You signed in with another tab or window. Reload to refresh your session.You signed out in another tab or window. Reload to refresh your session.You switched accounts on another tab or window. Reload to refresh your session.Dismiss alert
{{ message }}
This repository has been archived by the owner on Aug 15, 2020. It is now read-only.
According to the prediction requirements, the time series is granular in minutes. We first remove all the parts with missing rate exceeding 10% in the time series, but in the remaining time series, there are still some sparse time series.
For example, the time series of consumers paying per minute has a value of 0 at many points in time. If these sequences are directly modeled, the prediction effect of the model may be greatly reduced. So, how do you preprocess these sparse time series before building a predictive model? Have you ever encountered a similar situation and how is it handled? Thank you
The following image is a screenshot of some of the data in the sparse time series (the length of time is one month)
The text was updated successfully, but these errors were encountered:
Sign up for freeto subscribe to this conversation on GitHub.
Already have an account?
Sign in.
According to the prediction requirements, the time series is granular in minutes. We first remove all the parts with missing rate exceeding 10% in the time series, but in the remaining time series, there are still some sparse time series.
For example, the time series of consumers paying per minute has a value of 0 at many points in time. If these sequences are directly modeled, the prediction effect of the model may be greatly reduced. So, how do you preprocess these sparse time series before building a predictive model? Have you ever encountered a similar situation and how is it handled? Thank you
The following image is a screenshot of some of the data in the sparse time series (the length of time is one month)
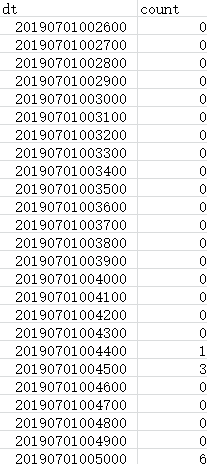
The text was updated successfully, but these errors were encountered: