on your local computer
-
-##### R Version {.unnumbered}
-
-We will use R version 4.0.5, which you can download and install from [CRAN](https://cran.rstudio.com). To check your version, run this in your RStudio console:
-
-```{r r-version, eval=FALSE}
-R.version$version.string
-```
-
-If you have R version 4.0.0 that will likely work fine as well.
-
-##### RStudio Version {.unnumbered}
-
-We will be using RStudio version 1.4 or later, which you can download and install [here](https://www.rstudio.com/products/rstudio/download/) To check your RStudio version, run the following in your RStudio console:
-
-```{r rstudio-version, eval=FALSE}
-RStudio.Version()$version
-```
-
-If the output of this does not say `1.4` or higher, you should update your RStudio. Do this by selecting Help -\> Check for Updates and follow the prompts.
-
-##### Package installation {.unnumbered}
-
-Run the following lines to check that all of the packages we need for the training are installed on your computer.
-
-```{r package-install, eval = FALSE}
-packages <- c("dplyr", "tidyr", "readr", "devtools", "usethis", "roxygen2", "leaflet", "ggplot2", "DT", "scales", "shiny", "sf", "ggmap", "broom", "captioner", "MASS")
-
-for (package in packages) {
-
- if (!(package %in% installed.packages())) { install.packages(package) }
-
- }
-
-rm(packages) # remove variable from workspace
-
-# Now upgrade any out-of-date packages
-update.packages(ask=FALSE)
-```
-
-If you haven't installed all of the packages, this will automatically start installing them. If they are installed, it won't do anything.
-
-Next, create a new R Markdown (File -\> New File -\> R Markdown). If you have never made an R Markdown document before, a dialog box will pop up asking if you wish to install the required packages. Click yes.
-
-At this point, RStudio and R should be all set up.
-
-##### Setting up git locally {.unnumbered}
-
-If you haven't downloaded git already, you can do so [here](https://git-scm.com/).
-
-If you haven't already, go to [github.com](http://github.com) and create an account.
-
-Then you can follow the instructions that we used above to set your email address and user name.
-
-##### Note for Windows Users {.unnumbered}
-
-If you get "command not found" (or similar) when you try these steps through the RStudio terminal tab, you may need to set the type of terminal that gets launched by RStudio. Under some git install scenarios, the git executable may not be available to the default terminal type. Follow the instructions on the RStudio site for [Windows specific terminal options](https://support.rstudio.com/hc/en-us/articles/115010737148-Using-the-RStudio-Terminal#appendix). In particular, you should choose "New Terminals open with Git Bash" in the Terminal options (`Tools->Global Options->Terminal`).
-
-In addition, some versions of windows have difficulty with the command line if you are using an account name with spaces in it (such as "Matt Jones", rather than something like "mbjones"). You may need to use an account name without spaces.
-
-##### Updating a previous R installation {.unnumbered}
-
-**This is useful for users who already have R with some packages installed and need to upgrade R, but don't want to lose packages.** If you have never installed R or any R packages before, you can skip this section.
-
-If you already have R installed, but need to update, and don't want to lose your packages, these two R functions can help you. The first will save all of your packages to a file. The second loads the packages from the file and installs packages that are missing.
-
-Save this script to a file (e.g. `package_update.R`).
-
-```{r, eval = F}
-#' Save R packages to a file. Useful when updating R version
-#'
-#' @param path path to rda file to save packages to. eg: installed_old.rda
-save_packages <- function(path){
-
- tmp <- installed.packages()
- installedpkgs <- as.vector(tmp[is.na(tmp[,"Priority"]), 1])
- save(installedpkgs, file = path)
-}
-
-#' Update packages from a file. Useful when updating R version
-#'
-#' @param path path to rda file where packages were saved
-update_packages <- function(path){
- tmp <- new.env()
- installedpkgs <- load(file = path, envir = tmp)
- installedpkgs <- tmp[[ls(tmp)[1]]]
- tmp <- installed.packages()
-
- installedpkgs.new <- as.vector(tmp[is.na(tmp[,"Priority"]), 1])
- missing <- setdiff(installedpkgs, installedpkgs.new)
- install.packages(missing)
- update.packages(ask=FALSE)
-}
-```
-
-Source the file that you saved above (eg: `source(package_update.R)`). Then, run the `save_packages` function.
-
-```{r, eval = F}
-save_packages("installed.rda")
-```
-
-Then quit R, go to [CRAN](https://cran.rstudio.com), and install the latest version of R.
-
-Source the R script that you saved above again (eg: `source(package_update.R)`), and then run:
-
-```{r, eval = F}
-update_packages("installed.rda")
-```
-
-This should install all of your R packages that you had before you upgraded.
diff --git a/materials/sections/survey-workflows.Rmd b/materials/sections/survey-workflows.Rmd
deleted file mode 100644
index a0e45b1a..00000000
--- a/materials/sections/survey-workflows.Rmd
+++ /dev/null
@@ -1,285 +0,0 @@
-## Reproducible Survey Workflows
-
-### Learning Objectives
-
-- Overview of survey tools
-- Generating a reproducible survey report with Qualtrics
-
-
-### Introduction
-
-Surveys and questionnaires are commonly used research methods within social science and other fields. For example, understanding regional and national population demographics, income, and education as part of the [National Census](https://www.census.gov/en.html) activity, assessing audience perspectives on specific topics of research interest (e.g. the work by Tenopir and colleagues on [Data Sharing by Scientists](https://journals.plos.org/plosone/article?id=10.1371/journal.pone.0021101)), evaluation of learning deliverables and outcomes, and consumer feedback on new and upcoming products. These are distinct from the use of the term survey within natural sciences, which might include geographical surveys ("the making of measurement in the field from which maps are drawn"), ecological surveys ("the process whereby a proposed development site is assess to establish any environmental impact the development may have") or biodiversity surveys ("provide detailed information about biodiversity and community structure") among others.
-
-Although surveys can be conducted on paper or verbally, here we focus on surveys done via software tools. Needs will vary according to the nature of the research being undertaken. However, there is fundamental functionality that survey software should provide including:
-
-1. The ability to create and customize questions
-1. The ability to include different types of questions
-1. The ability to distribute the survey and manage response collection
-1. The ability to collect, summarize, and (securely) store response data
-
-More advanced features can include:
-
-1. **Visual design and templates** - custom design might include institutional branding or aesthetic elements. Templates allow you to save these designs and apply to other surveys
-1. **Question piping** - piping inserts answers from previous questions into upcoming questions and can personalize the survey experience for users
-1. **Survey logic** - with question logic and skip logic you can control the inclusion / exclusion of questions based on previous responses
-1. **Randomization** - the ability to randomize the presentation of questions within (blocks of) the survey
-1. **Branching** - this allows for different users to take different paths through the survey. Similar to question logic but at a bigger scale
-1. **Language support** - automated translation or multi-language presentation support
-1. **Shared administration** - enables collaboration on the survey and response analysis
-1. **Survey export** - ability to download (export) the survey instrument
-1. **Reports** - survey response visualization and reporting tools
-1. **Institutional IRB approved** - institutional IRB policy may require certain software be used for research purposes
-
-Commonly used survey software within academic (vs market) research include Qualtrics, Survey Monkey and Google Forms. Both qualtrics and survey monkey are licensed (with limited functionality available at no cost) and google forms is free.
-
-
-
-### Building workflows using Qualtrics
-
-In this lesson we will use the [`qualtRics`](https://github.com/ropensci/qualtRics) package to reproducible access some survey results set up for this course.
-
-#### Survey Instrument
-
-The survey is very short, only four questions. The first question is on it's own page and is a consent question, after a couple of short paragraphs describing what the survey is, it's purpose, how long it will take to complete, and who is conducting it. This type of information is required if the survey is governed by an IRB, and the content will depend on the type of research being conducted. In this case, this survey is not for research purposes, and thus is not governed by IRB, but we still include this information as it conforms to the [Belmont Principles](https://www.hhs.gov/ohrp/regulations-and-policy/belmont-report/read-the-belmont-report/index.html#xinform). The Belmont Principles identify the basic ethical principles that should underlie research involving human subjects.
-
-
-
-The three main questions of the survey have three types of responses: a multiple choice answer, a multiple choice answer which also includes an "other" write in option, and a free text answer. We'll use the results of this survey, which was sent out to NCEAS staff to fill out, to learn about how to create a reproducible survey report.
-
-
-
-
-
-First, open a new RMarkdown document and add a chunk to load the libraries we'll need for this lesson:
-
-```{r, eval = FALSE}
-library(qualtRics)
-library(dplyr)
-library(tidyr)
-library(knitr)
-library(ggplot2)
-library(kableExtra)
-```
-
-Next, we need to set the API credentials. This function modifies the `.Renviron` file to set your API key and base URL so that you can access Qualtrics programmatically.
-
-The API key is as good as a password, so care should be taken to not share it publicly. For example, you would never want to save it in a script. The function below is the rare exception of code that should be run in the console and not saved. It works in a way that you only need to run it once, unless you are working on a new computer or your credentials changed. Note that in this book, we have not shared the actual API key, for the reasons outlined above. You should have an e-mail with the API key in it. Copy and paste it as a string to the `api_key` argument in the function below:
-
-```{r, eval = FALSE}
-qualtrics_api_credentials(api_key = "", base_url = "ucsb.co1.qualtrics.com", install = TRUE)
-```
-
-#### Aside {.aside -}
-
-The .Renviron file is a special user controlled file that can create environment variables. Every time you open Rstudio, the variables in your environment file are loaded as...environment variables! Environment variables are named values that are accessible by your R process. They will not show up in your environment pane, but you can get a list of all of them using `Sys.getenv()`. Many are system defaults.
-
-#### {-}
-
-To view or edit your `.Renviron` file, you can use `usethis::edit_r_environ()`.
-
-To get a list of all the surveys in your Qualtrics instance, use the `all_surveys` function.
-
-```{r, eval = FALSE}
-surveys <- all_surveys()
-kable(surveys) %>%
- kable_styling()
-```
-
-This function returns a list of surveys, in this case only one, and information about each, including an identifier and it's name. We'll need that identifier later, so let's go ahead and extract it using base R from the data frame.
-
-```{r, eval = FALSE}
-i <- which(surveys$name == "Survey for Data Science Training")
-id <- surveys$id[i]
-```
-
-You can retrieve a list of the questions the survey asked using the `survey_questions` function and the survey `id`.
-
-```{r, eval = FALSE}
-questions <- survey_questions(id)
-kable(questions) %>%
- kable_styling()
-```
-
-This returns a `data.frame` with one row per question with columns for question id, question name, question text, and whether the question was required. This is helpful to have as a reference for when you are looking at the full survey results.
-
-To get the full survey results, run `fetch_survey` with the survey id.
-
-```{r, eval = FALSE}
-survey_results <- fetch_survey(id)
-```
-
-The survey results table has tons of information in it, not all of which will be relevant depending on your survey. The table has identifying information for the respondents (eg: `ResponseID`, `IPaddress`, `RecipientEmail`, `RecipientFirstName`, etc), much of which will be empty for this survey since it is anonymous. It also has information about the process of taking the survey, such as the `StartDate`, `EndDate`, `Progress`, and `Duration`. Finally, there are the answers to the questions asked, with columns labeled according to the `qname` column in the questions table (eg: Q1, Q2, Q3). Depending on the type of question, some questions might have multiple columns associated with them. We'll have a look at this more closely in a later example.
-
-#### Question 2 {-}
-
-Let's look at the responses to the second question in the survey, "How long have you been programming?" Remember, the first question was the consent question.
-
-We'll use the `dplyr` and `tidyr` tools we learned earlier to extract the information. Here are the steps:
-
-- `select` the column we want (`Q1`)
-- `group_by` and `summarize` the values
-
-```{r, eval = FALSE}
-q2 <- survey_results %>%
- select(Q2) %>%
- group_by(Q2) %>%
- summarise(n = n())
-```
-
-We can show these results in a table using the `kable` function from the `knitr` package:
-
-```{r, eval = FALSE}
-kable(q2, col.names = c("How long have you been programming?",
- "Number of responses")) %>%
- kable_styling()
-```
-
-#### Question 3
-
-For question 3, we'll use a similar workflow. For this question, however there are two columns containing survey answers. One contains the answers from the controlled vocabulary, the other contains any free text answers users entered.
-
-To present this information, we'll first show the results of the controlled answers as a plot. Below the plot, we'll include a table showing all of the free text answers for the "other" option.
-
-```{r, eval = FALSE}
-q3 <- survey_results %>%
- select(Q3) %>%
- group_by(Q3) %>%
- summarise(n = n())
-```
-
-```{r, eval = FALSE}
-ggplot(data = q3, mapping = aes(x = Q3, y = n)) +
- geom_col() +
- labs(x = "What language do you currently use most frequently?", y = "Number of reponses") +
- theme_minimal()
-```
-
-Now we'll extract the free text responses:
-
-```{r, eval = FALSE}
-q3_text <- survey_results %>%
- select(Q3_7_TEXT) %>%
- drop_na()
-
-kable(q3_text, col.names = c("Other responses to 'What language do you currently use mose frequently?'")) %>%
- kable_styling()
-```
-
-#### Question 4
-
-The last question is just a free text question, so we can just display the results as is.
-
-```{r, eval = FALSE}
-q4 <- survey_results %>%
- select(Q4) %>%
- rename(`What data science tool or language are you most excited to learn next?` = Q4) %>%
- drop_na()
-
-kable(q4, col.names = "What data science tool or language are you most excited to learn next?") %>%
- kable_styling()
-```
-
-
-### Other survey tools
-
-#### Google forms {-}
-
-Google forms can be a great way to set up surveys, and it is very easy to interact with the results using R. The benefits of using google forms are a simple interface and easy sharing between collaborators, especially when writing the survey instrument.
-
-The downside is that google forms has far fewer features than Qualtrics in terms of survey flow and appearance.
-
-To show how we can link R into our survey workflows, I've set up a simple example survey [here](https://docs.google.com/forms/d/1Yh3IxygzuLXzJvTHl-lskMy7YrQgmeWgr2bEw5gwdIM/edit?usp=sharing).
-
-I've set up the results so that they are in a new spreadsheet [here:](https://docs.google.com/spreadsheets/d/1CSG__ejXQNZdwXc1QK8dKouxphP520bjUOnZ5SzOVP8/edit?resourcekey#gid=1527662370). To access them, we will use the `googlesheets4` package.
-
-First, open up a new R script and load the `googlesheets4` library:
-
-```{r}
-library(googlesheets4)
-```
-
-Next, we can read the sheet in using the same URL that you would use to share the sheet with someone else. Right now, this sheet is public
-
-```{r, echo = FALSE}
-gs4_deauth()
-```
-
-
-```{r}
-responses <- read_sheet("https://docs.google.com/spreadsheets/d/1CSG__ejXQNZdwXc1QK8dKouxphP520bjUOnZ5SzOVP8/edit?usp=sharing")
-```
-
-The first time you run this, you should get a popup window in your web browser asking you to confirm that you want to provide access to your google sheets via the tidyverse (googlesheets) package.
-
-My dialog box looked like this:
-
-
-
-Make sure you click the third check box enabling the Tidyverse API to see, edit, create, and delete your sheets. Note that you will have to tell it to do any of these actions via the R code you write.
-
-When you come back to your R environment, you should have a data frame containing the data in your sheet! Let's take a quick look at the structure of that sheet.
-
-```{r}
-glimpse(responses)
-```
-
-So, now that we have the data in a standard R `data.frame`, we can easily summarize it and plot results. By default, the column names in the sheet are the long fully descriptive questions that were asked, which can be hard to type. We can save those questions into a vector for later reference, like when we want to use the question text for plot titles.
-
-```{r}
-questions <- colnames(responses)[2:5]
-glimpse(questions)
-```
-
-We can make the responses data frame more compact by renaming the columns of the vector with short numbered names of the form `Q1`. Note that, by using a sequence, this should work for sheets from just a few columns to many hundreds of columns, and provides a consistent question naming convention.
-
-```{r}
-names(questions) <- paste0("Q", seq(1:4))
-colnames(responses) <- c("Timestamp", names(questions))
-glimpse(responses)
-```
-
-Now that we've renamed our columns, let's summarize the responses for the first question. We can use the same pattern that we usually do to split the data from Q1 into groups, then summarize it by counting the number of records in each group, and then merge the count of each group back together into a summarized data frame. We can then plot the Q1 results using `ggplot`:
-
-```{r}
-q1 <- responses %>%
- select(Q1) %>%
- group_by(Q1) %>%
- summarise(n = n())
-
-ggplot(data = q1, mapping = aes(x = Q1, y = n)) +
- geom_col() +
- labs(x = questions[1],
- y = "Number of reponses",
- title = "To what degree did the course meet expectations?") +
- theme_minimal()
-```
-
-
-
-##### Bypassing authentication for public sheets {-}
-
-If you don't want to go through a little interactive dialog every time you read in a sheet, and your sheet is public, you can run the function `gs4_deauth()` to access the sheet as a public user. This is helpful for cases when you want to run your code non-interactively. This is actually how I set it up for this book to build!
-
-##### Challenge {- .exercise}
-
-Now that you have some background in accessing survey data from common tools, let's do a quick exercise with Google Sheets. First, create a google sheet with the following columns that reflect a hypothetical survey result:
-
-- Timestamp
-- Q1: How much did your proficiency with survey tools in R change? 1 = None, 2 = A little, 3 = A lot
-- Q2: How many years or partial years had you used R prior to this course?
-- Q3: How many years or partial years had you used statistics before this course?
-
-Next populate the spreadhsheet with 5 to 10 rows of sample data that you make up. Now that you have the Google sheet in place, copy its URL and use it to do the following in R:
-
-1. Load the google sheet into an R data.frame using the `googlesheets` package
-2. Save the column headers as a vector of questions
-3. Rename the question columns with short, consistent names
-4. Summarize and plot the results for Q1 as a bar chart.
-
-#### Survey Monkey {-}
-
-Similar to Qualtrics and qualtRics, there is an open source R package for working with data in Survey Monkey: [Rmonkey](https://github.com/cloudyr/Rmonkey). However, the last updates were made 5 years ago, an eternity in the software world, so it may or may not still function as intended.
-
-There are also commercial options available. For example, [cdata](https://www.cdata.com/kb/tech/surveymonkey-jdbc-r.rst) have a driver and R package that enable access to an analysis of Survey Monkey data through R.
-
-
diff --git a/materials/sections/text-analysis.Rmd b/materials/sections/text-analysis.Rmd
deleted file mode 100644
index 9f84d24d..00000000
--- a/materials/sections/text-analysis.Rmd
+++ /dev/null
@@ -1,404 +0,0 @@
----
-author: "Jeanette Clark"
----
-
-## Extracting Data for Text Analysis
-
-### Learning Objectives
-
-- What a token is and how they are used
-- How to use stop words
-- How to customize stop words
-- How to give structure to unstructured text
-
-### Introduction
-
-Much of the information covered in this chapter is based on [Text Mining with R: A Tidy Approach](https://www.tidytextmining.com/index.html) by Julia Silge and David Robinson. This is a great book if you want to go deeper into text analysis.
-
-Text mining is the process by which unstructured text is transformed into a structured format to prepare it for analysis. This can range from the simple example we show in this lesson, to much more complicated processes such as using OCR (optical character recognition) to scan and extract text from pdfs, or web scraping.
-
-Once text is in a structured format, analysis can be performed on it. The inherent benefit of quantitative text analysis is that it is highly scalable. With the right computational techniques, massive quantities of text can be mined and analyzed many, many orders of magnitude faster than it would take a human to do the same task. The downside, is that human language is inherently nuanced, and computers (as you may have noticed) think very differently than we do. In order for an analysis to capture this nuance, the tools and techniques for text analysis need to be set up with care, especially when the analysis becomes more complex.
-
-There are a number of different types of text analysis. In this lesson we will show some simple examples of two: word frequency, and sentiment analysis.
-
-#### Setup {.setup -}
-
-First we'll load the libraries we need for this lesson:
-
-```{r, warning = FALSE, message = FALSE}
-library(dplyr)
-library(tibble)
-library(readr)
-library(tidytext)
-library(wordcloud)
-library(reshape2)
-```
-
-
-Load the survey data back in using the code chunks below:
-
-```{r, echo = F}
-survey_raw <- read_csv("../data/survey_data.csv", show_col_types = FALSE)
-
-events <- read_csv("../data/events.csv", show_col_types = FALSE)
-```
-
-```{r, eval = F}
-survey_raw <- read_csv("https://dev.nceas.ucsb.edu/knb/d1/mn/v2/object/urn%3Auuid%3A71cb8d0d-70d5-4752-abcd-e3bcf7f14783", show_col_types = FALSE)
-
-events <- read_csv("https://dev.nceas.ucsb.edu/knb/d1/mn/v2/object/urn%3Auuid%3A0a1dd2d8-e8db-4089-a176-1b557d6e2786", show_col_types = FALSE)
-```
-
-
-```{r}
-survey_clean <- survey_raw %>%
- select(-notes) %>%
- mutate(Q1 = if_else(Q1 == "1", "below expectations", Q1)) %>%
- mutate(Q2 = tolower(Q2))
-
-survey_joined <- left_join(survey_clean, events, by = "StartDate")
-```
-
-#### {-}
-
-We are going to be working in the "tidy text format." This format stipulates that the text column of our data frame contains rows with only one token per row. A token, in this case, is a meaningful unit of text. Depending on the analysis, that could be a word, two words, or phrase.
-
-First, let's create a data frame with responses to question 3, with the one token per row. We use the `unnest_tokens` function from `tidytext`, after selecting columns of interest.
-
-```{r}
-q3 <- survey_joined %>%
- select(StartDate, location, Q3) %>%
- unnest_tokens(output = word, input = Q3)
-```
-
-
-```{r, echo = FALSE}
-DT::datatable(q3, rownames = F)
-```
-
-You'll see that we now have a very long data frame with only one word in each row of the text column. Some of the words aren't so interesting though. The words that are likely not useful for analysis are called "stop words". There is a list of stop words contained within the `tidytext` package and we can access it using the `data` function. We can then use the `anti_join` function to return only the words that are not in the stop word list.
-
-```{r}
-data(stop_words)
-
-q3 <- anti_join(q3, stop_words)
-```
-
-```{r, echo = FALSE}
-DT::datatable(q3, rownames = F)
-```
-
-Now, we can do normal `dplyr` analysis to examine the most commonly used words in question 3. The `count` function is helpful here. We could also do a `group_by` and `summarize` and get the same result. We can also `arrange` the results, and get the top 10 using `slice_head`.
-
-```{r}
-q3_top <- q3 %>%
- count(word) %>%
- arrange(-n) %>%
- slice_head(n = 10)
-```
-
-
-```{r, echo = FALSE}
-DT::datatable(q3_top, rownames = F)
-```
-
-#### Term frequency {- .aside}
-
-Right now, our counts of the most commonly used non-stop words are only moderately informative because they don't take into context how many other words, responses, and courses there are. A widely used metric to analyze and draw conclusions from word frequency, including frequency within documents (or courses, in our case) is called tf-idf. This is the term frequency (number of appearances of a term divided by total number of terms), multiplied by the inverse document frequency (the natural log of the number of documents divided by the number of documents containing the term). The [tidytext book](https://www.tidytextmining.com/tfidf.html) has great examples on how to calculate this metric easily using some built in functions to the package.
-
-#### {-}
-
-Let's do the same workflow for question 4:
-
-```{r}
-q4 <- survey_joined %>%
- select(StartDate, location, Q4) %>%
- unnest_tokens(output = word, input = Q4) %>%
- anti_join(stop_words)
-
-q4_top <- q4 %>%
- count(word) %>%
- arrange(-n) %>%
- slice_head(n = 10)
-```
-
-```{r, echo = FALSE}
-DT::datatable(q4_top, rownames = F)
-```
-
-Perhaps not surprisingly, the word data is mentioned a lot! In this case, it might be useful to add it to our stop words list. You can create a data.frame in place with your word, and an indication of the lexicon (in this case, your own, which we can call custom). Then we use `rbind` to bind that data frame with our previous stop words data frame.
-
-```{r}
-custom_words <- data.frame(word = "data", lexicon = "custom")
-
-stop_words_full <- rbind(stop_words, custom_words)
-```
-
-Now we can run our question 4 analysis again, with the `anti_join` on our custom list.
-
-```{r}
-q4 <- survey_joined %>%
- select(StartDate, location, Q4) %>%
- unnest_tokens(output = word, input = Q4) %>%
- anti_join(stop_words_full)
-
-q4_top <- q4 %>%
- count(word) %>%
- arrange(-n) %>%
- slice_head(n = 10)
-```
-
-
-## Unstructured Text
-
-The above example showed how to analyze text that was contained within a tabular format (a csv file). There are many other text formats that you might want to analyze, however. This might include pdf documents, websites, word documents, etc. Here, we'll look at how to read in the text from a PDF document into an analysis pipeline like above.
-
-Before we begin, it is important to understand that not all PDF documents can be processed this way. PDF files can store information in many ways, including both images and text. Some PDF documents, particularly older ones, or scanned documents, are images of text and the bytes making up the document do not contain a 'readable' version of the text in the image, it is an image not unlike one you would take with a camera. Other PDF documents will contain the text as character strings, along with the information on how to render it on the page (such as position and font). The analysis that follows will only work on PDF files that fit the second description of the format. If the PDF document you are trying to analyze is more like the first, you would need to first use a technique called Optical Character Recognition (OCR) to interpret the text in the image and store it in a parsable way. Since this document can be parsed, we'll proceed without doing OCR.
-
-First we'll load another library, `pdftools`, which will read in our PDF, and the `stringr` library, which helps manipulate character strings.
-
-```{r, message=FALSE, warning=FALSE}
-library(pdftools)
-library(stringr)
-```
-
-Next, navigate to the dataset [Elizabeth Rink and Gitte Adler Reimer. 2022. Population Dynamics in Greenland: A Multi-component Mixed-methods Study of Pregnancy Dynamics in Greenland (2014-2022). Arctic Data Center. doi:10.18739/A21Z41V1R.](http://doi.org/doi:10.18739/A21Z41V1R). Right click the download button next to the top PDF data file called 'Translation_of_FG_8_Ilulissat_170410_0077.pdf'.
-
-First we create a variable with a path to a location where we want to save the file.
-
-```{r, echo = FALSE}
-path <- tempfile()
-```
-
-```{r, eval = FALSE}
-path <- "data/Translation_of_FG_8_Ilulissat_170410_0077.pdf"
-```
-
-Then use `download.file` to download it and save it to that path.
-
-```{r}
-download.file("https://arcticdata.io/metacat/d1/mn/v2/object/urn%3Auuid%3A34999083-2fa1-4222-ab27-53204327e8fc", path)
-```
-
-The `pdf_text` function extracts the text from the PDF file, returning a vector of character strings with a length equal to the number of pages in the file. So, our return value is loaded into R, but maybe not that useful yet because it is just a bunch of really long strings.
-
-```{r}
-txt <- pdf_text(path)
-
-class(txt)
-```
-
-Luckily, there is a function that will turn the pdf text data we just read in to a form that is compatible with the rest of the `tidytext` tools. The `tibble::enframe` function, converts the list into a `data.frame`. We then change one column name to describe what the column actually is (page number).
-
-```{r}
-txt_clean <- txt %>%
- enframe() %>%
- rename(page = name)
-```
-
-We can do the same analysis as above, unnesting the tokens and removing stop words to get the most frequent words:
-
-
-```{r}
-pdf_summary <- txt_clean %>%
- unnest_tokens(output = word, input = value) %>%
- anti_join(stop_words) %>%
- count(word) %>%
- arrange(-n) %>%
- slice_head(n = 10)
-```
-
-```{r, echo = FALSE}
-DT::datatable(pdf_summary, rownames = F)
-```
-
-
-If we look at the result, and then back at the original document, it is clear that there is more work needed to get the data to an analyzable state. The header and footer of each page of the PDF were included in the text we analyzed, and since they are repeated every page (and aren't really the subject of our inquiry anyway), should be removed from the text after we read it into R but before we try to calculate the most used words. It might also be beneficial to try and separate out the questions from responses, if we wanted to analyze just the responses or just the questions.
-
-To help us clean things up, first let's split our value column currently containing full pages of text by where there are double newlines (`\n\n`). You can see in the original PDF how this demarcates the responses, which contain single newlines within each paragraph, and two new lines (an empty line) between paragraphs. You can see an example of this within the text we have read in by examining just the first 500 characters of the first page of data.
-
-```{r}
-substr(txt_clean$value[1], 1,500)
-```
-
-To split our character vectors, we will use the `str_split` function. It splits a character vector according to a separator, and stores the values in a list. To show more clearly, let's look at a dummy example. We can split a string of comma separated numbers into a list with each individual number.
-
-```{r}
-x <- "1,2,3,4,5"
-str_split(x, ",")
-```
-
-
-In the real dataset, we'll use `str_split` and `mutate`, which will create a list of values within each row of the `value` column. So each cell in the `value` column contains a list of values like the result of the example above. We can "flatten" this data so that each cell only has one value by using the `unnest` function, which takes as arguments the columns to flatten. Let's take the example above, and make it a little more like our real data.
-
-First turn the original dummy vector into a data frame, and do our split as before, this time using `mutate`.
-
-```{r}
-x_df <- data.frame(x = x) %>%
- mutate(x = str_split(x, ","))
-x_df
-```
-Then you can run the `unnest` on the column of split values we just created.
-
-```{r}
-x_df_flat <- x_df %>%
- unnest(cols = x)
-
-x_df_flat
-```
-Now that we know how this works, let's do it on our dataset with the double newline character as the separator.
-
-```{r}
-txt_clean <- txt_clean %>%
- mutate(value = str_split(value, "\n\n")) %>%
- unnest(cols = value)
-```
-
-```{r}
-DT::datatable(txt_clean, rownames = F)
-```
-
-
-You can see that our questions and answers are now easily visible because they all start with wither Q or A. The other lines are blank lines or header/footer lines from the document. So, let's extract the first few characters of each line into a new column using `substr`, with the goal that we'll run a `filter` for rows that start with Q or A, thus discarding all the other rows.
-
-First, we extract the first 4 characters of each row and using `mutate` create a new column with those values called `id`.
-
-```{r}
-txt_clean <- txt_clean %>%
- mutate(id = substr(value, 1, 4))
-```
-
-Let's have a look at the unique values there:
-
-```{r}
-unique(txt_clean$id)
-```
-
-So unfortunately some of the text is a tiny bit garbled, there are newlines before at least some Q and A ids. We can use mutate again with `str_replace` to replace those `\n` with a blank value, which will remove them.
-
-```{r}
-txt_clean <- txt_clean %>%
- mutate(id = substr(value, 1, 4)) %>%
- mutate(id = str_replace(id, "\n", ""))
-```
-
-```{r}
-unique(txt_clean$id)
-```
-
-Now we will use `substr` again to get the first two characters of each id.
-
-```{r}
-txt_clean <- txt_clean %>%
- mutate(id = substr(value, 1, 4)) %>%
- mutate(id = str_replace(id, "\n", "")) %>%
- mutate(id = substr(id, 1, 2))
-```
-
-```{r}
-unique(txt_clean$id)
-```
-
-Finally, we can run the filter. Here, we filter for `id` values that start with either a Q or an A using the `grepl` function and a regular expression. We won't go much into regular expression details, but there is a chapter in the appendix for more about how they work.
-
-Here is an example of grepl in action. It returns a true or false for whether the value of x starts with (signified by `^`) a Q or A (signified by QA in square brackets).
-
-```{r}
-x <- c("Q3", "F1", "AAA", "FA")
-grepl("^[QA]", x)
-```
-
-So let's run that within a `filter` which will return only rows where the `grepl` would return TRUE.
-
-```{r}
-txt_clean <- txt_clean %>%
- mutate(id = substr(value, 1, 4)) %>%
- mutate(id = str_replace(id, "\n", "")) %>%
- mutate(id = substr(id, 1, 2)) %>%
- filter(grepl("^[QA]", id))
-```
-
-```{r, echo = FALSE}
-DT::datatable(txt_clean, rownames = F)
-```
-
-Finally, as our last cleaning step we replace all instances of the start of a string that contains a Q or A, followed by a digit and a colon, with an empty string (removing them from the beginning of the line.
-
-```{r}
-txt_clean <- txt_clean %>%
- mutate(id = substr(value, 1, 4)) %>%
- mutate(id = str_replace(id, "\n", "")) %>%
- mutate(id = substr(id, 1, 2)) %>%
- filter(grepl("^[QA]", id)) %>%
- mutate(value = str_replace_all(value, "[QA][0-9]\\:", ""))
-```
-
-```{r, echo = FALSE}
-DT::datatable(txt_clean, rownames = F)
-```
-
-Finally, we can try the same analysis again as above to look for the most commonly used words.
-
-```{r}
-pdf_summary <- txt_clean %>%
- unnest_tokens(output = word, input = value) %>%
- anti_join(stop_words) %>%
- count(word) %>%
- arrange(-n) %>%
- slice_head(n = 10)
-```
-
-```{r, echo = FALSE}
-DT::datatable(pdf_summary, rownames = F)
-```
-
-## Sentiment Analysis
-
-In sentiment analysis, tokens (in this case our single words) are evaluated against a dictionary of words where a sentiment is assigned to the word. There are many different sentiment lexicons, some with single words, some with more than one word, and some that are aimed at particular disciplines. When embarking on a sentiment analysis project, choosing your lexicon is one that should be done with care. Sentiment analysis can also be done using machine learning algorithms.
-
-With that in mind, we will next do a very simple sentiment analysis on our Q3 and Q4 answers using the [bing lexicon](https://www.cs.uic.edu/~liub/FBS/sentiment-analysis.html) from Bing Liu and collaborators, which ships with the `tidytext` package.
-
-First we will use the `get_sentiments` function to load the lexicon.
-
-```{r}
-bing <- get_sentiments("bing")
-```
-
-Next we do an inner join to return the words from question 3 that are contained within the lexicon.
-
-```{r}
-q3_sent <- inner_join(q3, bing, by = "word")
-```
-
-```{r, echo=F}
-DT::datatable(q3_sent, rownames = F)
-```
-
-There are a variety of directions you could go from here, analysis wise, such as calculating an overall sentiment index for that question, plotting sentiment against some other variable, or, making a fun word cloud like below! Here we bring in `reshape2::acast` to create a sentiment matrix for each word, and pass that into `wordcloud::comparison.cloud` to look at a wordcloud that indicates the frequency and sentiment of the words in our responses.
-
-```{r}
-q3_sent %>%
- count(word, sentiment, sort = TRUE) %>%
- acast(word ~ sentiment, value.var = "n", fill = 0) %>%
- comparison.cloud(colors = c("gray20", "gray80"),
- max.words = 100, title.size = 2)
-```
-
-Let's look at the question 4 word cloud:
-
-```{r}
-q4 %>%
- inner_join(bing, by = "word") %>%
- count(word, sentiment, sort = TRUE) %>%
- acast(word ~ sentiment, value.var = "n", fill = 0) %>%
- comparison.cloud(colors = c("gray20", "gray80"),
- max.words = 100, title.size = 2)
-```
-
-
-## Summary
-
-In this lesson, we learned:
-
-- how to analyze structured text data from a survey using stop words and sentiment analysis
-- how to give structure to unstructured text data from a PDF to do some analysis using stop words and times words are used
\ No newline at end of file
diff --git a/materials/sections/visualization-shiny.Rmd b/materials/sections/visualization-shiny.Rmd
deleted file mode 100644
index f073d636..00000000
--- a/materials/sections/visualization-shiny.Rmd
+++ /dev/null
@@ -1,610 +0,0 @@
-## Introduction to Shiny
-
-### Learning Objectives
-
-In this lesson we will:
-
-- review the capabilities in Shiny applications
-- learn about the basic layout for Shiny interfaces
-- learn about the server component for Shiny applications
-- build a simple shiny application for interactive plotting
-
-### Overview
-
-[Shiny](http://shiny.rstudio.com/) is an R package for creating interactive data visualizations embedded in a web application that you and your colleagues can view with just a web browser. Shiny apps are relatively easy to construct, and provide interactive features for letting others share and explore data and analyses.
-
-There are some really great examples of what Shiny can do on the RStudio webite like [this one exploring movie metadata](https://shiny.rstudio.com/gallery/movie-explorer.html). A more scientific example is a tool from the SASAP project exploring [proposal data from the Alaska Board of Fisheries](https://sasap-data.shinyapps.io/board_of_fisheries/). There is also an app for [Delta monitoring efforts](https://deltascience.shinyapps.io/monitoring/).
-
-
-
-
-
-Most any kind of analysis and visualization that you can do in R can be turned into a useful interactive visualization for the web that lets people explore your data more intuitively But, a Shiny application is not the best way to preserve or archive your data. Instead, for preservation use a repository that is archival in its mission like the [KNB Data Repository](https://knb.ecoinformatics.org), [Zenodo](https://zenodo.org), or [Dryad](https://datadryad.org). This will assign a citable identifier to the specific version of your data, which you can then read in an interactive visualiztion with Shiny.
-
-For example, the data for the Alaska Board of Fisheries application is published on the KNB and is citable as:
-
-Meagan Krupa, Molly Cunfer, and Jeanette Clark. 2017. Alaska Board of Fisheries Proposals 1959-2016. Knowledge Network for Biocomplexity. [doi:10.5063/F1QN652R](https://doi.org/10.5063/F1QN652R).
-
-While that is the best citation and archival location of the dataset, using Shiny, one can also provide an easy-to-use exploratory web application that you use to make your point that directly loads the data from the archival site. For example, the Board of Fisheries application above lets people who are not inherently familiar with the data to generate graphs showing the relationships between the variables in the dataset.
-
-We're going to create a simple shiny app with two sliders so we can interactively control inputs to an R function. These sliders will allow us to interactively control a plot.
-
-### Create a sample shiny application
-
-- File > New > Shiny Web App...
-- Set some fields:
-
- - Name it "myapp" or something else
- - Select "Single File"
- - Choose to create it in a new folder called 'shiny-demo'
- - Click Create
-
-RStudio will create a new file called `app.R` that contains the Shiny application.
-Run it by choosing `Run App` from the RStudio editor header bar. This will bring up
-the default demo Shiny application, which plots a histogram and lets you control
-the number of bins in the plot.
-
-
-
-Note that you can drag the slider to change the number of bins in the histogram.
-
-### Shiny architecture
-
-A Shiny application consists of two functions, the `ui` and the `server`. The `ui`
-function is responsible for drawing the web page, while the `server` is responsible
-for any calculations and for creating any dynamic components to be rendered.
-
-Each time that a user makes a change to one of the interactive widgets, the `ui`
-grabs the new value (say, the new slider min and max) and sends a request to the
-`server` to re-render the output, passing it the new `input` values that the user
-had set. These interactions can sometimes happen on one computer (e.g., if the
-application is running in your local RStudio instance). Other times, the `ui` runs on
-the web browser on one computer, while the `server` runs on a remote computer somewhere
-else on the Internet (e.g., if the application is deployed to a web server).
-
-
-
-
-### Interactive scatterplots
-
-Let's modify this application to plot Yolo bypass secchi disk data in a time-series, and allow aspects of the plot to be interactively changed.
-
-#### Load data for the example
-
-Use this code to load the data at the top of your `app.R` script. Note we are using `contentId` again, and we have filtered
-for some species of interest.
-
-```{r load_bgchem, eval = FALSE}
-library(shiny)
-library(contentid)
-library(dplyr)
-library(ggplot2)
-library(lubridate)
-
-sha1 <- 'hash://sha1/317d7f840e598f5f3be732ab0e04f00a8051c6d0'
-delta.file <- contentid::resolve(sha1, registries=c("dataone"), store = TRUE)
-
-# fix the sample date format, and filter for species of interest
-delta_data <- read.csv(delta.file) %>%
- mutate(SampleDate = mdy(SampleDate)) %>%
- filter(grepl("Salmon|Striped Bass|Smelt|Sturgeon", CommonName))
-
-names(delta_data)
-
-```
-
-#### Add a simple timeseries using ggplot
-
-We know there has been a lot of variation through time in the delta, so let's plot a time-series of Secchi depth. We do so by switching out the histogram code for a simple ggplot, like so:
-
-```{r server_chunk, eval=FALSE}
-server <- function(input, output) {
-
- output$distPlot <- renderPlot({
-
- ggplot(delta_data, mapping = aes(SampleDate, Secchi)) +
- geom_point(colour="red", size=4) +
- theme_light()
- })
-}
-```
-
-If you now reload the app, it will display the simple time-series instead of
-the histogram. At this point, we haven't added any interactivity.
-
-In a Shiny application, the `server` function provides the part of the application
-that creates our interactive components, and returns them to the user interface (`ui`)
-to be displayed on the page.
-
-#### Add sliders to set the start and end date for the X axis
-
-To make the plot interactive, first we need to modify our user interface to include
-widgits that we'll use to control the plot. Specifically, we will add a new slider
-for setting the `minDate` parameter, and modify the existing slider to be used for
-the `maxDate` parameter. To do so, modify the `sidebarPanel()` call to include two
-`sliderInput()` function calls:
-
-```{r ui_chunk, eval=FALSE}
-sidebarPanel(
- sliderInput("minDate",
- "Min Date:",
- min = as.Date("1998-01-01"),
- max = as.Date("2020-01-01"),
- value = as.Date("1998-01-01")),
- sliderInput("maxDate",
- "Max Date:",
- min = as.Date("1998-01-01"),
- max = as.Date("2020-01-01"),
- value = as.Date("2005-01-01"))
-)
-```
-
-If you reload the app, you'll see two new sliders, but if you change them, they don't
-make any changes to the plot. Let's fix that.
-
-#### Connect the slider values to the plot
-
-Finally, to make the plot interactive, we can use the `input` and `output` variables
-that are passed into the `server` function to access the current values of the sliders.
-In Shiny, each UI component is given an input identifier when it is created, which is used as the name of the value in the input list. So, we can access the minimum depth as `input$minDate` and the max as `input$maxDate`. Let's use these values now by adding limits to our X axis
-in the ggplot:
-
-```{r shiny_ggplot_interactive, eval=FALSE}
- ggplot(delta_data, mapping = aes(SampleDate, Secchi)) +
- geom_point(colour="red", size=4) +
- xlim(c(input$minDate,input$maxDate)) +
- theme_light()
-```
-
-At this point, we have a fully interactive plot, and the sliders can be used to change the
-min and max of the Depth axis.
-
-
-
-Looks so shiny!
-
-#### Reversed Axes?
-
-What happens if a clever user sets the minimum for the X axis at a greater value than the maximum?
-You'll see that the direction of the X axis becomes reversed, and the plotted points display right
-to left. This is really an error condition. Rather than use two independent sliders, we can modify
-the first slider to output a range of values, which will prevent the min from being greater than
-the max. You do so by setting the value of the slider to a vector of length 2, representing
-the default min and max date for the slider, such as `c(as.Date("1998-01-01"), as.Date("2020-01-01"))`. So, delete the second slider,
-rename the first, and provide a vector for the value, like this:
-
-```{r shiny_sidebar, eval=FALSE}
-sliderInput("date",
- "Date:",
- min = as.Date("1998-01-01"),
- max = as.Date("2020-01-01"),
- value = c(as.Date("1998-01-01"), as.Date("2020-01-01")))
-)
-```
-
-Now, modify the ggplot to use this new `date` slider value, which now will be returned
-as a vector of length 2. The first element of the depth vector is the min, and the
-second is the max value on the slider.
-
-```{r shiny_limvector, eval=FALSE}
- ggplot(delta_data, mapping = aes(SampleDate, Secchi)) +
- geom_point(colour="red", size=4) +
- xlim(c(input$date[1],input$date[2])) +
- theme_light()
-```
-
-
-
-
-### Extending the user interface with dynamic plots
-
-If you want to display more than one plot in your application, and provide
-a different set of controls for each plot, the current layout would be too simple.
-Next we will extend the application to break the page up into vertical sections, and
-add a new plot in which the user can choose which variables are plotted. The current
-layout is set up such that the `FluidPage` contains the title element, and then
-a `sidebarLayout`, which is divided horizontally into a `sidebarPanel` and a
-`mainPanel`.
-
-
-
-#### Vertical layout
-
-To extend the layout, we will first nest the existing `sidebarLayout` in a new
-`verticalLayout`, which simply flows components down the page vertically. Then
-we will add a new `sidebarLayout` to contain the bottom controls and graph.
-
-
-
-This mechanism of alternately nesting vertical and horizontal panels can be used
-to segment the screen into boxes with rules about how each of the panels is resized,
-and how the content flows when the browser window is resized. The `sidebarLayout`
-works to keep the sidebar about 1/3 of the box, and the main panel about 2/3, which
-is a good proportion for our controls and plots. Add the verticalLayout, and the
-second sidebarLayout for the second plot as follows:
-
-```{r shiny_vertical, eval=FALSE}
- verticalLayout(
- # Sidebar with a slider input for depth axis
- sidebarLayout(
- sidebarPanel(
- sliderInput("date",
- "Date:",
- min = as.Date("1998-01-01"),
- max = as.Date("2020-01-01"),
- value = c(as.Date("1998-01-01"), as.Date("2020-01-01")))
- ),
- # Show a plot of the generated distribution
- mainPanel(
- plotOutput("distPlot")
- )
- ),
-
- tags$hr(),
-
- sidebarLayout(
- sidebarPanel(
- selectInput("x_variable", "X Variable", cols, selected = "SampleDate"),
- selectInput("y_variable", "Y Variable", cols, selected = "Count"),
- selectInput("color_variable", "Color", cols, selected = "CommonName")
- ),
-
- # Show a plot with configurable axes
- mainPanel(
- plotOutput("varPlot")
- )
- ),
- tags$hr()
-```
-
-Note that the second `sidebarPanel` uses three `selectInput` elements to provide dropdown
-menus with the variable columns (`cols`) from our data frame. To manage that, we need to
-first set up the cols variable, which we do by saving the variables names
-from the `delta_data` data frame to a variable:
-
-```{r shiny_cols_2, eval=FALSE, echo=TRUE}
-sha1 <- 'hash://sha1/317d7f840e598f5f3be732ab0e04f00a8051c6d0'
-delta.file <- contentid::resolve(sha1, registries=c("dataone"), store = TRUE)
-
-# fix the sample date format, and filter for species of interest
-delta_data <- read.csv(delta.file) %>%
- mutate(SampleDate = mdy(SampleDate)) %>%
- filter(grepl("Salmon|Striped Bass|Smelt|Sturgeon", CommonName))
-
-cols <- names(delta_data)
-```
-
-
-#### Add the dynamic plot
-
-Because we named the second plot `varPlot` in our UI section, we now need to modify
-the server to produce this plot. Its very similar to the first plot, but this time
-we want to use the selected variables from the user controls to choose which
-variables are plotted. These variable names from the `$input` are character
-strings, and so would not be recognized as symbols in the `aes` mapping in ggplot. As recommended by the tidyverse authors, we use the [non-standard evaluation](https://dplyr.tidyverse.org/articles/programming.html) syntax of `.data[["colname"]]` to access the variables.
-
-```{r shiny_aes_string, eval=FALSE, echo=TRUE}
- output$varPlot <- renderPlot({
- ggplot(delta_data, aes(x = .data[[input$x_variable]],
- y = .data[[input$y_variable]],
- color = .data[[input$color_variable]])) +
- geom_point(size = 4)+
- theme_light()
- })
-```
-
-
-### Finishing touches: data citation
-
-Citing the data that we used for this application is the right thing to do, and easy.
-You can add arbitrary HTML to the layout using utility functions in the `tags` list.
-
-```{r shiny_citation, eval=FALSE}
- # Application title
- titlePanel("Yolo Bypass Fish and Water Quality Data"),
- p("Data for this application are from: "),
- tags$ul(
- tags$li("Interagency Ecological Program: Fish catch and water quality data from the Sacramento River floodplain and tidal slough, collected by the Yolo Bypass Fish Monitoring Program, 1998-2018.",
- tags$a("doi:10.6073/pasta/b0b15aef7f3b52d2c5adc10004c05a6f", href="http://doi.org/10.6073/pasta/b0b15aef7f3b52d2c5adc10004c05a6f")
- )
- ),
- tags$br(),
- tags$hr(),
-```
-
-
-The final application shows the data citation, the depth plot, and the
-configurable scatterplot in three distinct panels.
-
-
-
-### Publishing Shiny applications
-
-Once you've finished your app, you'll want to share it with others. To do so, you need to
-publish it to a server that is set up to [handle Shiny apps](https://shiny.rstudio.com/deploy/).
-Your main choices are:
-
-- [shinyapps.io](http://www.shinyapps.io/) (Hosted by RStudio)
- - This is a service offered by RStudio, which is initially free for 5 or fewer apps
- and for limited run time, but has paid tiers to support more demanding apps. You
- can deploy your app using a single button push from within RStudio.
-- [Shiny server](https://www.rstudio.com/products/shiny/shiny-server/) (On premises)
- - This is an open source server which you can deploy for free on your own hardware.
- It requires more setup and configuration, but it can be used without a fee.
-- [RStudio connect](https://www.rstudio.com/products/connect/) (On premises)
- - This is a paid product you install on your local hardware, and that contains the most
- advanced suite of services for hosting apps and RMarkdown reports. You can
- publish using a single button click from RStudio.
-
-A comparison of [publishing features](https://rstudio.com/products/shiny/shiny-server/) is available from RStudio.
-
-#### Publishing to shinyapps.io
-
-The easiest path is to create an account on shinyapps.io, and then configure RStudio to
-use that account for publishing. Instructions for enabling your local RStudio to publish
-to your account are displayed when you first log into shinyapps.io:
-
-
-
-Once your account is configured locally, you can simply use the `Publish` button from the
-application window in RStudio, and your app will be live before you know it!
-
-
-
-### Summary
-
-Shiny is a fantastic way to quickly and efficiently provide data exploration for your
-data and code. We highly recommend it for its interactivity, but an archival-quality
-repository is the best long-term home for your data and products. In this example,
-we used data drawn directly from the [EDI repository](http://doi.org/10.6073/pasta/b0b15aef7f3b52d2c5adc10004c05a6f)
-in our Shiny app, which offers both the preservation guarantees of an archive, plus
-the interactive data exploration from Shiny. You can utilize the full power of R and
-the tidyverse for writing your interactive applications.
-
-### Full source code for the final application
-
-```{r shinyapp_source, eval=FALSE}
-
-library(shiny)
-library(contentid)
-library(dplyr)
-library(ggplot2)
-library(lubridate)
-
-# read in the data from EDI
-sha1 <- 'hash://sha1/317d7f840e598f5f3be732ab0e04f00a8051c6d0'
-delta.file <- contentid::resolve(sha1, registries=c("dataone"), store = TRUE)
-
-# fix the sample date format, and filter for species of interest
-delta_data <- read.csv(delta.file) %>%
- mutate(SampleDate = mdy(SampleDate)) %>%
- filter(grepl("Salmon|Striped Bass|Smelt|Sturgeon", CommonName))
-
-cols <- names(delta_data)
-
-
-
-# Define UI for application that draws a two plots
-ui <- fluidPage(
-
- # Application title and data source
- titlePanel("Sacramento River floodplain fish and water quality dataa"),
- p("Data for this application are from: "),
- tags$ul(
- tags$li("Interagency Ecological Program: Fish catch and water quality data from the Sacramento River floodplain and tidal slough, collected by the Yolo Bypass Fish Monitoring Program, 1998-2018.",
- tags$a("doi:10.6073/pasta/b0b15aef7f3b52d2c5adc10004c05a6f", href="http://doi.org/10.6073/pasta/b0b15aef7f3b52d2c5adc10004c05a6f")
- )
- ),
- tags$br(),
- tags$hr(),
-
- verticalLayout(
- # Sidebar with a slider input for time axis
- sidebarLayout(
- sidebarPanel(
- sliderInput("date",
- "Date:",
- min = as.Date("1998-01-01"),
- max = as.Date("2020-01-01"),
- value = c(as.Date("1998-01-01"), as.Date("2020-01-01")))
- ),
- # Show a plot of the generated timeseries
- mainPanel(
- plotOutput("distPlot")
- )
- ),
-
- tags$hr(),
-
- sidebarLayout(
- sidebarPanel(
- selectInput("x_variable", "X Variable", cols, selected = "SampleDate"),
- selectInput("y_variable", "Y Variable", cols, selected = "Count"),
- selectInput("color_variable", "Color", cols, selected = "CommonName")
- ),
-
- # Show a plot with configurable axes
- mainPanel(
- plotOutput("varPlot")
- )
- ),
- tags$hr()
- )
-)
-
-# Define server logic required to draw the two plots
-server <- function(input, output) {
-
- # turbidity plot
- output$distPlot <- renderPlot({
-
- ggplot(delta_data, mapping = aes(SampleDate, Secchi)) +
- geom_point(colour="red", size=4) +
- xlim(c(input$date[1],input$date[2])) +
- theme_light()
- })
-
- # mix and match plot
- output$varPlot <- renderPlot({
- ggplot(delta_data, aes(x = .data[[input$x_variable]],
- y = .data[[input$y_variable]],
- color = .data[[input$color_variable]])) +
- geom_point(size = 4) +
- theme_light()
- })
-}
-
-
-# Run the application
-shinyApp(ui = ui, server = server)
-
-```
-
-
-### A shinier app with tabs and a map!
-
-```{r, eval = F}
-library(shiny)
-library(contentid)
-library(dplyr)
-library(tidyr)
-library(ggplot2)
-library(lubridate)
-library(shinythemes)
-library(sf)
-library(leaflet)
-library(snakecase)
-
-# read in the data from EDI
-sha1 <- 'hash://sha1/317d7f840e598f5f3be732ab0e04f00a8051c6d0'
-delta.file <- contentid::resolve(sha1, registries=c("dataone"), store = TRUE)
-
-# fix the sample date format, and filter for species of interest
-delta_data <- read.csv(delta.file) %>%
- mutate(SampleDate = mdy(SampleDate)) %>%
- filter(grepl("Salmon|Striped Bass|Smelt|Sturgeon", CommonName)) %>%
- rename(DissolvedOxygen = DO,
- Ph = pH,
- SpecificConductivity = SpCnd)
-
-cols <- names(delta_data)
-
-sites <- delta_data %>%
- distinct(StationCode, Latitude, Longitude) %>%
- drop_na() %>%
- st_as_sf(coords = c('Longitude','Latitude'), crs = 4269, remove = FALSE)
-
-
-
-# Define UI for application
-ui <- fluidPage(
- navbarPage(theme = shinytheme("flatly"), collapsible = TRUE,
- HTML('Sacramento River Floodplain Data'), id="nav",
- windowTitle = "Sacramento River floodplain fish and water quality data",
-
- tabPanel("Data Sources",
- verticalLayout(
- # Application title and data source
- titlePanel("Sacramento River floodplain fish and water quality data"),
- p("Data for this application are from: "),
- tags$ul(
- tags$li("Interagency Ecological Program: Fish catch and water quality data from the Sacramento River floodplain and tidal slough, collected by the Yolo Bypass Fish Monitoring Program, 1998-2018.",
- tags$a("doi:10.6073/pasta/b0b15aef7f3b52d2c5adc10004c05a6f", href="http://doi.org/10.6073/pasta/b0b15aef7f3b52d2c5adc10004c05a6f")
- )
- ),
- tags$br(),
- tags$hr(),
- p("Map of sampling locations"),
- mainPanel(leafletOutput("map"))
- )
- ),
-
- tabPanel(
- "Explore",
- verticalLayout(
- mainPanel(
- plotOutput("distPlot"),
- width = 12,
- absolutePanel(id = "controls",
- class = "panel panel-default",
- top = 175, left = 75, width = 300, fixed=TRUE,
- draggable = TRUE, height = "auto",
- sliderInput("date",
- "Date:",
- min = as.Date("1998-01-01"),
- max = as.Date("2020-01-01"),
- value = c(as.Date("1998-01-01"), as.Date("2020-01-01")))
-
- )
- ),
-
- tags$hr(),
-
- sidebarLayout(
- sidebarPanel(
- selectInput("x_variable", "X Variable", cols, selected = "SampleDate"),
- selectInput("y_variable", "Y Variable", cols, selected = "Count"),
- selectInput("color_variable", "Color", cols, selected = "CommonName")
- ),
-
- # Show a plot with configurable axes
- mainPanel(
- plotOutput("varPlot")
- )
- ),
- tags$hr()
- )
- )
- )
-)
-
-# Define server logic required to draw the two plots
-server <- function(input, output) {
-
-
- output$map <- renderLeaflet({leaflet(sites) %>%
- addTiles() %>%
- addCircleMarkers(data = sites,
- lat = ~Latitude,
- lng = ~Longitude,
- radius = 10, # arbitrary scaling
- fillColor = "gray",
- fillOpacity = 1,
- weight = 0.25,
- color = "black",
- label = ~StationCode)
- })
-
- # turbidity plot
- output$distPlot <- renderPlot({
-
- ggplot(delta_data, mapping = aes(SampleDate, Secchi)) +
- geom_point(colour="red", size=4) +
- xlim(c(input$date[1],input$date[2])) +
- labs(x = "Sample Date", y = "Secchi Depth (m)") +
- theme_light()
- })
-
- # mix and match plot
- output$varPlot <- renderPlot({
- ggplot(delta_data, mapping = aes(x = .data[[input$x_variable]],
- y = .data[[input$y_variable]],
- color = .data[[input$color_variable]])) +
- labs(x = to_any_case(input$x_variable, case = "title"),
- y = to_any_case(input$y_variable, case = "title"),
- color = to_any_case(input$color_variable, case = "title")) +
- geom_point(size=4) +
- theme_light()
- })
-}
-
-
-# Run the application
-shinyApp(ui = ui, server = server)
-```
-
-
-### Resources
-
-- [Main Shiny site](http://shiny.rstudio.com/)
-- [Official Shiny Tutorial](http://shiny.rstudio.com/tutorial/)